1.4.2 Bernoulli’s Restricted Boltzmann Machines
A restricted Boltzmann machine (RBM) is a generative stochastic artificial neural network which learns a probability distribution over its set of inputs. Please see Figure 1.6.
Bernoulli’s RBM has binary type of hidden and visible units hi and vi, respectively, and a matrix of weights w. It also has bias weights ai and bi for visible and hidden units, respectively. With these, the energy equation can be written as follows:
(1.1) 
The probability distribution over the hidden and visible layers in terms of energy is as follows:
(1.2) 
Z is a normalizing constant just to make the sum of all probabilities equal to 1.
The conditional probability of h given v is as follows:
(1.3) 
The conditional probability of v given h is as follows:
(1.4) 
The individual activation probabilities are as follows:
(1.5) 
(1.6) 
For ANN, the results are as follows.
For ANN model with one hidden layer, the accuracy vs. epochs plot ( Figure 1.7).
For ANN model with two hidden layers, the accuracy vs. epochs plot ( Figure 1.8).
For ANN model with three hidden layers, the accuracy vs. epochs plot ( Figure 1.9).
For ANN model with four hidden layers, the accuracy vs. epochs plot ( Figure 1.10).
The accuracy vs. number of hidden layers in ANN plot Figure (1.11).
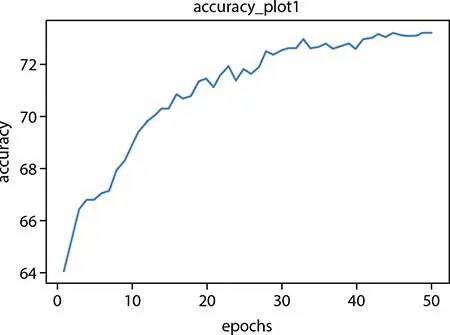
Figure 1.7 Accuracy plot for one hidden layer–based ANN.
Figure 1.8 Accuracy plot for two hidden layer–based ANN.
Figure 1.9 Accuracy plot for three hidden layer–based ANN.
Figure 1.10 Accuracy plot for four hidden layer–based ANN.
Figure 1.11 Accuracy vs. hidden layer.
From the above-mentioned graphs, it is evident that the accuracy at hidden layer 4 is good. So, we will be predicting the difficult of code 8 with the ANN model with four hidden layers and Bernoulli’s RBM.
The ANN model predicts that the person feels the unknown code easy by a probability of 0.530607.
The Bernoulli RBM model predicts that the person feels the unknown code easy by a probability of 0.679071.
The data collected was quite less when compared to the data required for ANN. The less data accounts for the less accuracy generated by the model. We fail to consider higher number of hidden layers since there is a chance of over fitting. The main reason to generate the graphs of accuracy vs. epochs is to see how the accuracy is changing with respect to epochs. The main reason to generate the graph of accuracy vs. hidden layer is to see which number of hidden layers is best to make the prediction. This model can be very much helpful in the software industries to make predictions on how much stress a person would face if he is to do a coding part of a project. So, the company can give the required instruction to the employee and suggest some methods which can increase the skill level of the person which reduce the mental work load while he does the project and also improving the capacity of the employee. We can also use this in the universities to see how much stress a student faces when he tries to solve programming questions. Using this data, the university officials can tweak their teaching policies and try to improvise the skill level of the student and help in improving the new generation day by day.
1.7 Advantages and Disadvantages of the Study
The advantages of the study are multifold. First, it helps academicians and industry professionals to understand a novel process of mental workload prediction and analysis. Second, it contributes in the application of deep learning in mental work load prediction. Third, based on the authors’ knowledge, this is first which provides an application of deep learning in prediction of mental workload during code debugging. The disadvantage of the study can be attributed to the use of small data set for the prediction of mental workload.
1.8 Limitations of the Study
The analysis related to this study has been conducted based on the small dataset of eye movements collected during the code debugging. As the dataset is small, the results which have been obtained out of the analysis may be misleading in terms of prediction and accuracy.
In this paper, a deep learning has been proposed for the prediction of mental workload based on eye movements’ data. First, the data set is collected based on the eye movements during code debugging. Second, the raw eye movement data has been pre-processed and features are extracted for the further analysis. Third, the analysis has been conducted based on the deep learning models. As we can see that the accuracy of the model is quite low, so in the future works, we would try to make a new dataset with more data which can be easily fed to any deep learning network and also work on tuning some parameters of the artificial neural networks which help in increasing the accuracy of the model. The work load prediction which we obtained from the deep learning tool shows that the 8th code which was of unknown difficulty is easy to the person debugging the code. But the probability is slightly >0.5 so doing with more other codes will help in tuning our model and help to increase the accuracy of the predictions.
1. Sweller, J., Van Merrienboer, J.J.G., Paas, F.G., Cognitive architecture and instructional design. Educ. Psychol. Rev ., 10, 3, 251–296, 1998.
Читать дальше