AI for Finance
Finance is another area that’s been transformed by information technology, allowing resources to be efficiently reallocated across the globe at the speed of light and enabling affordable financing for everything from mortgages to startup companies. Progress in AI is likely to offer great future profit opportunities from financial trading: most stock market buy/sell decisions are now made automatically by computers, and my graduating MIT students routinely get tempted by astronomical starting salaries to improve algorithmic trading.
Verification is important for financial software as well, which the American firm Knight Capital learned the hard way on August 1, 2012, by losing $440 million in forty-five minutes after deploying unverified trading software.13 The trillion-dollar “Flash Crash” of May 6, 2010, was noteworthy for a different reason. Although it caused massive disruptions for about half an hour before markets stabilized, with shares of some prominent companies such Procter & Gamble swinging in price between a penny and $100,000,14 the problem wasn’t caused by bugs or computer malfunctions that verification could have avoided. Instead, it was caused by expectations being violated: automatic trading programs from many companies found themselves operating in an unexpected situation where their assumptions weren’t valid—for example, the assumption that if a stock exchange computer reported that a stock had a price of one cent, then that stock really was worth one cent.
The flash crash illustrates the importance of what computer scientists call validation: whereas verification asks “Did I build the system right?,” validation asks “Did I build the right system?” *2For example, does the system rely on assumptions that might not always be valid? If so, how can it be improved to better handle uncertainty?
AI for Manufacturing
Needless to say, AI holds great potential for improving manufacturing, by controlling robots that enhance both efficiency and precision. Ever-improving 3-D printers can now make prototypes of anything from office buildings to micromechanical devices smaller than a salt grain.15 While huge industrial robots build cars and airplanes, affordable computer-controlled mills, lathes, cutters and the like are powering not merely factories, but also the grassroots “maker movement,” where local enthusiasts materialize their ideas at over a thousand community-run “fab labs” around the world.16 But the more robots we have around us, the more important it becomes that we verify and validate their software. The first person known to have been killed by a robot was Robert Williams, a worker at a Ford plant in Flat Rock, Michigan. In 1979, a robot that was supposed to retrieve parts from a storage area malfunctioned, and he climbed into the area to get the parts himself. The robot silently began operating and smashed his head, continuing for thirty minutes until his co-workers discovered what had happened.17 The next robot victim was Kenji Urada, a maintenance engineer at a Kawasaki plant in Akashi, Japan. While working on a broken robot in 1981, he accidentally hit its on switch and was crushed to death by the robot’s hydraulic arm.18 In 2015, a twenty-two-year-old contractor at one of Volkswagen’s production plants in Baunatal, Germany, was working on setting up a robot to grab auto parts and manipulate them. Something went wrong, causing the robot to grab him and crush him to death against a metal plate.19
Although these accidents are tragic, it’s important to note that they make up a minuscule fraction of all industrial accidents. Moreover, industrial accidents have decreased rather than increased as technology has improved, dropping from about 14,000 deaths in 1970 to 4,821 in 2014 in the United States.20 The three above-mentioned accidents show that adding intelligence to otherwise dumb machines should be able to further improve industrial safety, by having robots learn to be more careful around people. All three accidents could have been avoided with better validation: the robots caused harm not because of bugs or malice, but because they made invalid assumptions—that the person wasn’t present or that the person was an auto part.
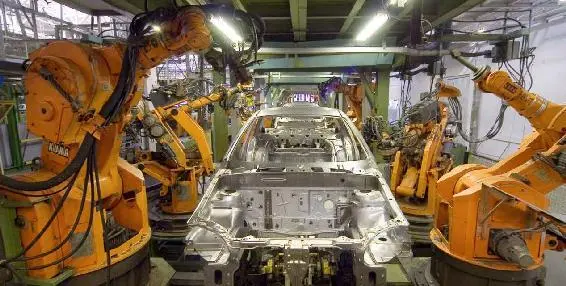
Figure 3.3: Whereas traditional industrial robots are expensive and hard to program, there’s a trend toward cheaper AI-powered ones that can learn what to do from workers with no programming experience.
AI for Transportation
Although AI can save many lives in manufacturing, it can potentially save even more in transportation. Car accidents alone took over 1.2 million lives in 2015, and aircraft, train and boat accidents together killed thousands more. In the United States, with its high safety standards, motor vehicle accidents killed about 35,000 people last year—seven times more than all industrial accidents combined.21 When we had a panel discussion about this in Austin, Texas, at the 2016 annual meeting of the Association for the Advancement of Artificial Intelligence, the Israeli computer scientist Moshe Vardi got quite emotional about it and argued that not only could AI reduce road fatalities, but it must: “It’s a moral imperative!” he exclaimed. Because almost all car crashes are caused by human error, it’s widely believed that AI-powered self-driving cars can eliminate at least 90% of road deaths, and this optimism is fueling great progress toward actually getting self-driving cars out on the roads. Elon Musk envisions that future self-driving cars will not only be safer, but will also earn money for their owners while they’re not needed, by competing with Uber and Lyft.
So far, self-driving cars do indeed have a better safety record than human drivers, and the accidents that have occurred underscore the importance and difficulty of validation. The first fender bender caused by a Google self-driving car took place on February 14, 2016, because it made an incorrect assumption about a bus: that its driver would yield when the car pulled out in front of it. The first lethal crash caused by a self-driving Tesla, which rammed into the trailer of a truck crossing the highway on May 7, 2016, was caused by two bad assumptions:22 that the bright white side of the trailer was merely part of the bright sky, and that the driver (who was allegedly watching a Harry Potter movie) was paying attention and would intervene if something went wrong. *3
But sometimes good verification and validation aren’t enough to avoid accidents, because we also need good control: ability for a human operator to monitor the system and change its behavior if necessary. For such human-in-the-loop systems to work well, it’s crucial that the human-machine communication be effective. In this spirit, a red light on your dashboard will conveniently alert you if you accidentally leave the trunk of your car open. In contrast, when the British car ferry Herald of Free Enterprise left the harbor of Zeebrugge on March 6, 1987, with her bow doors open, there was no warning light or other visible warning for the captain, and the ferry capsized soon after leaving the harbor, killing 193 people.23
Another tragic control failure that might have been avoided by better machine-human communication occurred during the night of June 1, 2009, when Air France Flight 447 crashed into the Atlantic Ocean, killing all 228 on board. According to the official accident report, “the crew never understood that they were stalling and consequently never applied a recovery manoeuvre”—which would have involved pushing down the nose of the aircraft—until it was too late. Flight safety experts speculated that the crash might have been avoided had there been an “angle-of-attack” indicator in the cockpit, showing the pilots that the nose was pointed too far upward.24
Читать дальше