2.10 Smart Sensing and Mobile Computing
Sensor‐enabled mobile devices have been widely used for various applications (Guihai et al. 2015 ). Real‐time people‐counting techniques through event tracking and mobile crowd‐sensing give business owners and operators a new channel to communicate with their customers. Internet and smartphone applications, enable a person's location to be followed anywhere in the globe almost in real time through navigation satellites. A mobile phone listens to the relative positions of different GPS satellites and is able determine its location on a map. The mobile location data is transferred to cloud servers via cell towers, or Wi‐Fi or Bluetooth connection ( Figure 2.9). Cloud servers track and analyze the anonymous location data, and give back the gathered history and live data to mobile applications. One of the well‐known GPS applications is the car navigator, which shows the location of the car on the map and advanced applications suggest car routes without traffic jams. A user can see the suggested route on the map and follow his location on the map when he moves on the route (Google 2019 ). One can also follow another person's (mobile's) location on a map in real time with the other person's permission. Some applications also count the relative passenger numbers on defined map locations and track visit durations. Train applications can use, e.g. GPS ‐based Automatic Vehicle Routing ( AVR ) with APC (Trimet 2019 ). They support travellers in planning their trips and can give on‐board passenger information and real‐time information of route‐related matters (Passengera 2019 ).
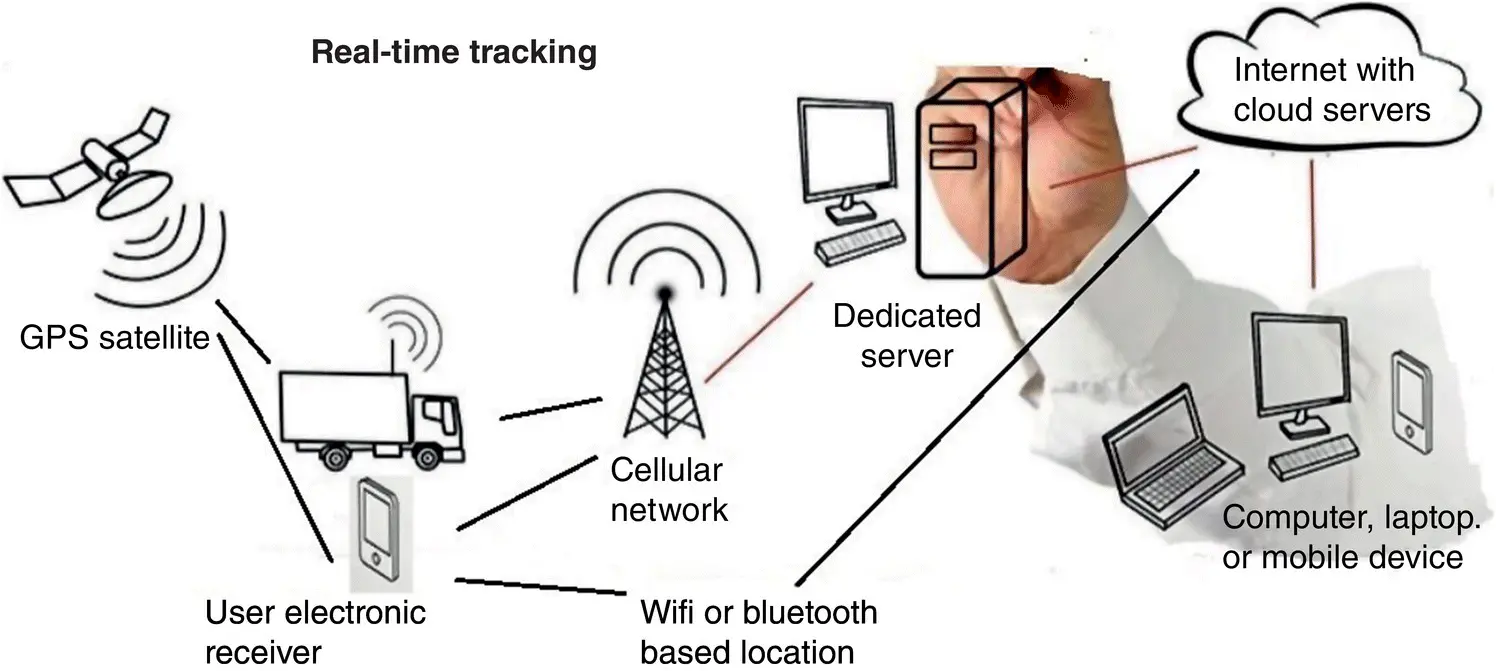
Figure 2.9 Modern user real‐time tracking service.
