Just as most digital computers gain efficiency by splitting their work into multiple steps and reusing computational modules many times, so do many artificial and biological neural networks. Brains have parts that are what computer scientists call recurrent rather than feedforward neural networks, where information can flow in multiple directions rather than just one way, so that the current output can become input to what happens next. The network of logic gates in the microprocessor of a laptop is also recurrent in this sense: it keeps reusing its past information, and lets new information input from a keyboard, trackpad, camera, etc., affect its ongoing computation, which in turn determines information output to, say, a screen, loudspeaker, printer or wireless network. Analogously, the network of neurons in your brain is recurrent, letting information input from your eyes, ears and other senses affect its ongoing computation, which in turn determines information output to your muscles.
The history of learning is at least as long as the history of life itself, since every self-reproducing organism performs interesting copying and processing of information—behavior that has somehow been learned. During the era of Life 1.0, however, organisms didn’t learn during their lifetime: their rules for processing information and reacting were determined by their inherited DNA, so the only learning occurred slowly at the species level, through Darwinian evolution across generations.
About half a billion years ago, certain gene lines here on Earth discovered a way to make animals containing neural networks, able to learn behaviors from experiences during life. Life 2.0 had arrived, and because of its ability to learn dramatically faster and outsmart the competition, it spread like wildfire across the globe. As we explored in chapter 1, life has gotten progressively better at learning, and at an ever-increasing rate. A particular ape-like species grew a brain so adept at acquiring knowledge that it learned how to use tools, make fire, speak a language and create a complex global society. This society can itself be viewed as a system that remembers, computes and learns, all at an accelerating pace as one invention enables the next: writing, the printing press, modern science, computers, the internet and so on. What will future historians put next on that list of enabling inventions? My guess is artificial intelligence.
As we all know, the explosive improvements in computer memory and computational power (figure 2.4 and figure 2.8) have translated into spectacular progress in artificial intelligence—but it took a long time until machine learning came of age. When IBM’s Deep Blue computer overpowered chess champion Garry Kasparov in 1997, its major advantages lay in memory and computation, not in learning. Its computational intelligence had been created by a team of humans, and the key reason that Deep Blue could outplay its creators was its ability to compute faster and thereby analyze more potential positions. When IBM’s Watson computer dethroned the human world champion in the quiz show Jeopardy! , it too relied less on learning than on custom-programmed skills and superior memory and speed. The same can be said of most early breakthroughs in robotics, from legged locomotion to self-driving cars and self-landing rockets.
In contrast, the driving force behind many of the most recent AI breakthroughs has been machine learning . Consider figure 2.11, for example. It’s easy for you to tell what it’s a photo of, but to program a function that inputs nothing but the colors of all the pixels of an image and outputs an accurate caption such as “A group of young people playing a game of frisbee” had eluded all the world’s AI researchers for decades. Yet a team at Google led by Ilya Sutskever did precisely that in 2014. Input a different set of pixel colors, and it replies “A herd of elephants walking across a dry grass field,” again correctly. How did they do it? Deep Blue–style, by programming handcrafted algorithms for detecting frisbees, faces and the like? No, by creating a relatively simple neural network with no knowledge whatsoever about the physical world or its contents, and then letting it learn by exposing it to massive amounts of data. AI visionary Jeff Hawkins wrote in 2004 that “no computer can…see as well as a mouse,” but those days are now long gone.
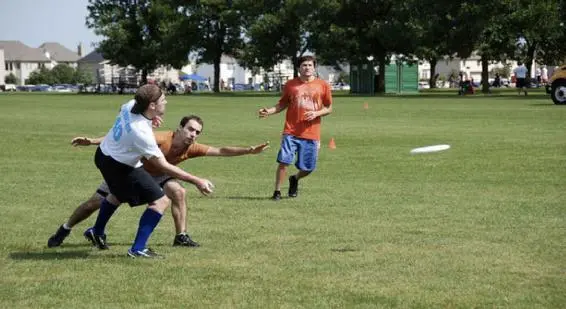
Figure 2.11: “A group of young people playing a game of frisbee”—that caption was written by a computer with no understanding of people, games or frisbees.
Just as we don’t fully understand how our children learn, we still don’t fully understand how such neural networks learn, and why they occasionally fail. But what’s clear is that they’re already highly useful and are triggering a surge of investments in deep learning. Deep learning has now transformed many aspects of computer vision, from handwriting transcription to real-time video analysis for self-driving cars. It has similarly revolutionized the ability of computers to transform spoken language into text and translate it into other languages, even in real time—which is why we can now talk to personal digital assistants such as Siri, Google Now and Cortana. Those annoying CAPTCHA puzzles, where we need to convince a website that we’re human, are getting ever more difficult in order to keep ahead of what machine-learning technology can do. In 2015, Google DeepMind released an AI system using deep learning that was able to master dozens of computer games like a kid would—with no instructions whatsoever—except that it soon learned to play better than any human. In 2016, the same company built AlphaGo, a Go-playing computer system that used deep learning to evaluate the strength of different board positions and defeated the world’s strongest Go champion. This progress is fueling a virtuous circle, bringing ever more funding and talent into AI research, which generates further progress.
We’ve spent this chapter exploring the nature of intelligence and its development up until now. How long will it take until machines can out-compete us at all cognitive tasks? We clearly don’t know, and need to be open to the possibility that the answer may be “never.” However, a basic message of this chapter is that we also need to consider the possibility that it will happen, perhaps even in our lifetime. After all, matter can be arranged so that when it obeys the laws of physics, it remembers, computes and learns—and the matter doesn’t need to be biological. AI researchers have often been accused of over-promising and under-delivering, but in fairness, some of their critics don’t have the best track record either. Some keep moving the goalposts, effectively defining intelligence as that which computers still can’t do, or as that which impresses us. Machines are now good or excellent at arithmetic, chess, mathematical theorem proving, stock picking, image captioning, driving, arcade game playing, Go, speech synthesis, speech transcription, translation and cancer diagnosis, but some critics will scornfully scoff “Sure—but that’s not real intelligence!” They might go on to argue that real intelligence involves only the mountaintops in Moravec’s landscape (figure 2.2) that haven’t yet been submerged, just as some people in the past used to argue that image captioning and Go should count—while the water kept rising.
Assuming that the water will keep rising for at least a while longer, AI’s impact on society will keep growing. Long before AI reaches human level across all tasks, it will give us fascinating opportunities and challenges involving issues such as bugs, laws, weapons and jobs. What are they and how can we best prepare for them? Let’s explore this in the next chapter.
Читать дальше