2.4 Experimental Results and Analysis
The term “tissue characteristics” covers a wide range of meanings, from qualitative assessment to various scientific measurements. In the extensive literature available, we expand the definition of terms, show the relationship between tissue features and images, identify some relevant physical parameters, and briefly describe the subject’s medical history. Excerpts are provided to explain how.
2.4.1 Tissue Characterization and Risk Stratification
According to an article titled “Automatic classification of lung cancer from cellular images using deep symmetric neural networks”, we evaluated three types of cancer and trained for classification. However, pictures from the same sample belonged to the same group.
While executing cross-validation algorithm in different sets of images, we found that, in Set 1, there are 28 items and respective cross-validation score is 5,280 for adenocarcinoma. There are 42 items and cross-validation score is 5478 for squamous cell carcinoma. For small cell carcinoma, there are 26 images and cross-validation score is 5,070.
In Set 2, there are 28 items and respective cross-validation score is 5,184 for adenocarcinoma. There are 37 items and cross-validation score is 5,220 for squamous cell carcinoma. For small cell carcinoma, there are 33 images and cross-validation score is 5,280.
In Set 2, there are 26 items and respective cross-validation score is 5,040 for adenocarcinoma. There are 46 items and cross-validation score is 5,310 for squamous cell carcinoma. For small cell carcinoma, there are 32 images and cross-validation score is 5,214.
2.4.2 Samples of Cancer Data and Analysis
Figures 2.2and 2.3show a sample of cancer types correctly classified and classified using the data amplification method. The classification confusion matrix. Squamous cell carcinoma is often mistaken for adenocarcinoma.
After successfully applying classification model in this dataset, we have to test the accuracy, precision, and recall of the model. So, we observed the confusion matrix whose results are as follows: actual and predicted value of adenocarcinoma is 89% matched and only 11% items are not predicted correctly. Squamous cell carcinoma is predicted correctly 60% which is less as compared to adenocarcinoma. Actual and predicted value of small cell carcinoma is 70% matched and only 30% items are not predicted correctly.
It shows the original image classification and the wide range of image accuracy, respectively. Results obtained using magnification images show that the classification accuracy for adenocarcinoma, squamous cell carcinoma, and small cell cancer is 89.0%, 60.0% and 70.3%, respectively, and the overall corrected rate is 71.1%. In addition, apply plug-in to improve the classification.
Finally, we improved our classification model and accuracy score of trained set and augmented sets are given below:
Adenocarcinoma predicted 73% original images of lung cancer, whereas 89% for augmented images. We found that 45% original images are correctly classified and 60% augmented images are classified for squamous cell carcinoma. Small cell carcinoma is classified correctly 75% for original images and 70% for augmented images.
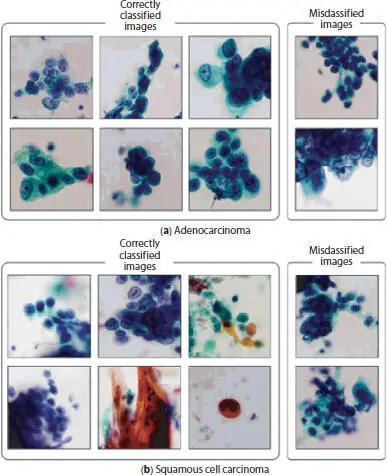
Figure 2.2 Sample images of correctly classified and misclassified carcinoma.
Figure 2.3 More sample images of correctly classified and misclassified carcinoma.
In the three types of lung cancer, the efficiency of the unsupervised clustering was maximum in adenocarcinoma and minimum in squamous cell carcinoma. Squamous cell adenocarcinoma requires more images. DCNN helped to correctly classify 70% of lung cancer cells.
AI will not only identify and predict at-risk patients but will also be a large dataset available to hospitals and healthcare providers to identify changes in patient health and medical outcomes, as well as accurate diagnoses. There are cases that use faster and personal support.
Treatment plan especially is provided in the manuscript for patients suffering with chronic diseases. AI information on infection states depends on learning calculations and doctor experienced in the treatment of patients with indications, signs, conclusions, medicines, and comparative results.
Most clinical data fall into a wide range of limited categories, although they are distant and may be limited by potential sampling, but future studies on the adoption of approved standards are not required. This allows AI algorithms to learn information and enhance the creation of reinforcement learning loops.
2.6 Future Scope, Limitations, and Possible Applications
The forthcoming examination is required to investigate how AI can customize treatment choices for singular patients to a clinician. The nature of data that AI gains from is likewise significant and an expected boundary to the far-reaching selection of exactness medication. The size of data needed for deep learning and the variety of strategies utilized makes it hard to obtain an away from of how precisely AI frameworks may function in genuine practice or how reproducible they might be in various clinical contexts.
Forthcoming exploration openings are necessary to address “social inclination” in AI calculations and sufficient advances should be taken to abstain from compounding medical care differences when utilizing AI apparatuses to save patients are famous. Tolerant security should be ensured and more noteworthy straightforwardness into algorithmic. Fairness is expected to guarantee acknowledgment of AI by suppliers and patients.
IoT solutions for healthcare that collect, transmit, and visualize data in complex intelligent systems via wearable and field sensor networks can facilitate analytics, activity detection, and decision-making. AI and ML technologies play a significant role in this transition, but their implementation requires computational power. It is often only available using cloud services.
In fact, with the increasing amount of data generated by sensors, the performance of ML-based cloud processing has several weaknesses for various reasons.
2.7 Recommendations and Consideration
AI in healthcare is ready to bring change and disrupt medical care. While not giving up marketing and profitability of drug addiction is the wisest guide, it balances AI, the need for comprehensive healthcare to plan and manage and reduce potential unexpected consequences.
It is wise to take. For AI, the best solution is to start with a real healthcare issue, involving the relevant stakeholders, first-line users, patients, and their families (including artificial and non-AI options). You need to find a solution and work on it. It is implemented and extended by our five goals: better health, better care experience, doctor health, lower cost, and common rights.
The nature of administration is significantly influenced by the nature of your internet association, making it difficult to use. Healthcare providers require shorter response times to address potential health risks, especially when performance such as early detection, risk prevention, and activity diagnosis is guaranteed in real time.
Читать дальше