The effect size, or size of effect, is simply the observed magnitude of a difference between two measurements or the strength of the association between two variables. For example, if there is a very obvious difference between the outcomes produced by two clinical treatments with a high proportion of patients cured, we would say the effect size is large. On the other hand, if the difference between the treatment outcomes were barely noticeable then we would say that the effect size is small. In general, the larger the effect size the larger will be the practical or clinical importance of the result. The effect size in clinical treatments is clearly important, but the effect size also impacts on the assessment of theories – where the observed effect size strongly influences the credibility of the theory.
A common question is how do we know what effect size is of practical importance? That depends. If we think of prices, a difference of $ 1 between two car insurance quotes would probably not be considered important. However, a $ difference in the cost of a coffee offered by two similar cafés would likely influence our choice of café. Sometimes, it is more difficult than this. For example, a drug that produces an absolute risk reduction of 1% might appear to be a small effect size. The reduction means that of 100 people taking the drug there would be 1 fewer people suffering from the disease. If the baseline rate of the disease is 10% in people not taking the drug, then taking the drug would reduce this to 9%. Again this might appear small, but if we consider a million people, this would represent an extra 10 000 people being affected if they did not take the drug.
Reporting the effect size for the results of a study is important, informing readers about the impact of the findings. Effect size is also used in the context of planning a study, since it influences the statistical power of the study. Here, it may be specified in three ways. First, the effect size may be that expected from similar previous published work or even from a pilot study. Second, it may be the minimum effect size that is of practical or clinical importance. Third, it may be simply an effect size that is considered to represent a useful effect. For example, in testing a new treatment in hypertensive patients with systolic blood pressure of 140 mmHg or more, a clinician might judge that a mean reduction of at least 10 mmHg would be clinically important and have a clear desirable health outcome. This would represent the minimum effect size. Alternatively, a clinician might not specify a minimum but merely judge that a mean decrease of 15 mmHg would be clinically important. (In practice, other aspects of a new treatment need to be considered – will the treatment be financially affordable and what might be the likely adverse side effects?)
In most areas of research, there is some effect, however small, for a treatment difference or a correlation. Tukey in 1991 [32] explained this in a forthright manner ‘Statisticians classically asked the wrong question – and were willing to answer with a lie, one that was often a downright lie. They asked “Are the effects of A and B different?” and they were willing to answer “no.” All we know about the world teaches us that the effects of A and B are always different – in some decimal place – for any A and B. Thus asking “Are the effects different?” is foolish’. Hence, we generally need to think about how large an effect is, rather than whether one is present or not. The latter practice is encouraged by the statistical testing approach where p is lesser than or greater than some significance level.
The habit of thinking about effect size forces the researcher to focus on the phenomenon under study. It places emphasis on practical/clinical importance of findings. One scientist, clearly interested in effect sizes, expresses their frustration ‘Honestly, at some point I'd like to work on things where the effect size is grounded on a real-world measurable outcome, but if I'm just looking at difference between psych measures, I'm not sure how to define it other than that’ (Twitter @PaoloAPalma 22 May 2019). This brings us on to how to define effect size.
Which metric should be used to define effect size? Baguley argues convincingly that the best measure of effect size uses the original units rather than standardized measures, and that the use of verbal labels such as ‘large’ or ‘small’ can sometimes be misleading [33]. What may be considered a large effect in one area (e.g. epidemiology) may be considered small in another (e.g. a drug treatment for hypertension). A popular standardized measure of effect size for a difference in means is d . This is actually Hedges' standardized statistic using the sample standard deviation SD rather than Cohen's using the population parameter σ . 4
(1.1) 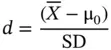
The relative effect sizes using d can be described as:
d |
Description |
0.2 |
Small |
0.5 |
Medium |
0.8 |
Large |
1.3 |
Very large |
A more general measure is provided by the correlation coefficient r . However, the transform between r and d is not linear since r is restricted to −1 and 1, while d varies between negative and positive infinity. For example a medium effect r of 0.3 corresponds to a d of 0.63 (on the large side), and a large r of .5 corresponds to a very large effect in d of 1.15. Using d allows us to relate more naturally to the measurements that are made.
Effect size is generally unaffected by sample size, unlike the p value. If the null is not true then the p value obtained will vary according to the sample size: other things being equal, the larger the sample, the smaller the p value. When considering sample size and strength of evidence provided by p values, opposite conclusions are reached by different statisticians [4], p. 71. In Figure 1.2, the 95% confidence intervals around means are plotted for two sets of data. For each interval, the same standard deviation is used and the same p value is obtained for the mean's difference from 0. However, the sample sizes vary, so that with N = 4, there is a 2.6 difference from 0, and for N = 80, there is a 0.6 difference from 0. Hence, the size of the effect is much larger for the interval using few observations, which might indicate that this result is of more practical importance than the result obtained with a larger data set. However, it is also argued that the data with larger N represents stronger evidence, although its effect size is much smaller and the p values identical.
Figure 1.2 Effect size versus sample size: which provides most evidence against H 0?
Evidence is measured by the natural logarithm of the LR, known as the support S . The words evidence and support will be used interchangeably.
Giving decimal places during calculations is tricky. The decimal places given for values in the text are usually given to an accuracy that allows one to check formulae and equations, often given in stages. Occasionally, there will be mismatches with the final answer which will be based on the most accurate calculation possible. These can usually be checked from the raw data using Excel or R.
Читать дальше