Stephen Winters-Hilt - Informatics and Machine Learning
Здесь есть возможность читать онлайн «Stephen Winters-Hilt - Informatics and Machine Learning» — ознакомительный отрывок электронной книги совершенно бесплатно, а после прочтения отрывка купить полную версию. В некоторых случаях можно слушать аудио, скачать через торрент в формате fb2 и присутствует краткое содержание. Жанр: unrecognised, на английском языке. Описание произведения, (предисловие) а так же отзывы посетителей доступны на портале библиотеки ЛибКат.
- Название:Informatics and Machine Learning
- Автор:
- Жанр:
- Год:неизвестен
- ISBN:нет данных
- Рейтинг книги:3 / 5. Голосов: 1
-
Избранное:Добавить в избранное
- Отзывы:
-
Ваша оценка:
- 60
- 1
- 2
- 3
- 4
- 5
Informatics and Machine Learning: краткое содержание, описание и аннотация
Предлагаем к чтению аннотацию, описание, краткое содержание или предисловие (зависит от того, что написал сам автор книги «Informatics and Machine Learning»). Если вы не нашли необходимую информацию о книге — напишите в комментариях, мы постараемся отыскать её.
Discover a thorough exploration of how to use computational, algorithmic, statistical, and informatics methods to analyze digital data Informatics and Machine Learning: From Martingales to Metaheuristics
ad hoc, ab initio
Informatics and Machine Learning: From Martingales to Metaheuristics
Informatics and Machine Learning — читать онлайн ознакомительный отрывок
Ниже представлен текст книги, разбитый по страницам. Система сохранения места последней прочитанной страницы, позволяет с удобством читать онлайн бесплатно книгу «Informatics and Machine Learning», без необходимости каждый раз заново искать на чём Вы остановились. Поставьте закладку, и сможете в любой момент перейти на страницу, на которой закончили чтение.
Интервал:
Закладка:
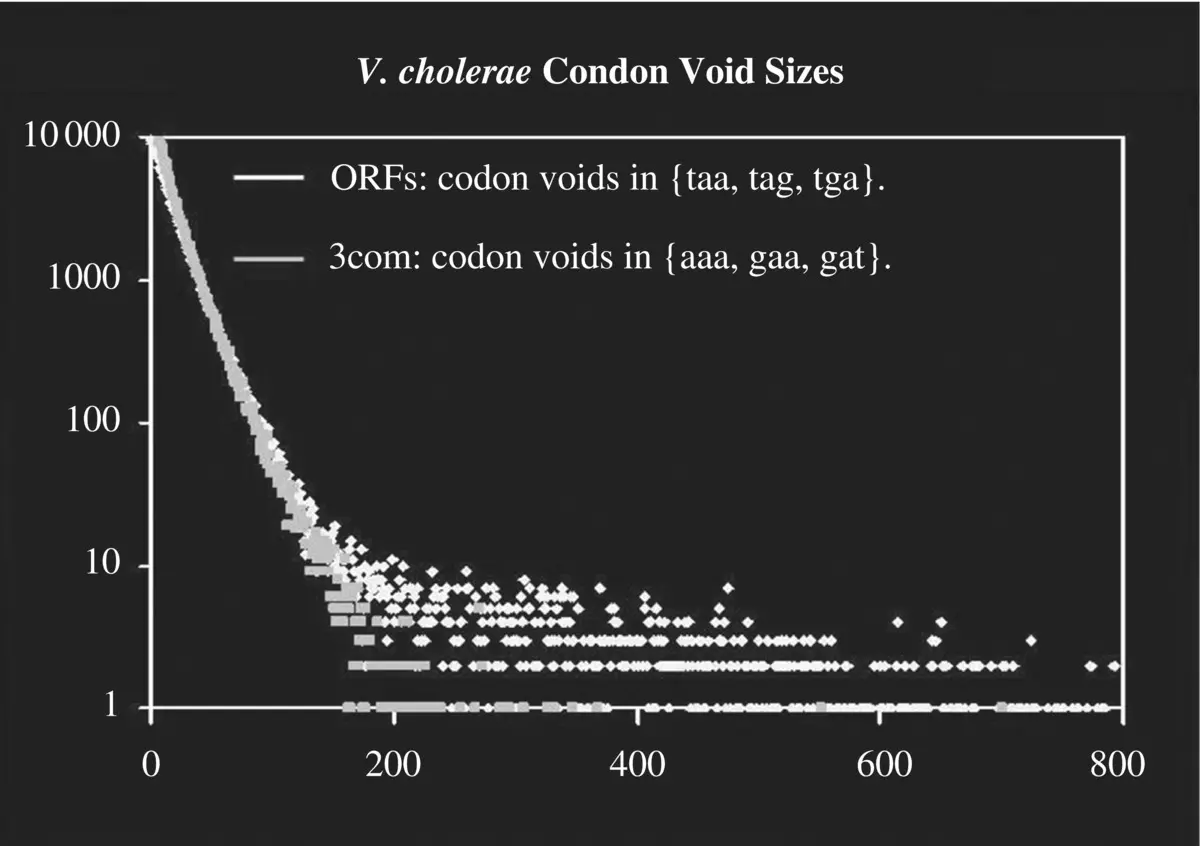
Figure 3.2 ORF encoding structure is revealed in the V. cholera genome by gaps between stop codons in the genomic sequence. X ‐axis shows the size of the gap in codon count between reference codons (stops for conventional ORFs, or 3com set for comparisons in table), Y ‐axis shows the counts.
