Bioinformatics and Medical Applications
Здесь есть возможность читать онлайн «Bioinformatics and Medical Applications» — ознакомительный отрывок электронной книги совершенно бесплатно, а после прочтения отрывка купить полную версию. В некоторых случаях можно слушать аудио, скачать через торрент в формате fb2 и присутствует краткое содержание. Жанр: unrecognised, на английском языке. Описание произведения, (предисловие) а так же отзывы посетителей доступны на портале библиотеки ЛибКат.
- Название:Bioinformatics and Medical Applications
- Автор:
- Жанр:
- Год:неизвестен
- ISBN:нет данных
- Рейтинг книги:5 / 5. Голосов: 1
-
Избранное:Добавить в избранное
- Отзывы:
-
Ваша оценка:
- 100
- 1
- 2
- 3
- 4
- 5
Bioinformatics and Medical Applications: краткое содержание, описание и аннотация
Предлагаем к чтению аннотацию, описание, краткое содержание или предисловие (зависит от того, что написал сам автор книги «Bioinformatics and Medical Applications»). Если вы не нашли необходимую информацию о книге — напишите в комментариях, мы постараемся отыскать её.
The main topics addressed in this book are big data analytics problems in bioinformatics research such as microarray data analysis, sequence analysis, genomics-based analytics, disease network analysis, techniques for big data analytics, and health information technology.
Audience Bioinformatics and Medical Applications: Big Data Using Deep Learning Algorithms
Bioinformatics and Medical Applications — читать онлайн ознакомительный отрывок
Ниже представлен текст книги, разбитый по страницам. Система сохранения места последней прочитанной страницы, позволяет с удобством читать онлайн бесплатно книгу «Bioinformatics and Medical Applications», без необходимости каждый раз заново искать на чём Вы остановились. Поставьте закладку, и сможете в любой момент перейти на страницу, на которой закончили чтение.
Интервал:
Закладка:
This article outlines the main approaches to lung cancer prediction to date and highlights some of the relative strengths and weaknesses. They discuss some of the challenges of developing and validating such technologies, as well as clinical acceptance strategies. They review the main approaches used to classify lymph nodes and predict lung cancer from CT imaging data. In our experience, using the right training data and using a comprehensive CNN, achieving classification performance in regions with low 90s AUC points and sufficient training data [9].
According to Choi H. and Na KJ in this study, a gene correlation network, we created a risk classification model for lung adenocarcinoma. An extension of future research is the use of this method in concurrent networks of cancer progression. Advances in technology change the DL design and the way toward choosing delegate qualities to improve expectation exactness. They found that NetScore was related with sex, status of smoking, phase, and sub-atomic subtype. In summary, a high NetScore trend in men, smokers, and KRAS mutants was delayed and observed to be positive [6].
Finally, they expected future clinical trials designed with all around controlled clinical and obsessive factors to help find clinical applications for their new danger grouping models.
Yin Li and his team members have predicted the risk of lung adenocarcinoma (LUAD) is important in determining subsequent treatment strategies. Molecular biomarkers may improve risk classification for LUAD [11].
Yin Li et al. analyzed the gene expression profile of LUAD patients by atlas cancer genome (TCGA) and omnibus gene expression (GEO) analysis. They first evaluated the prognostic relationship for each gene using three separate algorithms: Notable Function, Random Forest, and Variable Coke Regression. Next, survival-related genes were included in the LASSO minimum and selection function models to create a LUAD risk prediction model [17].
They initially identified large dataset significant survival-related genes. A hybrid strategy was used to identify key genes associated with survival in large datasets. Enhancement analysis showed an association of these genes with tumor development and progression. A risk prediction model was created using the LASSO method. The risk model was approved with two outside sets and one free set. Patients in the high-hazard bunch had a lower danger of repeat (RFS) and in general endurance (OS) than patients at low risk. We also created a registry that predicts LUAD patient operating systems, including models and risk stages.
Hence, they conclude risk models may serve as a pragmatic and reliable predictor of LUAD and may provide new experiences into the atomic instruments of infection [11, 16].
The paper was written by Francisco Azuaje titled as “Artificial intelligence for precision oncology: beyond patient stratification” [1].
Francisco Azuaje described axial data from medical conditions and treatment options as a key challenge for accurate oncology. AI offers an unparalleled opportunity to enhance such predictive capabilities in laboratories and clinics. AI, including ML, which is the most well-known area of research, has been able to accurately identify tumors beyond relatively well-known detection patterns such as single-source omics and supervised classification of imaging datasets.
