Regarding Neuromarketing techniques, we read up n the recent research that linked EEG signals with predicting consumer behavior and emotions on self-reported ratings.
The correlation between neurons’ activities and the decision-making process is studied [7] during shopping have extensively been exploited to ascertain the bond between brain mapping and decision-making while visualizing a supermarket. The participants were asked to select one of every 3 brands after an interval of 90 stops. They discovered improvement in choice-predictions brand-wise. They also established significant correla-tions between right-parietal cortex activation with the participant’s previous experience with the brand.
The researchers [8] explored the Neuro-signals of 18 participants while evaluating products for like/dislike. It also incorporated eye-tracking methodology for recording participant’s choice from set of 3 images and capturing Neuro-signals at the same time. They implemented PCA and FFT for preprocessing the EEG data. After processing mutual data amongst preference and various EEG bands, they noticed major activity in “theta bands” in the frontal, occipital and parietal lobes.
The authors [9] tried to analyze and predict the 10 participant’s preference regarding consumer products in a visualization scenario. In the next procedure, the products were grouped into pair and presented to participants which recorded increase frequencies on mid frontal lobe and also studied the theta band EEG signals correlating to the products.
It has implemented an application-oriented solution [10] for footwear retailing industry which put forward the pre-market prediction system using EEG data to forecast demand. They recorded 40 consumers in store while viewing the products, evaluating them and asked to label it as bought/not with an additional rating-based questionnaire. They concluded that 80–60% of accuracy was achieved while classifying products into the 2 categories.
A suggestive system [11] was created based on EEG signal analysis platform which coupled pre and post buying ratings in a virtual 3D product display. Here, they factored the emotions of the subjects by analyzing beta and alpha EEG signals.
The authors created a preference prediction system [12] for automobile brands in a portable form while conducting trial on 12 participants as they watched the promotional ad. The Laplacian filter and Butterworth band pass was implemented for preprocessing and 3 tactical features—“Power-Spectral Density”, “Spectral Energy” and “Spectral Centroid” was procured from alpha band. The prediction was done by “K-Nearest Neighbor” and “Probabilistic Neural Network” classification with 96% accuracy.
They used the scenario of predicting the consumer’s choice based on EEG signal analysis [13] while viewing the trailers which resulted in finding significant gamma and beta high frequencies with high correlation to participants and average preferences.
Participants were assessed on self-arousal and valence features while watching particular scenes in a movie [14]. They analyzed the data while factoring in 5 peripheral physiological signals relating them to movie’s content-based features which inferred that they can be used to categorize and rank the videos.
Here 19 participants were shown 2 colors for an interval of 1 s and during the time EEG oscillations were analyzed [15] on Neural mechanisms for correlations of color preferences.
They had 18 participants who were subjected to a set of choices and analyzed their Neuro-activity and Eye-tracking activity to brain-map regions associated with decision making and inter-dependence of regions for the said task [16]. They concluded with high synchronization amongst frontal lobe and occipital lobe giving major frequencies in theta, alpha and beta waves.
They are trying to establish a bond between Neuro-signals and the learning capacity of a model software [17] while assuming that the model has the capability to train itself for dominant alpha wave participants.
“Independent Component Analysis (ICA)” to separate multivariate signals coming from 120 channels of electro-cortical activity [18]. This was done to convert those signals into additive subcomponents. Patterns of sensory impulses were recorded which matched movement of the body.
They have used filter is as a stabilizing and filtering element in the ECG data of 26 volunteers and then applied Approximate Entropy on it for inter-subject evaluation of data as the part of a retrospective approach [19] while adding truthfulness to Entropy windows for its stable distribution. This filter is very extensively being used in Signal processing which led us to adopt it.
The study [20] is an experiment on ECG signals of 26 participants where approximate entropy method is implemented for examining the concentration. Approximation entropy window was taken less for intra-patient comparing to inter-patient and for filtering the noisy signals S-Golay method was implemented.
They have innovatively preprocessed the ECG signal using S–Golay filter technique [21]. With both quadratic degrees of smoothing and differentiation filter methods combinedly has processed ECG signals having sampling rate 500 Hz with seventeen points length.
A very unique “double-class motor imaginary Brain Computer Interface” was implemented with Recurrent Quantum Neural Network model for filtering EEG signals [22].
In the paper [23] using the S-Golay filter, the artifacts due to blinking of eyes are found out and it is eliminated adapting a noise removal method.
3.3 Methodology
3.3.1 Bagging Decision Tree Classifier
Among the many Machine Learning algorithms, this method forms a group of algorithms where several instances are created of black-box estimators on variable subsets from the base training set after which we aggregate their solo predictions to form a resultant prediction. This process is used as a path to minimize the variance of the foundation estimator i.e. a decision tree by including randomization within its creation process and building an ensemble from it. In multiple scenarios, this method consists a simple path to improve with regard to a single model, avoids making it a necessity to acclimatize to a foundation algorithm. It works best with fully developed decision trees as it reduces overfitting in comparison to boosting methods which generally work best in shallow decision trees. This classifier comes in many flavors but majorly differ from each other by the path that they draw variable subsets of the training set. In our case samples were extracted with replacement called as Bagging.
3.3.2 Gaussian Naïve Bayes Classifier
This classifier is based on probability which is combined within a Machine Learning model. Hence, it is based on “Bayes Theorem” which states that, we can derive the probability of an event1 given that a retrospective event2 has happened. Here, event2 is the witness and event1 is the hypothesis. The assumption here is that the features are non-dependent which means that the existence of one feature does not affect the other which is why it’s called Naïve. When predictions allocate a continuous value without being discrete, we can ascertain that those values are derived from gaussian distribution. Following is the general formula for Bayes theorem (3.1).
(3.1) 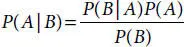
Since our case has a different set or input, our formula for this implementation changes to Equation (3.2).
(3.2) 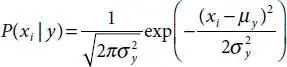
Читать дальше