3.3 ORF Discovery from Long‐Tail Distribution Anomaly
Once codon grouping is revealed, where a frequency analysis on codons on the stop codons (TAA, TAG, TGA) shows they are rare. Focusing on the stop codons it is easily found that the gaps between stop codons can be quite anomalous compared to the gaps between other codons (see prog2.py addendum 6):
------------------- prog2.py addendum 6 --------------------- # need gap stats between codons, the stop codon group (orf_finder), and # the 'common' reference group (corf_finder): def orf_finder ( seq, frame ): gapcounts = {} edgecounts = {} pattern = '[acgtACGT]' result = re.findall(pattern, seq) seqlen = len(seq) output_fh = open("orf_output", 'w') output_fh.close() oldindex=0 oldcodon="" for index in range(frame,seqlen-2): rem = (index+3-frame)%3 if rem!=0: continue codon = result[index]+result[index+1]+result[index+2] if (codon!="TAA" and codon!="TAG" and codon!="TGA"): continue else: gap = index - oldindex if gap%3!=0: print "gap=", gap, "index=", index break quant = 100 bin = gap/quant if oldindex!=0: if bin in gapcounts: gapcounts[bin]+=1 else: gapcounts[bin]=1 if oldcodon!="": edge=oldcodon + codon if edge in edgecounts: edgecounts[edge]+=1 else: edgecounts[edge]=1 slice = result[oldindex: index+2+1] output_fh = open("orf_output", 'a') slicejoin = "" slicejoin = slicejoin.join(slice) orfline = slicejoin + '\n' output_fh.write(orfline) oldindex=index oldcodon=codon npcounts = np.empty((0)) for i in sorted(gapcounts): npcounts = np.append(npcounts,gapcounts[i]+0.0) print "gapbin", i, "count =", gapcounts[i] ecounts = np.empty((0)) for i in sorted(edgecounts): ecounts = np.append(ecounts,edgecounts[i]+0.0) print "edgecodon", i, "count =", edgecounts[i] probs = count_to_freq(npcounts) #usage: orf_finder(EC_sequence,0) # def corf_finder ( seq, frame ): # same except not 'stop' boundariy condition but 'common': # if (codon!="AAA" and codon!="GAA" and codon!="GAT") #usage: #corf_finder(EC_sequence,0) --------------- prog2.py addendum 6 end ---------------------
ORFs are “open reading frames,” where the reference to what is open is lack of encounter with a stop codon when traversing the genome with a particular codon framing, e.g. ORFs are regions devoid of stop codons when traversed with the codon framing choice of the ORF. When referring to ORFs in most of the analysis we refer to ORFs of length 300 bases or greater. The restriction to larger ORFs is due to their highly anomalous occurrences and likely biological encoding origin (see Figure 3.2), e.g. the long ORFs give a strong indication of containing the coding region of a gene. By restricting to transcripts with ORFs >= 300 in length we have a resulting pool of transcripts that are mostly true coding transcripts.
