In terms of uncertainty propagation, it is typically achieved for an orthorectangular uncertainty structure using interval propagation for each focal element, using the methods mentioned in section 1.5for interval analysis.
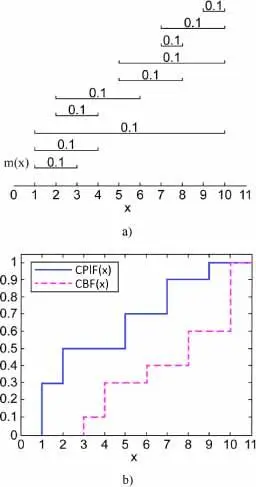
Figure 1.12. Illustrations of (a) a BPA function m(x); (b) the corresponding cumulative plausibility function (CPlF) and cumulative belief function (CBF)
1.8.2. Rules for combining belief mass functions
Evidence theory is based on the definition of a structure of uncertainties in the form of masses attributed to different focal elements. The definition of this uncertainty structure comes from experts. Analogously, as in the framework of possibility theory, rules have been defined in order to be able to aggregate different and sometimes divergent sources of uncertainty, for example, from different experts.
Let m1 and m2 denote two BPA functions with the respective sets Ci and Cj as focal elements. These functions can be aggregated according to the following rules:
– the Dempster rule, defined by:[1.22]The denominator reflects the conflict between the two sources through normalization. Note that therefore only the agreement between sources is retained in the aggregate uncertainty structure. A disadvantage of this rule is in the way it deals with divergent opinions and it is preferable to apply this rule only in cases where there is reasonable agreement between sources;
– the Yager rule, defined by:[1.23][1.24][1.25][1.26]The Yager rule attributes the conflict to the entire universe Ω, thus increasing the degree of ignorance associated with it;
– the weighted mean rule:
[1.27] 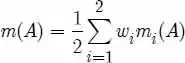
In this rule, the conflict is simply averaged with a weighting based on the confidence attributed to each expert. Other rules, as well as variations around the three presented, have been proposed and the reader can refer to Sentz and Ferson (2002) for more information.
1.9. Evaluation of epistemic uncertainty modeling
The quantification of epistemic uncertainties is a major challenge for achieving an integral framework for the quantification and propagation of uncertainties in industrial problems. Depending on the nature of the lack of knowledge, these uncertainties can be modeled in different ways. In some situations, epistemic uncertainties may be due to insufficient sampling, in which case the probabilistic framework, through the use of probability boxes ( p-boxes ), is probably the most relevant framework.
In other situations, no samples may be available and obtaining them would be too time consuming or costly, so only expert opinion can provide information on the uncertainties involved. Some consider that such advice cannot be objective and does not need to be mixed with rigorous, sample-based uncertainty quantification within a probabilistic framework. Others consider that, although imperfect, because it is subjective, expert opinions can contain useful information for uncertainty quantification and, rather than ignoring them, have focused on developing theories in order to be able to model the uncertainties expressed in this way. This is particularly the case with the theories of possibility and evidence that we have reviewed. Basically, both theories have the same objective, which is to bound an unknown probability distribution function by the construction of an uncertainty structure based on expert opinion. It is worth noting, however, that possibility theory is based on axioms which are quite different from those of probability theory, which can sometimes lead to counterintuitive results. These axioms also imply a relatively wide bounding for the unknown probability distribution function. Evidence theory, on the other hand, allows a narrower bounding for the distribution function, provided that it is possible to construct BPA functions with a very fine granularity. It also does not involve the more constraining axioms of possibility theory. To conclude, let us also note that in most real problems, epistemic and aleatory uncertainty modeling must be simultaneously considered. This combination of different approaches remains a challenge, especially from the point of view of the associated numerical costs.
Apostolakis, G. (1999). The distinction between aleatory and epistemic uncertainties is important: An example from the inclusion of aging effects into PSA. Proceedings of PSA ‘99, International Topical Meeting on Probabilistic Safety Assessment , 135–142.
Dempster, A.P. (1967). Upper and lower probabilities induced by a multivalued mapping. Ann. Math. Stat ., 38, 325–339.
Der Kiureghian, A. and Ditlevsen, O. (2009). Aleatory or epistemic? Does it matter? Structural Safety , 31(2), 105–112.
Dubois, D. and Prade, H. (1992). Combination of fuzzy information in the framework of possibility theory. In Data Fusion in Robotics and Machine Intelligence , Della Riccia, G., Lenz, H.J. and Fruse, R. (eds). Academic Press, New York.
Dvoretzky, A., Kiefer, J. and Wolfowitz, J. (1956). Asymptotic minimax character of the sample distribution function and of the classical multinomial estimator. The Annals of Mathematical Statistics , 27(3), 642–669.
Ferson, S., Joslyn, C.A., Helton, J.C., Oberkampf, W.L. and Sentz, K. (2004). Summary from the epistemic uncertainty workshop: Consensus amid diversity. Reliability Engineering and System Safety , 85, 355–369.
Ferson, S., Kreinovich, V., Grinzburg, L., Myers, D. and Sentz, K. (2015). Constructing probability boxes and Dempster–Shafer structures. Technical report, SAND-2015-4166J, Sandia National Lab, Albuquerque.
Hansen, E. and Walster, G.W. (2003). Global Optimization Using Interval Analysis: Revised and Expanded . CRC Press, Boca Raton.
Hoffman, F.O. and Hammonds, J.S. (1994). Propagation of uncertainty in risk assessments: The need to distinguish between uncertainty due to lack of knowledge and uncertainty due to variability. Risk Analysis , 14(5), 707–712.
Klir, G.J. (2004). Generalized information theory: Aims, results, and open problems. Reliability Engineering and System Safety , 85, 355–369.
Kreinovich, V. and Xiang, G. (2008). Fast algorithms for computing statistics under interval uncertainty: An overview. In Interval/Probabilistic Uncertainty and Non-classical Logics , Huynh, V.N., Nakamori, Y., Ono, H., Lawry, J., Kreinovich, V., Nguyen, H.T. (eds). Springer, Berlin.
Lemaire, M. (2014). Mechanics and Uncertainty . ISTE Ltd, London and John Wiley & Sons, New York.
National Research Council (2009). Evaluation of Quantification of Margins and Uncertainties Methodology for Assessing and Certifying the Reliability of the Nuclear Stockpile . The National Academies Press, Washington, D.C.
Nikolaidis, E., Chen, S., Cudney, H., Hatftka, R.T. and Rosca, R. (2004). Comparison of probability and possibility for design against catastrophic failure under uncertainty. Journal of Mechanical Design , 126(3), 386–394.
Oberguggenberger, M., King, J. and Schmelzer, B. (2009). Classical and imprecise probability methods for sensitivity analysis in engineering: A case study. International Journal of Approximate Reasoning , 50(4), 680–693.
Читать дальше