In the example about creating a factor-object for feedback one will have noticed that the plotfunction does show the labels in alphabetical order and not in an order that for us – humans – would be logical. It is possible to coerce a certain order in the labels by providing the levels – in the correct order – while creating the factor-object.
feedback <- c(‘Good’,‘Good’,‘Bad’,‘Average’,‘Bad’,‘Good’) factor_feedback <- factor(feedback, levels= c(“Bad”,“Average”,“Good”)) plot(factor_feedback)
In Figure 4.2on page 63 we notice that the order is now as desired (it is the order that we have provided via the attribute labels
in the function factor()
.
Generate Factors with the Function gl()
Function use for gl()
gl(n, k, length = n*k, labels = seq_len(n), ordered = FALSE) with
n: The number of levels
k: The number of replications (for each level)
length (optional): An integer giving the length of the result
labels (optional): A vector with the labels
ordered: A boolean variable indicating whether the results should be ordered.
gl()
gl(3,2,, c(“bad”,“average”,“good”),TRUE) ## [1] bad bad average average good good ## Levels: bad < average < good 
Figure 4.2 : The factor objects appear now in a logical order.
Question #4
Use the dataset mtcars (from the library MASS) and explore the distribution of number of gears. Then explore the correlation between gears and transmission.
Question #5
Then focus on the transmission and create a factor-object with the words “automatic” and “manual” instead of the numbers 0 and 1.
Use the ?mtcars
to find out the exact definition of the data.
mtcars
Question #6
Use the dataset mtcars (fromthe libraryMASS) and explore the distribution of the horsepower (hp). How would you proceed to make a factoring (e.g. Low, Medium, High) for this attribute? Hint: Use the function cut()
.
cut()
4.3.8 Data Frames
4.3.8.1 Introduction to Data Frames
Data frames are the prototype of all two-dimensional data (also known as “rectangular data”). For statistical analysis this is obviously an important data-type.
data frame
rectangular data
Data frames are very useful for statistical modelling; they are objects that contain data in a tabular way. Unlike a matrix in data frame each column can contain different types of data. For example, the first column can be factorial, the second logical, and the third numerical. It is a composite data type consisting of a list of vectors of equal length.
Data frames are created using the data.frame()
function.
data.frame()
# Create the data frame.data_test <- data.frame( Name = c(“Piotr”, “Pawel”,“Paula”,“Lisa”,“Laura”), Gender = c(“Male”, “Male”,“Female”, “Female”,“Female”), Score = c(78,88,92,89,84), Age = c(42,38,26,30,35) ) print(data_test) ## Name Gender Score Age ## 1 Piotr Male 78 42 ## 2 Pawel Male 88 38 ## 3 Paula Female 92 26 ## 4 Lisa Female 89 30 ## 5 Laura Female 84 35 # The standard plot function on a data-frame (Figure 4.3) # with the pairs() function: plot(data_test)
pairs()
