There was a human-like feature to this that I found somewhat unsettling: I was watching an AI that had a goal and learned to get ever better at achieving it, eventually outperforming its creators. In the previous chapter, we defined intelligence as simply the ability to accomplish complex goals, so in this sense, DeepMind’s AI was growing more intelligent in front of my eyes (albeit merely in the very narrow sense of playing this particular game). In the first chapter, we encountered what computer scientists call intelligent agents: entities that collect information about their environment from sensors and then process this information to decide how to act back on their environment. Although DeepMind’s game-playing AI lived in an extremely simple virtual world composed of bricks, paddles and balls, I couldn’t deny that it was an intelligent agent.
DeepMind soon published their method and shared their code, explaining that it used a very simple yet powerful idea called deep reinforcement learning .2 Basic reinforcement learning is a classic machine learning technique inspired by behaviorist psychology, where getting a positive reward increases your tendency to do something again and vice versa. Just like a dog learns to do tricks when this increases the likelihood of its getting encouragement or a snack from its owner soon, DeepMind’s AI learned to move the paddle to catch the ball because this increased the likelihood of its getting more points soon. DeepMind combined this idea with deep learning: they trained a deep neural net, as in the previous chapter, to predict how many points would on average be gained by pressing each of the allowed keys on the keyboard, and then the AI selected whatever key the neural net rated as most promising given the current state of the game.
When I listed traits contributing to my own personal feeling of self-worth as a human, I included the ability to tackle a broad range of unsolved problems. In contrast, being able to play Breakout and do nothing else constitutes extremely narrow intelligence. To me, the true importance of DeepMind’s breakthrough is that deep reinforcement learning is a completely general technique. Sure enough, they let the exact same AI practice playing forty-nine different Atari games, and it learned to outplay their human testers on twenty-nine of them, from Pong to Boxing, Video Pinball and Space Invaders.
It didn’t take long until the same AI idea had started proving itself on more modern games whose worlds were three-dimensional rather than two-dimensional. Soon DeepMind’s San Francisco–based competitors at OpenAI released a platform called Universe, where DeepMind’s AI and other intelligent agents can practice interacting with an entire computer as if it were a game: clicking on anything, typing anything, and opening and running whatever software they’re able to navigate—firing up a web browser and messing around online, for example.
Looking to the future of deep reinforcement learning and improvements thereupon, there’s no obvious end in sight. The potential isn’t limited to virtual game worlds, since if you’re a robot, life itself can be viewed as a game. Stuart Russell told me that his first major HS moment was watching the robot Big Dog run up a snow-covered forest slope, elegantly solving the legged locomotion problem that he himself had struggled to solve for many years.3 Yet when that milestone was reached in 2008, it involved huge amounts of work by clever programmers. After DeepMind’s breakthrough, there’s no reason why a robot can’t ultimately use some variant of deep reinforcement learning to teach itself to walk without help from human programmers: all that’s needed is a system that gives it points whenever it makes progress. Robots in the real world similarly have the potential to learn to swim, fly, play ping-pong, fight and perform a nearly endless list of other motor tasks without help from human programmers. To speed things up and reduce the risk of getting stuck or damaging themselves during the learning process, they would probably do the first stages of their learning in virtual reality.
Intuition, Creativity and Strategy
Another defining moment for me was when the DeepMind AI system AlphaGo won a five-game Go match against Lee Sedol, generally considered the top player in the world in the early twenty-first century.
It was widely expected that human Go players would be dethroned by machines at some point, since it had happened to their chess-playing colleagues two decades earlier. However, most Go pundits predicted that it would take another decade, so AlphaGo’s triumph was a pivotal moment for them as well as for me. Nick Bostrom and Ray Kurzweil have both emphasized how hard it can be to see AI breakthroughs coming, which is evident from interviews with Lee Sedol himself before and after losing the first three games:
• October 2015: “Based on its level seen…I think I will win the game by a near landslide.”
• February 2016: “I have heard that Google DeepMind’s AI is surprisingly strong and getting stronger, but I am confident that I can win at least this time.”
• March 9, 2016: “I was very surprised because I didn’t think I would lose.”
• March 10, 2016: “I’m quite speechless…I am in shock. I can admit that…the third game is not going to be easy for me.”
• March 12, 2016: “I kind of felt powerless.”
Within a year after playing Lee Sedol, a further improved AlphaGo had played all twenty top players in the world without losing a single match.
Why was this such a big deal for me personally? Well, I confessed above that I view intuition and creativity as two of my core human traits, and as I’ll now explain, I feel that AlphaGo displayed both.
Go players take turns placing black and white stones on a 19-by-19 board (see figure 3.2). There are vastly more possible Go positions than there are atoms in our Universe, which means that trying to analyze all interesting sequences of future moves rapidly gets hopeless. Players therefore rely heavily on subconscious intuition to complement their conscious reasoning, with experts developing an almost uncanny feel for which positions are strong and which are weak. As we saw in the last chapter, the results of deep learning are sometimes reminiscent of intuition: a deep neural network might determine that an image portrays a cat without being able to explain why. The DeepMind team therefore gambled on the idea that deep learning might be able to recognize not merely cats, but also strong Go positions. The core idea that they built into AlphaGo was to marry the intuitive power of deep learning with the logical power of GOFAI—which stands for what’s humorously known as “Good Old-Fashioned AI” from before the deep-learning revolution. They used a massive database of Go positions from both human play and games where AlphaGo had played a clone of itself, and trained a deep neural network to predict from each position the probability that white would ultimately win. They also trained a separate network to predict likely next moves. They then combined these networks with a GOFAI method that cleverly searched through a pruned list of likely future-move sequences to identify the next move that would lead to the strongest position down the road.
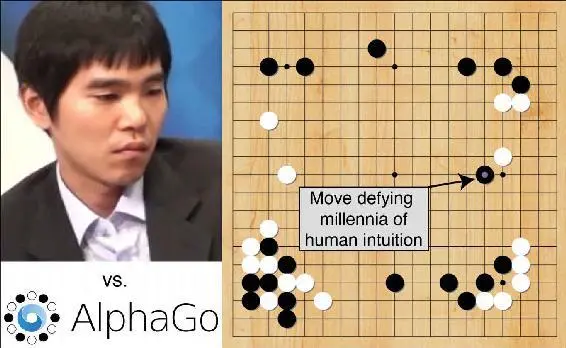
Figure 3.2: DeepMind’s AlphaGo AI made a highly creative move on line 5, in defiance of millennia of human wisdom, which about fifty moves later proved crucial to its defeat of Go legend Lee Sedol.
This marriage of intuition and logic gave birth to moves that were not merely powerful, but in some cases also highly creative. For example, millennia of Go wisdom dictate that early in the game, it’s best to play on the third or fourth line from an edge. There’s a trade-off between the two: playing on the third line helps with short-term territory gain toward the side of the board, while playing on the fourth helps with long-term strategic influence toward the center.
Читать дальше