1 ...6 7 8 10 11 12 ...16 Figure 1.7gives a brief look at how analytics and artificial intelligence technologies are converged. In the competitive world, operational changes and planning should be done at a quick rate to survive in the market. A decision should be taken fast and it can happen when the tools used for the prediction can give us a result in no time otherwise it may become a disaster for the company if it takes decisions a late as the competitor can overtake the market within no time. Many big and reliable companies have lost the market for taking late decisions it has happened in the past and can happen in the future also. For instance, consider a client relationship application in which the customer calls the executive for some reason, and in this interaction, with the customer, the executive must clear the doubts of the client and satisfy him by deciding in a short time. This helps the organization to retain the customer and helps to add more clients to them when the service provided to them is done in no time. The problem is that there is a large amount of data available and to process it also is difficult. As the data contains structured, semi-structured, and unstructured a large number of analytical models are need to be incorporated so that the prediction can be improved.
Table 1.1 Different types of analytics and their examples [11].
S. no. |
Analytics type |
Description |
Examples |
1 |
Descriptive Analytics |
Realize what transpires when using analytic procedures on past and present data. |
Which item styles are selling better this quarter as analyzed to last quarter? Which districts are displaying the most elevated/least development?What components are affecting development in various areas? |
2 |
Predictive Analytics |
Comprehend what may happen when utilizing statistical predictive modeling capabilities, that includes both data mining and AI. Predictive models use past and current data to forecast forthcoming outcomes. Models search for patterns, clusters of behavior, and events. Models recognize outliers. |
What are the forecasts for next quarter’s sales by items and territory?How does this affect unprocessed acquisitions, human resources and inventory Management? |
3 |
Prescriptive Analytics |
Use to create a framework for deciding what to do or not do in the future. The “prescient” component ought to be tended to in prescriptive examination to help recognize the overall outcomes of your activities. Utilize an iterative procedure so that your model can gain from the relationship among activities and results |
What is the best blend of items for every locale?In What Way the consumers in each zone respond to marketing promotions and deals? What type of the offer ought to be made to each client to fabricate dependability and increment deals? |
4 |
Machine Learning and Cognitive Computing |
A coordinated effort among people and machines to take care of complicated issues.Incorporate and evaluate different sources of data to anticipate results.Need relies upon the issues you are attempting to understand.Improve adequacy of critical thinking and decrease blunders in predicting outcomes. |
In What Manner the city environment is secure?Are there any cautions from the immense the measure of data spilling from checking gadgets (video, sound, and detecting gadgets for smoke or harmful gases)?Which blend of drugs will furnish the best result for a particular cancer patient based on precise attributes of the tumor and genetic sequencing? |
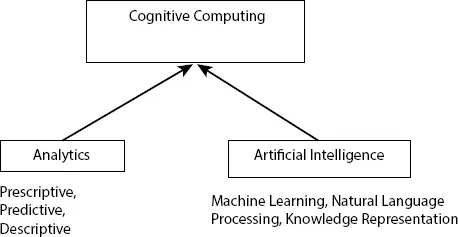
Figure 1.7 Figure showing the convergence of technologies.
Machine learning is the logical control that rose out of the general field of Artificial Intelligence. It is an interdisciplinary field where insights and information speculations are applied to discover the connections among the information and to build up programs by adapting consequently without human intercession. This procedure looks like the human learning process. Analysts are as yet attempting to make machines smart and act like people. This learning procedure begins with accessible information. Information assumes an essential job in the machine learning process. ML is also being utilized for information examination, such as identifying regularities in the information by fittingly managing incomplete information and the transformation of constant information.
Machine learning is multidisciplinary and is a subset of AI. However, it additionally consolidates the methods from statistics, control hypothesis, Cognitive Science as shown in Figure 1.8. The subsequent explanation is the exponential development of both accessible information and computer processing power. The order of AI additionally joins other information investigation disciplines like data mining, probability and statistics, computational complexity theory, Neurobiology, philosophy, and Information theory.
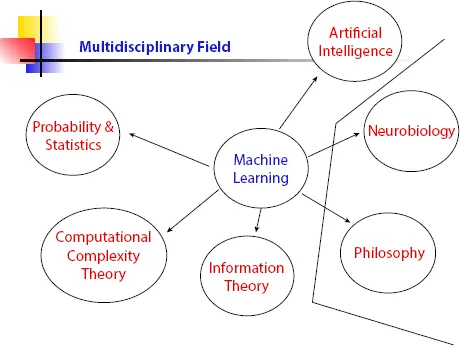
Figure 1.8 Machine learning.
Cognitive computing models use machine learning techniques dependent on inferential insights to identify or find designs that direct their behavioral patterns. Picking the fundamental learning way to deal with model recognition versus disclosure of examples ought to be founded on the available information and nature of the issues to be unraveled. AI regularly utilizes inferential insights (the reason for prescient, instead of precise examination) methods.
One of the more important uses of AI is to mechanize the procurement of information bases utilized by supposed master frameworks, which plans to imitate the dynamic procedure of human aptitude in a field. Be that as it may, the extent of its application has been developing.
The significant methodologies incorporate utilizing neural systems, case-based learning, hereditary calculations, rule enlistment, and analytical learning. While in the past they were applied autonomously, as of late these ideal models or models are being utilized in a crossbreed design, shutting the limits among them and empowering the improvement of increasingly compelling models. The blend of analytical techniques can guarantee compelling and repeatable and reliable outcomes, a necessary part for practical use in standard business and industry arrangements.
1.9 Machine Learning Process
1.9.1 Data Collection
The quantity and quality of data decide how our model performs. The gathered data is represented in a format which is further used in training
We can also get preprocessed data from Kaggle, UCI, or from any other public datasets.
Data preparation of machine learning process includes
Arranging information and set it up for preparing.
The cleaning process includes removing duplicate copies, handling mistakes, managing missing qualities, standardization, information type changes, and so on.
Randomizing information, which eradicates the impacts of the specific samples wherein we gathered or potentially, in any case, arranged our information.
Transforming information to identify pertinent connections between factors or class labels and characteristics (predisposition alert!), or perform other exploratory examination.
Читать дальше