Simon Haykin - Nonlinear Filters
Здесь есть возможность читать онлайн «Simon Haykin - Nonlinear Filters» — ознакомительный отрывок электронной книги совершенно бесплатно, а после прочтения отрывка купить полную версию. В некоторых случаях можно слушать аудио, скачать через торрент в формате fb2 и присутствует краткое содержание. Жанр: unrecognised, на английском языке. Описание произведения, (предисловие) а так же отзывы посетителей доступны на портале библиотеки ЛибКат.
- Название:Nonlinear Filters
- Автор:
- Жанр:
- Год:неизвестен
- ISBN:нет данных
- Рейтинг книги:5 / 5. Голосов: 1
-
Избранное:Добавить в избранное
- Отзывы:
-
Ваша оценка:
- 100
- 1
- 2
- 3
- 4
- 5
Nonlinear Filters: краткое содержание, описание и аннотация
Предлагаем к чтению аннотацию, описание, краткое содержание или предисловие (зависит от того, что написал сам автор книги «Nonlinear Filters»). Если вы не нашли необходимую информацию о книге — напишите в комментариях, мы постараемся отыскать её.
Discover the utility of using deep learning and (deep) reinforcement learning in deriving filtering algorithms with this insightful and powerful new resource Nonlinear Filters: Theory and Applications
Nonlinear Filters
Nonlinear Filters: Theory and Applications
Nonlinear Filters — читать онлайн ознакомительный отрывок
Ниже представлен текст книги, разбитый по страницам. Система сохранения места последней прочитанной страницы, позволяет с удобством читать онлайн бесплатно книгу «Nonlinear Filters», без необходимости каждый раз заново искать на чём Вы остановились. Поставьте закладку, и сможете в любой момент перейти на страницу, на которой закончили чтение.
Интервал:
Закладка:
2 (ii) Is it possible to perform inference from observables to the latent state variables?
At time instant , the inference problem to be solved is to find the estimate of
in the presence of noise, which is denoted by
. Depending of the value of
, estimation algorithms are categorized into three groups [3]:
1 (i) Prediction: ,
2 (ii) Filtering: ,
3 (iii) Smoothing: .
Regarding the mentioned two challenging questions, in order to improve performance, sophisticated representations can be deployed for the system under study. However, the corresponding inference algorithms may become computationally demanding. Hence, for designing efficient data‐driven inference algorithms, the following points must be taken into account [2]:
1 (i) The underlying assumptions for building a state‐space model must allow for reliable system identification and plausible long‐term prediction of the system behavior.
2 (ii) The inference mechanism must be able to capture rich dependencies.
3 (iii) The algorithm must be able to inherit the merit of learning machines to be trainable on raw data such as sensory inputs in a control system.
4 (iv) The algorithm must be scalable to big data regarding the optimization of model parameters based on the stochastic gradient descent method.
Regarding the important role of computation in inference problems, Section 1.3provides a brief account of the foundations of computing.
1.3 Construals of Computing
According to [4], a comprehensive theory of computing must meet three criteria:
1 (i) Empirical criterion: Doing justice to practice by keeping the analysis grounded in real‐world examples.
2 (ii) Conceptual criterion: Being understandable in terms of what it says, where it comes from, and what it costs.
3 (iii) Cognitive criterion: Providing an intelligible foundation for the computational theory of mind that underlies both artificial intelligence and cognitive science.
Following this line of thinking, it was proposed in [4] to distinguish the following construals of computation:
1 Formal symbol manipulation is rooted in formal logic and metamathematics. The idea is to build machines that are capable of manipulating symbolic or meaningful expressions regardless of their interpretation or semantic content.
2 Effective computability deals with the question of what can be done, and how hard it is to do it mechanically.
3 Execution of an algorithm or rule following focuses on what is involved in following a set of rules or instructions, and what behavior would be produced.
4 Calculation of a function considers the behavior of producing the value of a mathematical function as output, when a set of arguments is given as input.
5 Digital state machine is based on the idea of a finite‐state automaton.
6 Information processing focuses on what is involved in storing, manipulating, displaying, and trafficking of information.
7 Physical symbol systems is based on the idea that the way computers interact with symbols depends on their mutual physical embodiment. In this regard, computers may be assumed to be made of symbols.
8 Dynamics must be taken into account in terms of the roles that nonlinear elements, attractors, criticality, and emergence play in computing.
9 Interactive agents are capable of interacting and communicating with other agents and even people.
10 Self‐organizing or complex adaptive systems are capable of adjusting their organization or structure in response to changes in their environment in order to survive and improve their performance.
11 Physical implementation emphasizes on the occurrence of computational practice in real‐world systems.
1.4 Statistical Modeling
Statistical modeling aims at extracting information about the underlying data mechanism that allows for making predictions. Then, such predictions can be used to make decisions. There are two cultures in deploying statistical models for data analysis [5]:
Data modeling culture is based on the idea that a given stochastic model generates the data.