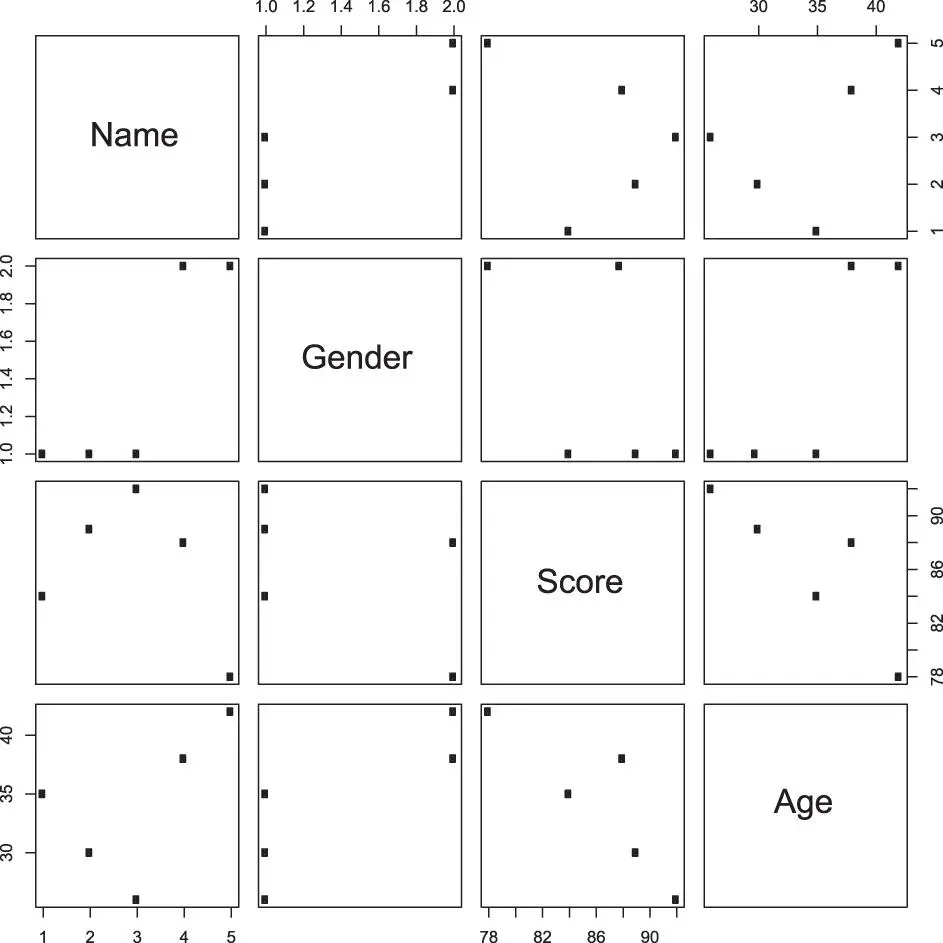
Figure 4.3 : The standard plot for a data frame in R shows each column printed in function of each other. This is useful to see correlations or how generally the data is structured.
4.3.8.2 Accessing Information from a Data Frame
Most data is rectangular, and in almost any analysis we will encounter data that is structured in a data frame. The following functions can be helpful to extract information from the data frame, investigate its structure and study the content.
summary()
head()
tail()
# Get the structure of the data frame: str(data_test) ## ‘data.frame’: 5 obs. of 4 variables: ## $ Name : Factor w/ 5 levels “Laura”,“Lisa”,..: 5 4 3 2 1 ## $ Gender: Factor w/ 2 levels “Female”,“Male”: 2 2 1 1 1 ## $ Score : num 78 88 92 89 84 ## $ Age : num 42 38 26 30 35 # Note that the names became factors (see warning below) # Get the summary of the data frame: summary(data_test) ## Name Gender Score Age ## Laura:1 Female:3 Min. :78.0 Min. :26.0 ## Lisa :1 Male :2 1st Qu.:84.0 1st Qu.:30.0 ## Paula:1 Median :88.0 Median :35.0 ## Pawel:1 Mean :86.2 Mean :34.2 ## Piotr:1 3rd Qu. :89.0 3rd Qu.:38.0 ## Max. :92.0 Max. :42.0 # Get the first rows: head(data_test) ## Name Gender Score Age ## 1 Piotr Male 78 42 ## 2 Pawel Male 88 38 ## 3 Paula Female 92 26 ## 4 Lisa Female 89 30 ## 5 Laura Female 84 35 # Get the last rows: tail(data_test) ## Name Gender Score Age ## 1 Piotr Male 78 42 ## 2 Pawel Male 88 38 ## 3 Paula Female 92 26 ## 4 Lisa Female 89 30 ## 5 Laura Female 84 35 # Extract the column 2 and 4 and keep all rowsdata_test.1 <-data_test[, c(2,4)] print(data_test.1) ## Gender Age ## 1 Male 42 ## 2 Male 38 ## 3 Female 26 ## 4 Female 30 ## 5 Female 35 # Extract columns by name and keep only selected rowsdata_test[ c(2 :4), c(2,4)] ## Gender Age ## 2 Male 38 ## 3 Female 26 ## 4 Female 30
Warning – Avoiding conversion to factors
The default behaviour of R is to convert strings to factors when a data.frame is created. Decades ago this was useful for performance reasons. Now, this is usually unwanted behaviour. a To avoid this put stringsAsFactors = FALSE
in the data.frame()
function.
d <- data.frame( Name = c(“Piotr”, “Pawel”,“Paula”,“Lisa”,“Laura”), Gender = c(“Male”, “Male”,“Female”, “Female”,“Female”), Score = c(78,88,92,89,84), Age = c(42,38,26,30,35), stringsAsFactors = FALSE ) d $Gender <- factor(d $Gender) # manually factorize gender str(d) ## ‘data.frame’: 5 obs. of 4 variables: ## $ Name : chr “Piotr” “Pawel” “Paula” “Lisa” … ## $ Gender: Factor w/ 2 levels “Female”,“Male”: 2 2 1 1 1 ## $ Score : num 78 88 92 89 84 ## $ Age : num 42 38 26 30 35
Читать дальше