The model finds a relation between the reward and the sequence of tasks, which lead to getting a reward.
1.10.4 The Significant Challenges in Machine Learning
Identifying good hypothesis space
Optimization of accuracy on unknown data
Insufficient Training Data.
It takes a great deal of information for most Machine Learning calculations to work appropriately. For underlying issues, regularly need a vast number of models, and for complex issues, for example, picture or discourse recognition you may require a great many models.
Representation of Training Data
It is critical, to sum up, the preparation of information on the new cases. By utilizing a non-representative preparing set, we prepared a model that is probably not going to make precise forecasts, particularly for poor and rich nations. It is essential to utilize a preparation set that is illustrative of the cases you need to generalize to. This is frequently harder than it sounds: if the example is excessively small, you will have inspecting clamor. However, even extremely enormous examples can be non-representative of the testing technique is defective. This is called sample data bias.
Quality of Data
If the preparation of information is loaded with mistakes, exceptions, and clamor it will make it harder for the framework to distinguish the basic examples, so your framework is less inclined to perform well. It is regularly definitely justified even despite the push to invest energy tidying up your preparation information. In all actuality, most information researchers spend a noteworthy piece of their time doing only that. For instance: If a few occurrences are exceptions, it might help to just dispose of them or attempt to fix the blunders physically. If a few examples are feeling the loss of a couple of highlights (e.g., 5% of your clients did not determine their age), you should choose whether you need to overlook this characteristic altogether, disregard these occasions, fill in the missing qualities (e.g., with the middle age), or train one model with the component and one model without it, etc.
Unimportant Features
The machine learning framework might be fit for learning if the preparation information contains enough significant features and not very many unimportant ones. Now days Feature engineering, became very necessary for developing any type of model. Feature engineering process includes choosing the most helpful features to prepare on among existing highlights, consolidating existing highlights to deliver an increasingly valuable one (as we saw prior, dimensionality decrease calculations can help) and then creating new features by social event new information.
Overfitting
Overfitting implies that the model performs well on the preparation information, yet it doesn’t sum up well. Overfitting happens when the model is excessively mind boggling comparative with the sum and din of the preparation information.
The potential arrangements to overcome the overfitting problem are
To improve the model by choosing one with fewer boundaries (e.g., a straight model instead of a severe extent polynomial model), by lessening the number of characteristics in the preparation of data.
To assemble all the more preparing information
To lessen the commotion in the preparation information (e.g., fix information blunders and evacuate anomalies)
Constraining a model to make it more straightforward and decrease the danger of overfitting is called regularization.
A hypothesis is an idea or a guess which needs to be evaluated. The hypothesis may have two values i.e. true or false. For example, “All hibiscus have the same number of petals”, is a general hypothesis. In this example, a hypothesis is a testable declaration dependent on proof that clarifies a few watched marvel or connection between components inside a universe or specific space. At the point when a researcher details speculation as a response to an inquiry, it is finished in a manner that permits it to be tested. The theory needs to anticipate a predicted result. The ability to explain the hypothesis phenomenon is increased by experimenting the hypothesis testing. The hypothesis may be compared with the logic theory. For example, “If x is true then y” is a logical statement, here x became our hypothesis and y became the target output.
Hypothesis space is the set of all the possible hypotheses. The machine learning algorithm finds the best or optimal possible hypothesis which maps the target function for the given inputs. The three main variables to be considered while choosing a hypothesis space are the total size of hypothesis space and randomness either stochastic or deterministic. The hypothesis is rejected or supported only after analyzing the data and find the evidence for the hypothesis. Based on data the confidence level of the hypothesis is determined.
In terms of machine learning, the hypothesis may be a model that approximates the target function and which performs mappings of inputs to outputs. But in cognitive computing, it is termed as logical inference. The available data for supporting the hypothesis may not always structured. In real-world applications, the data is mostly unstructured. Figure 1.11shows an upright pattern of hypothesis generation and scoring. Understanding and traversing through the unstructured information requires a new computing technology which is called cognitive computing. The intellectual frameworks can create different hypotheses dependent on the condition of information in the corpus at a given time. When all the hypotheses are generated then they can be assessed and scored. In the below fig of, IBM’s Watson derives the responses questions and score each response. Here 100 autonomous hypothesis might be produced for a question after parsing the question and extracting the features of the question. Each generated hypothesis might be scored using the pieces of evidence.
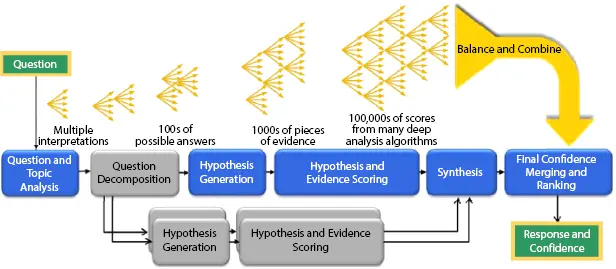
Figure 1.11 Hypotheses generation IBM Watson.
1.11.1 Hypothesis Generation
The hypothesis must be generalized and should map for the unseen cases also. The experiments are developed to test the general unseen case. There are two key ways a hypothesis might be produced in cognitive computing systems. The first is because of an express inquiry from the user, for example, “What may cause my fever and diarrhea?” The system generates all the possible explanations, like flu, COVID where we can see these symptoms. Sometimes the given data is not sufficient and might require some additional input and based on that the system refines the explanations. It might perceive that there are such a large number of answers to be valuable and solicitation more data from the client to refine the arrangement of likely causes.
This way to deal with hypothesis generation is applied where the objective of the model is to recognize the relations between the causes and its effects ex. Medical conditions and diseases. Normally, this kind of psychological framework will be prepared with a broad arrangement of inquiry/ answer sets. The model is trained using the available question and answer sets and generates candidate hypotheses.
The second sort of hypothesis generations doesn’t rely upon a client inquiring. Rather, the system continually searches for atypical information patterns that may demonstrate threats or openings. In this method, hypotheses are generated by identifying a new pattern. For example to detect unauthorized bank transactions the system generated those fraudulent transaction patterns, which became the hypothesis space. Then the cognitive computing model has to find the evidence to support or reject the hypothesis. The hypothesis space is mostly based on assumptions.
Читать дальше