While energy detection is a natural outcome of signal decoding, building specialized hardware for spectrum sensing can perform energy detection in different ways. This specialized hardware, which is sometimes referred to as an augmented sensor, may or may not have prior knowledge of the signal bases. The following subsections show different methods that can be utilized by the augmented sensors to perform energy detection.
2.3.2 Time Domain Energy Detection
With this technique, the spectrum sensor has to rely on using bandpass filters. The spectrum sensor is given a center frequency f 0and a bandwidth W to define the frequency range to sense. The spectrum sensor inputs the signal s ( t ) through the bandpass filter followed by a squaring device and an integrator, as shown in Figure 2.5. The details of how to build such a sensor are beyond the scope of this book. However, these sensors have the ability to define bandpass filters for any given center frequency f 0and any given bandwidth W in the broad‐spectrum range they are designed for. These sensors also have the ability to perform energy detection on a wide band of frequency divided into smaller sub‐bands in parallel.
Figure 2.5Time domain energy detection.
Notice the importance of T in the integrator in Figure 2.5. A signal with weak power spectral density such as a spread spectrum signal would needs a longer time period T . With augmented sensors, the bandpass filter has a critical transfer function that can be expressed as follows:
(2.2) 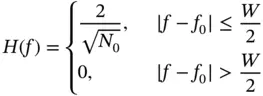
The reason for emphasizing Equation (2.2)is that the augmented sensor needs to estimate the noise one‐sided power spectral density N 0, which is a challenge when the augmented spectrum sensor may have no prior knowledge of the signal it is sensing and hence has no means to directly estimate the noise floor, as in the case of same‐channel in‐band sensing. Instead, the augmented sensor relies on normalizing for the noise power and uses this normalization to compute the probability of a false alarm and the probability of detection as detailed in Chapter 3. If we conceptualize how the sensor creates an energy detection sample every T seconds, then in a large number of samples we have a high probability that the signal being sensed was not transmitted during the entire time period T . Thus, the noise floor becomes the energy sample collected with the minimum energy detection. The importance of Equation (2.2) is that it expresses the noise one‐sided power spectral density that correlates to the noise floor.
In time domain spectrum sensing, the time duration that the sensed signal remaining in a particular state can affect the outcome of the spectrum sensor. This time duration is referred to as the dwell time . The spectrum sensor observation time length should correlate to dwell time. Chapter 3 shows that one advantage of same‐channel in‐band sensing is that the in‐band signal is sensed during a known state and the sensing technique does not observe the signal during multiple states within a single sensing window. That is, the sensing technique can have knowledge of whether the transmitted signal is present or not during the entire sensing window. On the other hand, augmented sensors using time domain energy detection, where the sensed signal may change state during the observation time, can lend a higher probability of false alarm.
2.3.3 Frequency Domain Energy Detection
With this energy detection technique, the sensor also has to be configured for a center frequency f 0and a bandwidth W to define the frequency range to sense. The sensor uses a bandpass filter, as with time domain energy detection, followed by an analog‐to‐digital convertor (ADC) to digitize the signal and FFT to convert the signal to the frequency domain ( Figure 2.6). The squaring device calculates the energy per each frequency coefficient and the mean value stage is used to calculate the average energy over the observed frequency band.
Figure 2.6Frequency domain energy detection.
As with time domain energy detection, frequency domain energy detection has to consider the presence of noise. The method used to estimate the noise power spectral density can rely on discrete Fourier transformation (DFT) where the digitized data is divided into segments and a sliding window is used to estimate the average noise spectral density. One reason to choose frequency domain energy detection over time domain energy detection in augmented sensors is the higher accuracy of noise estimation but the price for that is the need for more computational power.
Notice how with the three energy detection techniques covered so far, the outcome is simple:
1 Signal energy level at the defined carrier frequency f0 and bandwidth W, and
2 Noise floor energy at the same carrier and bandwidth.
It is important to note that the hypothesizing and decision‐making processes covered in Chapter 3 can be tricky under certain circumstances, such as fading channels. While frequency domain energy detection can implement good techniques such as the sliding window explained above, distributed and centralized DSA techniques can have a view of spectrum sensing that is more comprehensive than a local node. Distributed and centralized DSA techniques can analyze spectrum sensing information per RF neighbor and further overcome the uncertainty that can result from fading channels.
While Section 2.3.1introduced same‐channel in‐band sensing, Sections 2.3.2and 3.3.3 introduced the most common forms of augmented sensing where the augmented sensor can be configured to sense a frequency band defined by the carrier frequency f 0and bandwidth W as illustrated in Figures 2.4and 2.5. Augmented sensors can be built to sense a wide band of frequencies and sense multiple sub‐bands each defined by a carrier frequency f 0and a bandwidth W simultaneously. This simultaneous sensing utilizes parallel paths where each path starts with a bandpass filter configured for the carrier frequency f 0and bandwidth W for one of sub‐bands being sensed.
2.4 Signal Characteristics Spectrum Sensing
There are different signal characteristics that a spectrum sensor can detect. Here, we go beyond simple energy detection with no prior knowledge of the signal being sensed to having some prior knowledge of the signal and the ability to synthesize the detected signal to extract more information.
2.4.1 Matched Filter Based Spectrum Sensing
This technique requires pre‐knowledge of many aspects of the sensed signal such as bandwidth, operating frequency, modulation type and order, pulse shaping, and frame format. The spectrum sensor can quickly detect the presence of the sensed signal with high accuracy. This technique can be used before discovering more detailed signal characteristics such as spreading code and hopping pattern.
The matched filter will accentuate the targeted signal S ( t ) and will suppress other signals and noise. Notice that signals other than the targeted signal S ( t ) are essentially noise with respect to S ( t ). The impact of the suppressed signals and noise are referred to as W ( t ). The design of this matched filter includes:
Читать дальше