Algorithmic modeling culture uses algorithmic models to deal with an unknown data mechanism.
An algorithmic approach has the advantage of being able to handle large complex datasets. Moreover, it can avoid irrelevant theories or questionable conclusions.
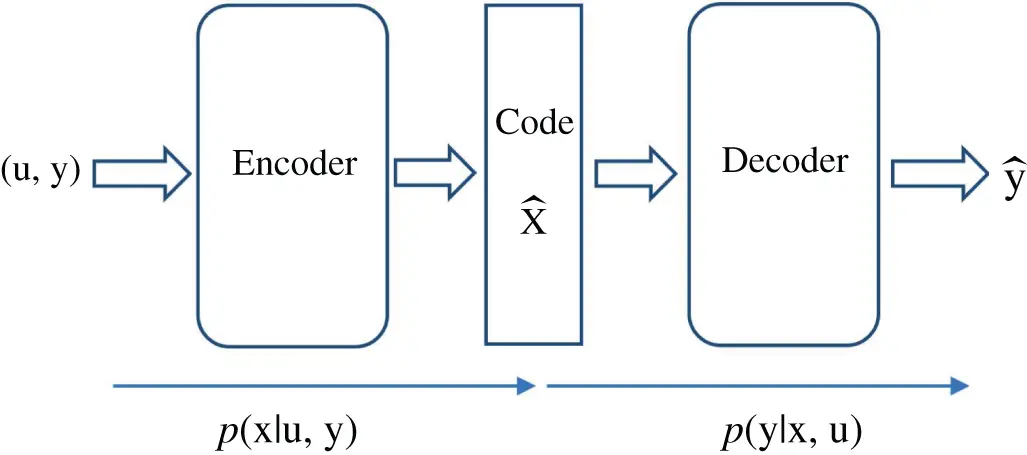
Figure 1.1 The encoder of an asymmetric autoencoder plays the role of a nonlinear filter.
Taking an algorithmic approach, in machine learning , statistical models can be classified as [6]:
1 (i) Generative models predict visible effects from hidden causes, .
2 (ii) Discriminative models infer hidden causes from visible effects, .
While the former is associated with the measurement process in a state‐space model, the latter is associated with the state estimation or filtering problem. Deploying machine learning, a wide range of filtering algorithms can be developed that are able to learn the corresponding state‐space models. For instance, an asymmetric autoencoder can be designed by combining a generative model and a discriminative model as shown in Figure 1.1[7]. Deep neural networks can be used to implement both the encoder and the decoder. Then, the resulting autoencoder can be trained in an unsupervised manner. After training, the encoder can be used as a filter, which estimates the latent state variables.
1.5 Vision for the Book
This book provides an algorithmic perspective on the nonlinear state/parameter estimation problem for discrete‐time systems, where measurements are available at discrete sampling times and estimators are implemented using digital processors. In Chapter 2, guidelines are provided for discretizing continuous‐time linear and nonlinear state‐space models. The rest of the book is organized as follows:
Chapter 2presents the notion of observability for deterministic and stochastic systems.
Chapters 3– 7cover classic estimation algorithms:
Chapter 3is dedicated to observers as state estimators for deterministic systems.
Chapter 4presents the general formulation of the optimal Bayesian filtering for stochastic systems.
Chapter 5covers the Kalman filter as the optimal Bayesian filter in the sense of minimizing the mean‐square estimation error for linear systems with Gaussian noise. Moreover, Kalman filter variants are presented that extend its applicability to nonlinear or non‐Gaussian cases.
Chapter 6covers the particle filter, which handles severe nonlinearity and non‐Gaussianity by approximating the corresponding distributions using a set of particles (random samples).
Chapter 7covers the smooth variable‐structure filter, which provides robustness against bounded uncertainties and noise. In addition to the innovation vector, this filter benefits from a secondary set of performance indicators.
Chapters 8– 11cover learning‐based estimation algorithms:
Chapter 8covers the basics of deep learning.
Chapter 9covers deep‐learning‐based filtering algorithms using supervised and unsupervised learning.
Читать дальшеИнтервал:
Закладка:
Похожие книги на «Nonlinear Filters»
Представляем Вашему вниманию похожие книги на «Nonlinear Filters» списком для выбора. Мы отобрали схожую по названию и смыслу литературу в надежде предоставить читателям больше вариантов отыскать новые, интересные, ещё непрочитанные произведения.
Обсуждение, отзывы о книге «Nonlinear Filters» и просто собственные мнения читателей. Оставьте ваши комментарии, напишите, что Вы думаете о произведении, его смысле или главных героях. Укажите что конкретно понравилось, а что нет, и почему Вы так считаете.