Below is code for the first graph. To create the next graph, you will have to create a new variable, the natural logarithm of newspapersper1000.
df <- read.csv("WorldBankData.csv") # "df" is the data frame. plot(df$newspapersper1000,df$lifeexp,xlab="Newspapers per1000", ylab="Life Expectancy",pch=19,cex.axis=1.5,cex.lab=1.15) abline(lm(lifeexp∼newspapersper1000,data=df),lty=2) lines(lowess(df$newspapersper1000,df$lifeexp)) plot(log(df$newspapersper1000),df$lifeexp,xlab="log(Newspapers per1000)",ylab="Life Expectancy",pch=19,cex.axis=1.5, cex.lab=1.15) abline(lm(lifeexp∼log(newspapersper1000),data=df),lty=2) lines(lowess(log(df$newspapersper1000),df$lifeexp))
To analyze these data, we can run a regression of life expectancy (LE) in years against the natural logarithm of the number of newspapers per 1000 persons (LN) for a large number of countries in a given year. The results are
(1.1) 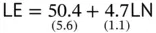
where standard errors are in parentheses, so both the coefficients have very high
‐statistics and are significant. This means that there is a relationship between life expectancy and the number of newspapers per 1000 people. But does this show that a country having more newspapers leads to longer lives for its citizens? Common sense says probably not. The natural logarithm of the number of newspapers is probably a proxy for other variables that drive life expectancy; countries that can afford newspapers can probably also afford better food, housing, and medical services. What we are observing is most likely a mere correlation, and, unfortunately, this sort of observational analysis should not be interpreted as causal .
Run the above simple regression. You should get the same coefficients and standard errors.
A better analysis would add more variables to the regression to “control” for other factors. So, let's try adding other variables that we expect to drive life expectancy: LHB (natural logarithm of the number of hospital beds per 1000 in the country), LP (natural logarithm of the number of physicians per 1000 in the country), IS (an index of improvements in sanitation), and IW (an index of improvements in water supply). Since we don't believe that newspapers cause longer life expectancy, we would expect that once we include these variables in the regression, the coefficient on LN will be reduced. The results are
(1.2) 
The coefficient on LN has not gone to zero; in fact, it hasn't changed much. The coefficients on all but one of the other variables that we know affect life expectancy are insignificant. What are we to make of this?
Run the above multiple regression. You should get the same coefficients and standard errors. Be sure you understand why the variables LN and LHB are “significant” while the others are not.
In reality, life expectancy is affected by a large set of variables in a complex way, and the natural logarithm of newspapers is a good proxy for these other variables. If we have some beliefs about which variables are more likely to be the true causes of an increase in life expectancy, we might be able to build a model that we think represents the cause and effect relationships. If we really want to find the causal effect of newspapers, we might also try more sophisticated methods that involve trying to find and compare countries that are similar in all respects except the number of newspapers per 1000 people. This sort of analysis leads into “the garden of forking paths,” a phrase used by the statistician Andrew Gelman (who specializes in causal inference) to describe the many decisions a researcher may take that can lead the researcher to unknowingly reaching spurious statistical conclusions.
For example, if an analyst analyzing the above data actually added variables and dropped variables until LN was insignificant and some other health‐related variables all were significant, she would have taken a trip through the garden of forking paths and would come up with a useless model. The model would be useless because she tested many hypotheses on the same set of data and her “results” almost assuredly are contaminated by false positives (i.e. type I errors): she thinks the coefficients are significant when they're really not.
To better illustrate this idea, let us have 10 covariates (independent variables) to use in building a model to describe a particular dependent variable, and we will be allowed to include anywhere from 1 to all 10 of the variables. For each variable there is a decision (fork) to include or exclude the variable. Then there are
possible models to choose from. A researcher just tries a sufficient number of models, dropping and including variables, until
at which point she freezes the model, and the choice of variables to include is justified after the variables have been included. Even a researcher who does not deliberately try all possible models still will make choices about including and excluding variables (“I thought X1 would be significant, but it wasn't, so I dropped it and tried X2”), which implies that her model is but one of many possible models that she just happened to select. Because of the garden of forking paths, a seemingly objective analysis is really quite subjective, and causality cannot be determined from subjective analyses.
The purpose of this example is to drive home the point that, in general, observational data simply are not up to the task of answering causal questions. In this book, we focus on an alternative approach to answering business questions, which is to conduct experiments.
We do not suggest that observational studies have no valid uses. To the contrary, there are many situations when experiments are not possible, and in such cases, there is no alternative to the use of observational data:
Sometimes it is impossible to run an experiment. For example, it would be unethical to randomly assign people to smoke versus not smoke, so our understanding of the causal relationship between smoking and cancer was built on observational data. (However, it took a long time to convince everyone, since observational data is easy to question.)
If you want to build a new store, it is foolish to construct several stores in random locations to test hypotheses about where to locate stores.
Establishing causality is not always necessary, and documenting correlations is sometimes sufficient for the purpose at hand. In fact, the whole field of “predictive analytics” focuses on prediction problems, where causality is not important. For example, if we are predicting defaults on mortgages, very often we only need to know the probability that a person will default, not the causal factors that determine the probability of default; correlation is sufficient, and causation is not necessary.
The outcome of interest is sufficiently rare that running an experiment with a large enough number of trials is expensive. Perhaps you can only afford a sample size of 100, but the response rate is 2%; you're never going to get a good estimate of the response rate with such a comparatively small sample.
Читать дальше