The analysis is comprehensive as the full spectrum of PIM ®profile incidents is examined; in other words, the PIM ®profile is not a number but a 16-element vector. Thus, two proteins have the same PIM ®profile if their 16-element vectors are equal.
Its use as part of a biochip is not yet completed; this restricts its use only to determine the predominant function of a protein; however, nowadays, it is not enough to identify the function/structure of a protein but to identify it in the blood of an organism and determine its number.
This will enable the PIM R profile as a rapid detection test.
3.5.4 SARS-CoV-2 Recognition Using PIM® Profile
The PIM ®system ( Section 3.5.1) was determined in the four SARS-CoV-2 structural proteins: spike, membrane, envelope, and nucleocapsid ( Section 3.3.3), and their smooth curves ( Figure 3.1) were plotted, it was observed that there is a similarity in these PIM ®profiles, except for the region between the polar interactions [P −, P −] and [N, P −] see ( Figure 3.2).

Figure 3.1 Relative frequency distribution of proteins that express the four SARS-CoV-2 structural viral protein group represented by “smooth curves”. Graphs were produced using EXCEL software. The X-axis represents the 16 charge/polarity interactions. The ellipse shows the region where curves do not match the trend.
Figure 3.2shows the PIM ®profile of the spike and envelope proteins behaving particularly differently, while the membrane is the translation of nucleocapsid.
A revision of the histograms ( Figure 3.3) of the relative frequency distribution of the residues in the sequences of the SARS-CoV-2 structural proteins (spike, envelope, membrane, and nucleocapsid) shows that any of them are similar. When in general, this behavior does not necessarily depend on the length of the sequence.
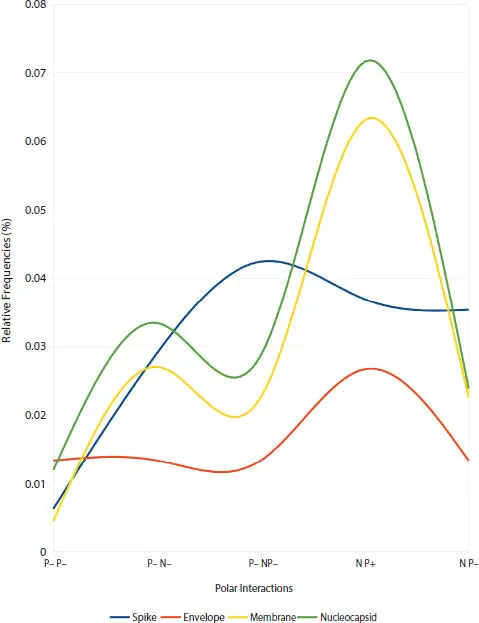
Figure 3.2 Zoom over the Figure 3.1. The X-axis represents the five polar interactions from [P−, P−] and [N, P−]. See ( Section 2.5.4).
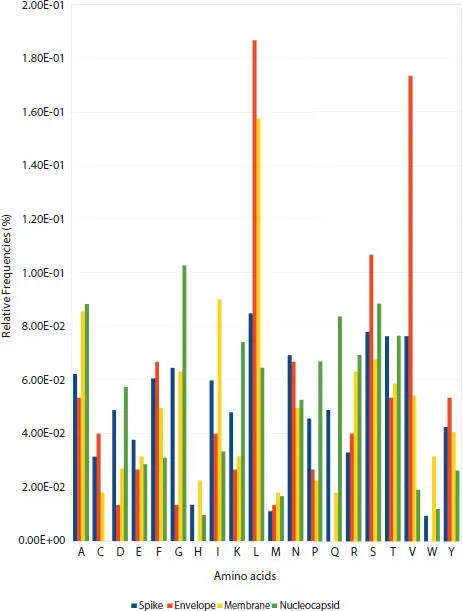
Figure 3.3 Histograms SARS-CoV-2 structural proteins.
Non-supervised algorithms will play a major role in proteomics and genomics applications, as they can be run regardless of the time consumed in the computational platform; we have to bear in mind that, in most cases, the time they will take to search a specific pattern is unknown since the algorithms are non-linear metrics, and therefore, it is not possible to scale the processing time.
All non-supervised algorithms do not depend on any human intervention, so they run freely on a platform until they cast some conclusion. These processes may well be supported by a Hidden Markov Model (HMM) that enables the total control of all processes.
