where
b ≡ bias value.
2.3.3 ANN With Continuous Characteristics
This model is also the extension of McCulloch-Pitts neuron model. Two stages are used to illustrate ANN with continuous characteristics. The schematic diagram of the model is presented in Figure 2.5. The linear combination of input values is calculated in the first stage. The weight value associated with each value of the input array lies between 0 and 1. The summation function can be expressed as σ in Equation (2.4).
(2.4) 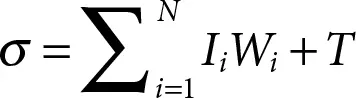
where
T ≡ extra input value associated with weight value 1 which represents the threshold or bias of a neuron.
Figure 2.3 Linear threshold function.
Figure 2.4 Schematic diagram of linear threshold gate.
The second stage of the model is the activation function which takes the sum-of-product value as the input and produces the output. The activity of this stage determines the characteristic of the ANN model. This function compresses the amplitude of the output so that it lies in the range of [0, 1] or [−1, 1]. The compression of the output signal is performed to mimic the signal produced by biological neuron in the form of continuous action-potential spikes.
The function used in the above discussed model is semi-linear and termed as logistic sigmoid function. The graphical depiction of the function is presented in Figure 2.6. The mathematical demonstration of logistic sigmoid function is presented in Equation (2.5).
(2.5) 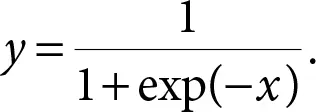
Figure 2.5 ANN model with continuous characteristics.
Figure 2.6 Graphical representation of logistic sigmoid function.
From the graphical representation, it is clear that for the large positive value of input x, the output value y tends to 1. On the other hand, for the negative value of x, y tends to 0. Again, if x approaches to −∞ or ∞, the slope of the graph becomes 0. The increment of slope occurs as x goes close to 0. These characteristics of the sigmoid graph are very crucial in ANN.
2.3.4 Single-Layer Neural Network
It is the simplest form of neural network model containing only one layer of input nodes which receives the weighted input and send it to the subsequent layer of receiving nodes. In some cases, there may be only one neuron exists at the receiving end. Even a single neuron of ANN has astonishing computational capability. As the activity of the input is limited to receiving and passing of input signal and it does not perform any computation, thus only true layer of neuron is single-layer network is the output layer. The basic model of single layer neural network is shown in Figure 2.7. The yellow nodes denote the input layer which receives x 1, x 2, x 3, ……, xN as the input and send it to the output layer represented by green nodes. Each of the nodes in the input layer is connected with each node in the output layer through the connection weight. If the weight value is zero, then indicates no connection between the nodes. The output nodes calculate the output values and generate the output y 1, y 2, y 3, ……, yN . Because of only one layer of link between the input and output, this model is termed as single layer network. It can only learn linear functions. The model shown in Figure 2.7is fully connected.
2.3.5 Multilayer Neural Network
It has more computational capabilities than the previously discussed single layer neural network. Apart from single-input layer and single-output layer, it has one or more hidden layers depending on the complexity of the computational problem. Unlike to single-layer network, multi-layer network can learn non-linear functions. Computations on the weighted inputs are performed in the hidden layers and generate the net input on which activation functions are applied to produce the actual output.
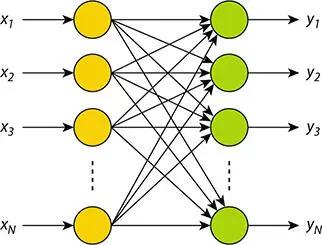
Figure 2.7 Single layer neural network.
Figure 2.8 Multilayer neural network.
Figure 2.8represents a model of fully connected multi-layer neural network. In this structure, the input layer shown by yellow nodes receive the inputs x 1, x 2, x 3, … …, xN and pass it to the first hidden layer, represented by gray nodes in Figure 2.8. If the model has multiple hidden layers, then the output from the first hidden layer is passed to the next hidden layer and so on. Finally, when the output from the last hidden layer reaches to the output layer represented by the green nodes, it produces the final output y 1, y 2, y 3, … …, yN . It can be said that the multi-layer network consists of a number of single layer network arrange in a cascading manner.
Learning is an impressive characteristic of human brain. But, the research on exact process of learning in human nervous system is still in its primary stage. The scientists claim that, learning in biological system occurs due to some alteration of neural structure and synaptic connections. ANN replicates the learning process of human nervous system and the ability to learn is one of the most unique features of ANN. The learning algorithms adjust the weighted connections between the neurons of neural network. The sequence of events occurs during learning process is as follows:
1 1. The environment stimulates the network in which it is embedded.
2 2. As a result of stimulation, the free parameters of the network get altered.
3 3. The system generates response to the environment in an enhanced way.
The purpose of the learning algorithm is to find an appropriate set of weight matrices so that it can generate output efficiently after mapping any input. The three classes of learning processes are listed below.
1 1. Supervised Learning: In this case, the learning algorithm provides the desired output along with the given input while training the ANN. Because of the input-output pair, the neural model becomes capable of calculating the error based on the target vs. actual output. Based on the calculated error, the model can be corrected by adjusting the weights.
Читать дальше