Figure 1.2. Comparing 14 OFDM symbols’ TTI duration of 4G and 5G
1.2.2.2. Data throughput variability
Next, let us investigate throughput requirements of the 5G specifications. At this point, we choose to compute and show requirements per handset modem chip. Note that there are additional device classes that support only a subset of the operating modes shown here. However, an advanced handset of the future should support all of the modes shown here, to use the full potential of different frequency ranges.
Previously, we performed a specification analysis (Damjancevic et al . 2019), although over the past 6 months the 5G specifications have expanded, and here we show the updated information. In Figure 1.3 and Figure 1.4, we have organized the throughput information and presented it in a readable form for FR1 and FR2, respectively. For comparison, we plot also the 4G data throughput requirement, which coexists in FR1, along with other legacy standards. Future FR2 5G systems will coexist with Super High Frequency (SHF) and Extremely High Frequency (EHF) communication and radar systems, which are region-specific, adding an extra layer of flexibility. Throughput is shown in maximum resource blocks (RB) 6 over time, per channel BW for one spatial MIMO data stream layer 7 , 8 in compliance with active (3GPP 2019c, d) specifications. From the figures, we can observe that:
1 1) there are many possible modes of operation;
2 2) there is a 352× difference between the processing data load corners (LTE, 1.4 MHz) – lower end and (μ = 3, 400 MHz) upper end in terms of RBs.
Figure 1.3. Processing load in kRB/s for 5G NR FR1 (Damjancevic et al. 2019)
We see a greater need for flexibility again emerging from the observations, compared to the 4G standard, with many more modes to support on top of the throughput difference. Now that we have identified the throughput corners in RBs,
we can assign and calculate the smallest and highest QAM and code bit rates allowed by the 4G 9 and 5G 10 specification sets, to the lower and upper ends, respectively.
Figure 1.4. Processing load in kRB/s for 5G NR FR2
This sets the low end at 200 kb/s per 1.4 MHz BW channel and spatial data layer 11 . Note that this is a hard bit rate, and the rate at different processing steps may be higher due to oversampling.
The high end, on the other hand, is set at 2.63 Gb/s per 400 MHz BW channel and spatial data layer. Note that we have insisted on emphasizing the “per channel, per layer”, since handsets have many operating modes and some of those modes may require a multitude of channels or layers active simultaneously, for example, CA 12 . The 5G standard allows modes that support a multitude of each, further increasing the effective number of RBs communicated. The extra RBs can be used to increase the overall throughput or to increase redundancy by sending the same data on another channel frequency or layer. 5G as of now supports up to 4 × 400 MHz CA (3GPP 2019d) for its upper-end mode and MIMO up to 8 layers of data (3GPP 2019e) extension. Whether or not both extension modes can overlap within 5G is not clear, since the two are often used with opposite goals. Namely, data layer extensions conserve the spectrum and provide throughput by reusing the same spectrum on a different link, while CA extensions use excess unused spectrum to provide throughput. However, reaching 6G, we cannot dismiss the possibility that the overlap could serve as a way of increasing throughput.
With this in mind, let us make two high-end cases, first using the CA extension and the second high-end use case overlapping both the CA and multiple data layers. Calculating the first, we have 10.52 Gb/s and 84.16 Gb/s , respectively. These rates could be used, for example, for large file transfers. The difference between the low-end and the two high-end throughput corners is approximately 5 × 10 4× or 4 × 10 5×, respectively. Therefore, the system needs to deal with vastly varying data processing loads during operation, highlighting the need for flexibility of the compute engine.
1.2.2.3. Specification summary
We can now combine both the latency and data throughput requirement into low- and high-end corners on the 2D plane. Low-end use case is clear: LTE legacy 1.4 MHz . High-end, however, has two options: either the highest data of μ = 3, BW = 400 MHz or the shortest deadline μ = 4. We chose the former as the combined high-end due to nonlinear scaling of operations per data point in algorithms. For example, to compute the 4096 inverse discrete Fourier transform (IDFT) used in μ = 3, BW = 400 MHz for a single OFDM symbol, it takes more operations than to compute 2 × 2048 IDFT for two symbols used in μ = 4, BW = 400 MHz , i.e. over the same duration, there are more operations in μ = 3, BW = 400 MHz . The corner cases are presented in Table 1.1, with expression of throughput in other units as well, for a more reader-friendly overview.
Table 1.1. Processing requirement corners as per standard specification
Use Case |
Throughput |
TTI |
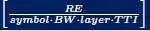 |
 |
 |
 |
 |
[ μs ] |
Low-end LTE legacy (3GPP 2019a, b) |
72 |
6 |
6 |
6 |
0.2 m |
1,000 |
CA high-end FR2 (3GPP 2019d, f) 4× CA, µ = 3,400 MHz |
3,168 |
264 |
1,056 |
8,448 |
10.52 |
125 |
MIMO CA high-end FR2 (3GPP 2019d, e, f)8×8, 4× CA, µ = 3,400 MHz |
3,168 |
264 |
8,448 |
67,584 |
84.16 |
125 |
1.2.3. Outcome of workloads
We see that the 3GPP specifications follow the trend and vision of 5G laid out in section 1.2.1, incorporating the variability of workloads as the central paradigm.
With throughput requirements varying by several orders of magnitude, a homogeneous HW solution would be very inefficient for both high-end and low-end use cases. Rather, a heterogeneous HW architecture that is a mixture of HW accelerator engines, banks of programmable processing elements and supporting memory systems would be efficient. Accelerator engines such as dedicated (application-specific) HW accelerators and ASIPs are ideal to deal with extreme high-end use cases and easy-to-scale low-varying algorithms or processing steps, due to their speed and efficient energy per data point consumption. While banks of programmable processing elements such as vDSPs (SIMD cores with signal processing-oriented instruction set architecture ) and generic scalar reduced instruction set computer (RISC) cores are ideal to deal with moderate–high to low-end use cases and processing steps that require flexibility, for example, choosing from a set which algorithm to perform, based on the device’s situational parameters and environmental conditions. Such HW is well suited for dealing with highly variable loads by powering HW modules on and off based on the current load. For example, if enough compute resources are available on the vDSPs, i.e. available idle cycles, we could run the communication kernels on the vDSPs in a time-multiplexed manner and keep the HW accelerators off.
Читать дальше