The above example shows a bootstrap finite state automaton (FSA) process on genomic data: first scan through the genomic data base‐by‐base and obtain counts on nucleotide pairs with different gap sizes between the nucleotides observed [1, 3]. This then allows a mutual information analysis on the nucleotide pairs taken at the different gap sizes. What is found for prokaryotic genomes (with their highly dense gene placement), is a clear signal indicating anomalous statistical linkages on bases three apart [1, 3]. What is discovered thereby is codon structure, where the coding information comes in groups of three bases. Knowing this, a bootstrap analysis of the 64 possible 3‐base groupings can then be done, at which point the anomalously low counts on “stop” codons is then observed. Upon identification of the stop codons their placement (topology) in the genome can then be examined and it is found that their counts are anomalously low because there are large stretches of regions with no stop codon (e.g. there are stop codon “voids,” known as “ORFs”). The codon void topologies are examined in a comparative genomic analysis in [1, 3]. As noted previously, the stop codons, which should occur every 21 codons on average if DNA sequence data was random, are sometimes not seen for stretches of several hundred codons (see Figure 3.2).
Not surprisingly, longer genes stand out clearly in this process, since their anomalous, clearly nonrandom DNA sequence, is being maintained as such, and not randomized by mutation, (as this would be selected against in the survival of the organism that is dependent on the gene revealed).
The preceding basic analysis can provide a gene‐finder on prokaryotic genomes that comprises a one‐page Python script that can perform with 90–99% accuracy depending on the prokaryotic genome. A second page of Python coding to introduce a “filter,” along the lines of the bootstrap learning process mentioned above, leads to an ab initio prokaryotic gene‐predictor with 98.0–99.9% accuracy. Python code to accomplish this is shown in what follows ( Chapter 4). In this process, all that is used is the raw genomic data (with its highly structured intrinsic statistics) and methods for identifying statistical anomalies and informatics structural anomalies: (i) anomalously high mutual information is identified (revealing codon structure); (ii) anomalously high (or low) statistics on an attribute or event is then identified (low stop codon counts, lengthy stop codon voids); then anomalously high sub‐sequences (binding site motifs) are found in the neighborhood of the identified ORFs (used in the filter).
3.3.1 Ab initio Learning with smORF’s, Holistic Modeling, and Bootstrap Learning
In work on prokaryotic gene prediction ( V. cholera in what follows), a program (smORF) was developed for an extended ORF characterization (to characterize “some more ORFs” with different trinucleotide delimiters than stops). Using that software with a simple start‐of‐coding heuristic it was possible to establish good gene prediction for ORFs of length greater than 500 nucleotides. The smORF gene identification was used in a bootstrap gene‐annotation process (where no initial training data was provided). Part of the functionality for smORF is encompassed in prog2.py program described thus far. The strength of the gene identification was then improved by use of a gap‐interpolating‐Markov‐model (gIMM’s to be described in Section 3.4). When applied to the identified coding regions (most of the >500 length ORFs), six gIMMs were used (one for each frame of the codons, with forward and backward read senses). If poorly gIMM‐scoring coding regions were rejected, performance improved, with results slightly better than those of the early Glimmer gene‐prediction software [125] , where an interpolating Markov model was used (but not generalized to permit gaps). More recent versions of Glimmer incorporate start‐codon modeling in order to strengthen predictions. One of the benefits of the gap‐interpolating generalization is that it permits regulatory motifs to be identified, particularly those sharing a common positional alignment with the start‐of‐coding region. Using the bootstrap‐identified genes from the smORF‐based gene‐prediction (including mis‐calls) as a training set permitted an unsupervised search for upstream regulatory structure. The classic Shine‐Dalgarno sequence (the ribosome binding site) was found to be the strongest signal in the 30‐base window upstream from the start codon. Similar results will be found with the full gene‐finder example in Chapter 4.
Читать дальшеИнтервал:
Закладка:
Похожие книги на «Informatics and Machine Learning»
Представляем Вашему вниманию похожие книги на «Informatics and Machine Learning» списком для выбора. Мы отобрали схожую по названию и смыслу литературу в надежде предоставить читателям больше вариантов отыскать новые, интересные, ещё непрочитанные произведения.
Обсуждение, отзывы о книге «Informatics and Machine Learning» и просто собственные мнения читателей. Оставьте ваши комментарии, напишите, что Вы думаете о произведении, его смысле или главных героях. Укажите что конкретно понравилось, а что нет, и почему Вы так считаете.