where y:= D 1/2 yand the graph spectral filter is defined as
Moreover,
for the symmetric normalized graph Laplacian; therefore, it acts as a linear decay low-pass filter in the graph frequency domain.
This graph spectral representation of a pixel-dependent filter suggests that the pixel-dependent filter W implicitly and simultaneously designs the underlying graph (and therefore, the GFT basis) and the spectral response of the graph filter . In other words, the GSP expression of the pixel-dependent filter is free to design the spectral response
, apart from the linear decay one, once we determine W. For example, let us consider the following spectral response:
[1.33] 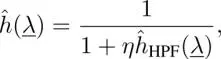
where
is an arbitrary graph high-pass filter and η > 0 is a parameter. In this case,
works as a graph low-pass filter and its spectral shape is controlled by
In fact, Gadde et al . (2013) show that equation [1.33]is the optimal solution for the following signal restoration problem:
[1.34] 
where z= x+ nwith additive noise nand
.
Image filtering sometimes needs numerous iterations to smooth out the details, in case of textured and/or noisy images. Therefore, to boost up the smoothing effect, the trilateral filter method (Choudhury and Tumblin 2003) first smooths the gradients of the image, and subsequently, the smoothed gradient is utilized to smooth the intensities. Its counterpart in the graph spectral domain is also proposed in Onuki et al. (2016) with the parameter optimization method for ρ in equation [1.33], which minimizes MSE after denoising it.
Figure 1.3. Image denoising example using bilateral filters. From left to right: Original, noisy (PSNR: 20.02 dB), bilateral filter in the pixel domain (PSNR: 26.23 dB), and bilateral filter in the graph frequency domain (PSNR: 27.14 dB). Both bilateral filters use the same parameters
Figure 1.3depicts an example of image denoising by the bilateral filter in the graph frequency domain (Gadde et al . 2013). The image is degraded by additive white Gaussian noise. The bilateral filter in the graph frequency domain uses the spectral filter parameterized in equation [1.33], with ĥ HPF= λ and η = 5. It is clear that the graph spectral version efficiently removes noise while preserving image edges.
1.5. Multiple graph filters: graph filter banks
In the previous sections, we only considered the case where a single graph spectral filter was applied. Several image processing applications, such as compression and restoration, often require multiple filters that have different passbands (typically low-pass and high-pass). This signal processing system – so-called filter banks – is also important for GSP. In this section, the spectral domain design of graph filter banks is briefly introduced.
Figure 1.4. Framework of graph filter bank
A typical framework of a graph filter bank is illustrated in Figure 1.4. The analysis transform decomposes the input signal into some graph frequency components using a set of graph filters { h k( L)} ( k = 0,..., M − 1). We assume that the graph operator is a graph Laplacian L; however, in general, any graph operator can be applied. The decomposed coefficients (called transformed coefficients ) are often downsampled by the sampling matrix
, where M kis the sampling ratio, to reduce the number of coefficients. As a result, the transformed coefficients in each subband are represented as
[1.35] 
The entire analysis transform is given as follows:
[1.36] 
The size of
is often called the redundancy of the transform. The redundancies of transforms are classified as follows:
– ρ = 1: critically sampled transform. The number of transformed coefficients is the same as N, i.e. the number of elements in x.
– ρ > 1: oversampled transform. The number of transformed coefficients is larger than N.
– ρ < 1: undersampled transform. The number of transformed coefficients is smaller than N.
If S k= I N, i.e. no sampling is performed, ρ = M , and the transform is called an undecimated transform. In general, undersampled transforms will lose the information of the original signal. They cannot recover the original signal xfrom the transformed coefficients.
After the analysis transformation, an arbitrary linear and nonlinear operation is performed to c kfor a target application. For example, small magnitude elements in c kare thresholded to denoise or compress the signal. Let us denote
as processed coefficients.
Читать дальше