However, a drawback of RNN is that it has problem “remembering” remote information. In RNN, long‐term memory is reflected in the weights of the network, which memorizes remote information via shared weights. Short‐term memory is in the form of information flow, where the output from the previous state is passed into the current state. However, when the sequence length
is large, the optimization of RNN suffers from vanishing gradient problem. For example, if the loss
is evaluated at
, the gradient w.r.t.
calculated via backpropagation can be written as
(10) 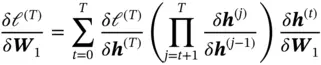
where
is the reason for the vanishing gradient. In RNN, the tanh function is commonly used as the activation function, so
(11) 
Therefore,
, and
is always smaller than 1. When
becomes larger, the gradient will get closer to zero, making it hard to train the network and update the weights with remote information. However, it is possible that relevant information is far apart in the sequence, so how to leverage remote information of a long sequence is important.
6.3 Long Short‐Term Memory Networks
To solve the problem of losing remote information, researchers proposed long short‐term memory (LSTM) networks. The idea of LSTM was introduced in Hochreiter and Schmidhuber [19], but it was applied to recurrent networks much later. The basic structure of LSTM is shown in Figure 9. It solves the problem of the vanishing gradient by introducing another hidden state
, which is called the cell state.
Since the original LSTM model was introduced, many variants have been proposed. Forget gate was introduced in Gers et al . [20]. It has been proven effective and is standard in most LSTM architectures. The forwarding process of LSTM with a forget gate can be divided into two steps. In the first step, the following values are calculated:
(12) 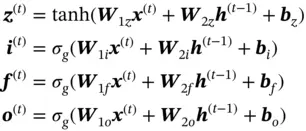
where
and
are weight matrix and bias, and
is the sigmoid function.
The two hidden states
and
are calculated by
(13) 
(14) 
where
represents elementwise product between matrices. In Equation ( 13), the first term multiplies
with
, controlling what information in the previous cell state can be passed to the current cell state. As for the second term,
stores the information passed from
and
, and
controls how much information from the current state is preserved in the cell state. The hidden state
depends on the current cell state and
, which decides how much information from the current cell state will be passed to the hidden state
.
Figure 9 Architecture of long short‐term memory network (LSTM).
In LSTM, if the loss
is evaluated at
, the gradient w.r.t.
calculated via backpropagation can be written as
(15) 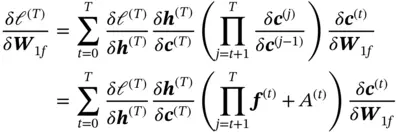
Читать дальше