1 ...7 8 9 11 12 13 ...16 Splitting data set into training and test data sets for learning and validating process.
Choosing the model is crucial in the machine learning process as the different algorithms are suitable for different tasks. Choosing an appropriate algorithm is a very important task.
The goal of training is to learn from data and use it to predict unseen data. For example in Linear, the regression algorithm would need to learn values for m (or W) and b (x is input, y is output)
In each iteration of the process, the model trains and improves its efficiency.
Model evaluation is done by a metric or combination of metrics and measures the performance of the model. The performance of the model is tested against previously unknown data. This unknown data may be from the real world and used to measure the performance and helps in tuning the model. Generally, the train and the split ratio is 80/20 or 70/30 depending on the data availability.
This progression alludes to hyperparameter tuning, which is a “fine art” instead of a science. Tune the model boundaries for improved execution. Straightforward model hyperparameters may include the number of preparing steps, learning rate, no of epochs, and so forth.
Utilizing further (test set) information which has, until this point, been retained from the model (and for which class names are known), are utilized to test the model; a superior estimate of how the model will act in reality.
1.10 Machine Learning Techniques
Machine learning comes in many different zests, depending on the algorithm and its objectives. The learning techniques are broadly classified into 3 types, Supervised learning, unsupervised, and reinforcement learning. Machine learning can be applied by specific learning strategies, such as:
1.10.1 Supervised Learning
It is a machine learning task of inferring function from labeled data. The model relies on pre-labeled data that contains the correct label for each input as shown in Figure 1.9. A supervised algorithm analyses the training example and produce an inferred function that can be used for mapping new examples. It is like learning with a teacher. The training data set is considered as a teacher. The teacher gives good examples for the student to memorize, and guide the student to derive general rules from these specific examples.
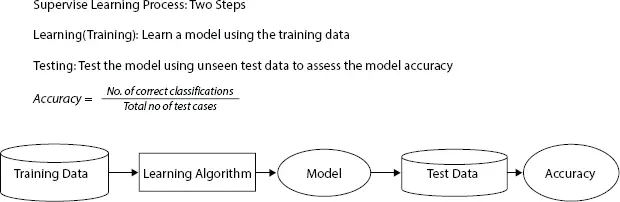
Figure 1.9 Supervised model.
In the Supervised learning technique, an algorithm learns from historical data and the related target labels which may consist of numeric values or string, as classes. And the trained model predicts the correct label when given with new examples.
The supervised approach is generally similar to human learning under the supervision of a teacher. There is a need to distinguish between regression problems, whose target is a numeric value, and classification problems, whose target is a qualitative variable, such as a class or a tag. A regression task determines the average prices of houses in the Boston area, and a classification task distinguishes between kinds of iris flowers based on their sepal and petal measures. A supervised strategy maps the data inputs and models them against desired outputs.
The supervised learning technique can be further divided into regression and classification problems.
Classification: In the classification problem, the output variable is a category, such as “red” or “blue” or “disease” and “no disease”. Classification emails into ‘spam’ or ‘not spam’ is another example.
Regression: In the regression problem, the output variable is a real value, such as “price” or “weight” or “sales”.
Some famous examples of supervised machine learning algorithms are:
SVM, Bayes, KNN, Random forest, Neural networks, Linear regression, Decision tree, etc.
1.10.2 Unsupervised Learning
An unsupervised strategy used to map the inputs and model them to find new trends. Derivative ones that combine these for a semi-supervised approach and others are also be used. Unsupervised learning is another form of machine learning algorithm which was applied to extract inferences from the large number of datasets consisting of input data without labeled responses.
Unsupervised learning happens when a calculation gains from plain models with no related reaction, leaving for the calculation to decide the information designs all alone. This sort of calculation will, in general, rebuild the information into something different, such as new highlights that may speak to a class or another arrangement of uncorrelated qualities. They are accommodating in giving people bits of knowledge into the significance of information and new valuable contributions to administered machine learning techniques.
The most widely recognized unsupervised learning technique is cluster analysis, which is utilized for exploratory information investigation to discover hidden examples or gathering in the information. It is like learning without a teacher. The machine learns through observation and finds structures in data.
Clustering and Association rule the two techniques that come under unsupervised learning.
Hierarchical clustering, K mean clustering, Markov models.
As a part of learning, it takes after the strategies people use to make sense of those specific articles or occasions are from a similar class, for example, by watching the level of similitude between objects. Some suggestion frameworks that find on the web through promoting robotization depend on this sort of learning.
This opens the entryway onto a huge number of utilizations for which AI can be utilized, in numerous territories, to depict, endorse, and find what is happening inside enormous volumes of assorted information.
1.10.3 Reinforcement Learning
Reinforcement Learning involves the mechanism of reward and punishment for the process of learning. In this type of learning, the objective is to maximize the reward and minimize the punishment. In Reinforcement Learning Errors help you learn because they have a penalty added (cost, loss of time, regret, pain, and so on).
Ex. when computers learn to play video games by themselves.
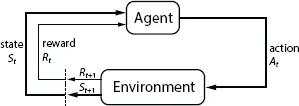
Figure 1.10 Reinforcement learning.
Reinforcement learning is connected to the applications for which the algorithm must make decisions and where the decisions held consequences. In the human world, it is similar to learning by trial and error. In cognitive computing, reinforcement learning is mostly used where numerous variables in the model are difficult to represent and the model has to do a sequence of tasks. For example Self-driving cars.
In reinforcement learning, we have an agent that acts in the environment as shown in Figure 1.10. The agent can take action and this action can impact the environment. In a particular stage, the agent takes an action and the environment goes to a new state and gives some reward to the agent, that reward may be positive can be a negative reward or penalty or can be nothing at that particular time step. But the agent is continually acting in this world.
Читать дальше