On the other hand, we consider that it will be necessary to reassess the metrics of the prediction programs, to explore differently the evaluation of proteins, both those stored in databases, and those identified in organic fluids that require a quick evaluation.
Metrics may also thoroughly explore only one characteristic and not several, like the method here presented, as we think it will be essential in the analysis of the new generation of algorithms, i.e., if the metric yields a number, it is very little information it provides; however, if it gives a vector, or a matrix, then the information can be considered exhaustive.
The proteomic and genomic for the production of pharmaceutical drugs will rapidly change to the design of biochips that will assess the proteins in organic fluids; this is a very important task, particularly for the latent threat the Coronaviridae family represents to humans due to its ability to disseminate and mutate.
A final observation about this subject is the advantage of the stochastic algorithm over the deterministic algorithm. Although the latter is more precise, they hardly offer any practical solution when the number of factors is large since the prediction of a biological microorganism is a multifactorial phenomenon, a deterministic algorithm will require huge memory and processing speed. Therefore, they will hardly provide a practical solution, as they will face the limitations of any computational architecture.
On the other hand, the stochastic functions are linear, which means that when evaluating multiple variables, the complexity will not increase, so these functions only give a probability value associated with the result, i.e., the associated value will be in the range between 0% a 100%.
The authors thank C. Celis-Juárez and L. Anderson-Coe for proof-reading. Funding: None. Contributions: Theoretical conceptualization and design: CP. Performance: CP. Data analysis: CP, GVA, and MFM. Discussion: CP, GVA, and MFM. Competing interests: We declare there are no financial and personal interests with other people or organizations that could inappropriately influence this work. Data availability: Copyright & Trademark. All rights reserved (México), 2018: Polarity Index Method ®. Software and Hardware: Hardware: The computational platform used was two HP Workstations z21400 — CMT — 4 x Intel Xeon E3-1270/3.4 GHz (Quad-Core) — RAM 8/4 GB — SSD 1 x 160 GB — DVD SuperMulti — Quadro 2000 — Gigabit LAN, Linux Fedora 64-bits. Cache Memory 8 MB. Cache Per Processor 8 MB. RAM 8/4. Software: Polarity Index Method (PIM ®). Supplementary Materials can be asked to ( polanco@unam.mx).
1. CDC, Human coronavirus types, National Center for Immunization and Respiratory Diseases (NCIRD), Division of Viral Diseases, Human coronarivus types section. Available at: https://www.cdc.gov/coronavirus/types.html. Last Updated February 15, 2020. Accessed November 22, 2021. 2020, https://www.cdc.gov/coronavirus/types.html.
2. Wikipedia contributors. Coronavirus. Wikipedia, The Free Encyclopedia. November 20, 2021, 05:45 UTC. Available at: https://en.wikipedia.org/w/index.php?title=Coronavirus&oldid=1056173146. Accessed November 22, 2021.
3. Wikipedia contributors. Human coronavirus NL63. Wikipedia, The Free Encyclopedia. August 22, 2021, 00:48 UTC. Available at: https://en.wikipedia.org/w/index.php?title=Human_coronavirus_NL63&oldid=1039988099. Accessed November 22, 2021.
4. World Health Organizacion, Middle East respiratory syndrome coronavirus (MERS-CoV) section, Available at: https://www.who.int/news-room/factsheets/detail/middle-east-respiratory-syndrome-coronavirus-(mers-cov). March 11, 2019. Accessed: November 22, 2021.
5. Wikipedia contributors. Human coronavirus HKU1 [Internet]. Wikipedia, The Free Encyclopedia; 2021 Nov 17, 12:27 UTC [cited 2021 Nov 22]. Available from: https://en.wikipedia.org/w/index.php?title=Human_coronavirus_HKU1&oldid=1055721823. Accessed: November 22, 2022.
6. World Health Organizacion, Severe Acute Respiratory Syndrome (SARS) section, Available at: https://www.who.int/health-topics/severe-acute-respiratory-syndrome#tab=tab_1. March 11, 2019. Accessed: November 22, 2021.
7. National Center for Immunization and Respiratory Diseases (NCIRD), Division of Viral Diseases, Coronavirus disease (COVID-19) section. Available at: https://www.who.int/health-topics/coronavirus#tab=tab3. Last Updated February 15, 2020. Accessed November 22, 2021.
Читать дальше