According to him, this perspective, major developments, and challenges in this regard argue that the scope and depth of AI research should be expanded in order to achieve geological advances in accurate oncology [1].
According to Xu J [20] in a large era of data on cancer genetics, wide availability of genetic information provided by next-generation sequencing techniques and rapid development of medical journals integrates AI approaches such as ML, detailed learning, and natural language processing to challenge big data and high-dimensional scalability and uses this method to process clinical data to handle big data. It brings the knowledge that you have. It is bent, using the base. Open and lie down, it is really medicine.
In this paper, they reviewed the current status and future guideline for using AI in cancer genomics in the field of workflow is genomic analysis for accurate cancer treatment. Existing AI solutions and their limitations in genetic testing for cancer and its diagnosis, including various contacts and interpretations, are being considered.
The tools or common algorithms available for the leading NLP technologies in literature extraction are reviewed and compared to evidence-based clinical recommendations.
According to him, this paper deals with data needs and algorithm transparency, the importance of preparing patients and physicians for real-time reproduction and assessment, and modern digital healthcare. They believe that AI is the main factor in the evolution of healthcare into a precise drug but of the precedent that needs to be created to ensure safety and beneficial effects on healthcare [20].
2.3 Proposed Systems
Based on histo-pathological image input, there are two steps:
• Model building: The shape of this model is based on the extraction function.
• Model Evaluation: Form biological communication.
Modeling attempts to preserve the extracted shape by extracting shape-based features with full focus on the shape of the model. The fixed size is considered a constraint, and it focuses on all other faces found to achieve that form of the constraint, making it easy to extract the entire model.
2.3.1 Framework or Architecture of the Work
However, model evaluation includes some of the biological significance of the form, which requires the physician to have the precise and accurate information needed to evaluate the form.
2.3.2 Model Steps and Parameters
Here, we will focus more on size-based clinical models. There are several steps like segmentation, dimension reduction, sampling, enhancement, and active contour mapping and classification of the objects which are involved that help you to design your model in a very efficient way (as per Figure 2.1).
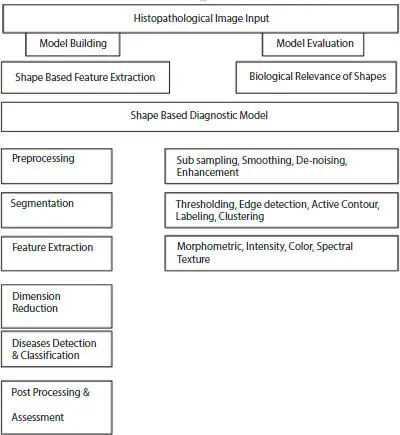
Figure 2.1 Framework of the experimental study of lung cancer stratification.
2.3.3 Discussions
Cancer has been the reason for death for a very long time. A little less than half of the cancer happens in individuals matured ≤70 to 85 years. The maximum death prone cancer is lung cancer where it threatens the all type of aged persons from adolescents to middle age to adults. Those who are addicted with drinking alcohol or smoking or females who were nonsmoking, all type of subjects were included in the list of casualties unfortunately from this illness.
Thus, it is needed to separate lung cancer based on risk included (highrisk, okay, and rising-risk). This investigation depends on building up an arrangement conspire for lung cancers through examining minuscule images and ordering them utilizing Deep Convolution Neural Network (DCNN), which is an AI (ML) structure.
Microscopic images of tissues will be characterized on the basis of risk involved using DCNN. Convolution neural networks are deep artificial neural networks that are utilized fundamentally for ordering images, group them by likenesses (photograph search), and perform object recognition inside the scenes. They are the calculations that can recognize faces, tumors, people, road signs, and numerous different parts of the graphic information.
The effectiveness of convolution networks in image recognition is one of the main reasons why the world has woken up for the productivity of deep learning. They are fueling significant progress in computer vision (CV), which has clear applications for self-driving vehicles, mechanical technology, security, clinical judgments, and medicines for the outwardly impaired.
Читать дальшеИнтервал:
Закладка:
Похожие книги на «Bioinformatics and Medical Applications»
Представляем Вашему вниманию похожие книги на «Bioinformatics and Medical Applications» списком для выбора. Мы отобрали схожую по названию и смыслу литературу в надежде предоставить читателям больше вариантов отыскать новые, интересные, ещё непрочитанные произведения.
Обсуждение, отзывы о книге «Bioinformatics and Medical Applications» и просто собственные мнения читателей. Оставьте ваши комментарии, напишите, что Вы думаете о произведении, его смысле или главных героях. Укажите что конкретно понравилось, а что нет, и почему Вы так считаете.