Inside buildings a user mobile application may not hear the GPS signals and it is not possible to use it in indoor wayfinding. Indoor Positioning Systems (IPS) that can locate people inside the buildings include sensors based on radio waves, magnetic fields, acoustic signals, or mobile phones. Transition from outdoor navigation to indoor navigation can be completely seamless. With an open Wi‐Fi or Bluetooth connection from a mobile phone to Internet, the user location can be determined, e.g. in airports or big city buildings. The indoor navigation platform is interfaced with the positioning system of the building, and it is possible to create building floor layouts with an IPS application. The user is then able to follow his own real time position on the floor map as shown in Figure 2.10, or to use location‐based services such as push messaging. Passenger crowding can be shown by heat maps. Needless to say that there are plenty of indoor navigation providers (mapspeople 2019 ; visioglobe 2019 ; proximi.io 2019 ). Wayfinding is one of the future applications that improves user experience when moving inside of different types of buildings. The challenge is the vertical positioning where elevators are essential in transporting passengers. To acquire comprehensive people flow on floors requires information of the building levels, which most probably will be solved by the future navigation systems.
Figure 2.10 Wayfinding by a mobile phone
( Source: proximi.io (2019 )).
Example 2.1 Figure 2.6shows the elevator load during an elevator stop. Let us assume car arrival load is 90 kg, minimum load is 10 kg, and departure load 75 kg. How many passenger transfers, P , during a stop are counted when using the three above mentioned, load‐based passenger counting methods.
First method: P = (90–75)/75 = 0.2 ≈ 0 transfers
The first method divides the difference of arrival and departure load by the average passenger weight and suggests no passenger transfers .
Second method: P exit = (90–10)/75 = 1.07 ≈ 1 and P enter = (75–10)/75 = 0.87 ≈ 0
The second method calculates the difference of arrival and minimum load for exiting passengers, and the difference of departure and minimum load for entering passengers. This method suggests that one passenger has exited the car .
Third method: P exit = 2 and P enter = 3 .
The third method counts all stepwise changes during a stop. The load has decreased two times and increased three times during the stop. This method suggests totally five passenger transfers during the elevator stop being the most accurate of the three methods .
3 Passenger Arrival Process in Buildings
3.1 Introduction
The passenger arrival process, i.e. the passenger inter‐arrival times when arriving in the elevator lobbies, is reviewed in this chapter. The difference between uniform, Poisson and compound batch Poisson arrivals is shown in Figure 3.1where the arrival rate is five persons in five minutes (Siikonen 2016). In uniform arrivals, passenger inter‐arrival time is constant. In the Poisson process, passengers arrive randomly in the lobbies and their inter‐arrival times vary. Over a longer time period, here 15 minutes, the arrival rate can stay constant. The third example shows passengers moving in small groups, i.e. batches. The inter‐arrival times of passenger batches are random and exponentially distributed.
Figure 3.1 Passenger inter‐arrival times in elevator lobbies.
Table 3.1 X 2‐test results for passenger entering through the security gates, office in Warsaw.
Time of day |
Arrival interval (s) |
X 2 |
Degrees of freedom |
X 2 0.95 |
X 2 0.05 |
Suitability of H 0‐hypothesis |
Daily 7:00–19:00 |
10 |
2241 |
7 |
2.2 |
14.1 |
Poor |
30 |
1632 |
11 |
4.6 |
19.7 |
Poor |
60 |
3120 |
11 |
4.6 |
19.7 |
Poor |
300 |
548 |
15 |
7.3 |
25.0 |
Poor |
8:00–9:00 |
10 |
63 |
10 |
3.9 |
18.3 |
Poor |
30 |
250 |
15 |
7.3 |
25.0 |
Poor |
60 |
12.6 |
14 |
6.6 |
23.7 |
Good |
300 |
208 |
15 |
7.3 |
25.0 |
Poor |
17:00–18:00 |
10 |
9.9 |
3 |
0.4 |
7.8 |
Poor |
30 |
4.3 |
4 |
0.7 |
9.5 |
Good |
60 |
9.4 |
6 |
1.6 |
12.6 |
Good |
300 |
9.5 |
13 |
5.9 |
22.4 |
Good |
Social behaviour affects the passenger arrival process. The arrival process can be observed manually on the entrance floors, but the data security gates also give the arrival rate information. Passenger trip from origin to destination floor is random but follows building population distribution on each floor and the utilization of floors, and it varies according to the traffic pattern at different times of day. Statistics of traffic patterns during the day can be measured automatically by elevator control systems or external devices where passengers are counted using the methods described in Chapter 2. Individual passenger journeys from the origin floor to the destination can be followed by the destination or access control system, or other ID‐based systems. From the measured origin–destination data, forecasts can be made to improve passenger service.
Читать дальше