1 ...7 8 9 11 12 13 ...16 Comprehensive Learning Particle Swarm Optimization (CLPSO)
Dragonfly Algorithm (DA)
Multi-objective particle swarm optimization (MOPSO).
The best cluster head is one who increases network efficiency and network lifetime. Selection can be performed based on defined parameters. To find the optimum solution we consider, the current fitness value of each node in comparison with the new fitness value, if the current value is better than the previous one, the old value is replaced by a new one, otherwise stays same. Figure 3.4is presenting the flow of proposed scheme. Table 3.1is describing defined simulation parameters.
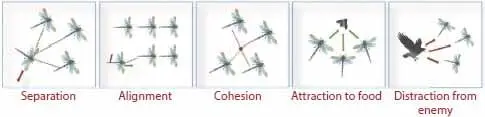
Figure 3.4 Primitive corrective patterns between dragonfly.
Table 3.1 Simulation parameters.
Parameters |
Values |
Population size |
100 |
Maximum iterations |
150 |
Lower bound (lb) |
0 |
Upper bound (ub) |
100 |
Dimensions |
2 |
Transmission range (m) |
2, 4, 6, 8, 10 |
Nodes |
50, 100, 150, 200, 250, 300 |
Mobility model |
Freely mobility model |
W1 |
0.5 |
W2 |
0.5 |
3.4.1 Dragonfly Algorithm
The main objective of the dragonfly is the survival. So they need to be attracted towards the food and distracted form the enemies [18]. Five main factors for position updating are shown in the Figure 3.4. Alignment formula is shown in Equation (3.1)[18].
[3.1] 
Table 3.2: Proposed Technique. Here Vj is the velocity of the j-th neighbor. Cohesion calculation formula [18].
Table 3.2 Dragonfly Algorithm.
1) Initialization of WBAN’s randomly in the network 2) Random direction of WBAN’s is defined 3) Speed and velocity of each WBAN is initialized 4) Mesh topology creation among nodes 5) For all Dragonflies same radius is initialized 6) Calculation of distance among all WBANs, normalizing and associating the distance values with corresponding nodes 7) For (iteration = 1) 1 to 10 8) Available nodes for cluster formation = All Node a) WHILE (Nodes Available for clustering! = empty) b) End while 9) FOR Drag-fly(i) = form 1 to Total population a) Source (Food/enemy) = empty, Food Source Cost = infinity, and Enemy Source cost = -infinity b) Objective Values calculation, of Dragonflies c) Update the radius d) Update sources (food and enemy) e) Update weights 10) END FOR 11) FOR Drag-fly(i) = From 1 to Total population a) FOR Drag-fly(i) = 1 to Total population i) Update neighboring radius b) ENDFOR c) Calculate Separation, Alignment, Cohesion, Enemy and Food weights 12) IF neighbor! =0 a) Velocity Update b) Position Update 13) Else a) Levy flight 14) END IF 15) END FOR 16) Best cost == Food fitness 17) END FOR 18) IF not clustered nodes>20% a) Goto line #1 19) Else a) Output 20) End IF |
[3.2] 
Attrition for food calculated as [18]:
[3.3] 
Here X+ is position of food source. And X is current position of an individual.
Distraction away from an enemy [18]:
[3.4] 
X—is position of an enemy.
3.5 Result and Simulations
The experimentation is being performed on the grid size of 1 km × 1 km. The transmission range of each node is varying from 2m, 4m, 6m, 8m, and 10m. To find the number of clusters we kept the number of nodes static against each transmission range. Number of nodes varies from 50, 100, 150, 200, 250, and 300. The proposed algorithm finds an optimized solution against each transmission range, which is presented in graphs below. One thing is commonly observed in all solutions less transmission range produce high number of clusters, it is because, with low transmission range, nodes have less coverage area, only a few other nodes are in the vicinity. So, with less transmission range number or clusters is increased, with a low number of CMs. In the comparison of CLPSO, DA, and MOPSO, we see that Figure 3.5A is describing the cluster formation for the number of nodes 50. On minimum transmission range 2, no clusters are formed by all three algorithms. But with an increase in transmission range, cluster formation tends to start. In Figure 3.5A, on transmission range 4 CLPSO is giving the worst result with a maximum number of cluster heads. On transmission range 6 MOPSO is worst and on Transmission range 8 again CLPSO is forming the maximum number of clusters. The variation between CLPSO and MOPSO can be seen in Figure 3.5A. DA is giving the best results with a minimum number of cluster heads in all Transmission ranges. By looking at all graphs (A,B,C,D) of Figure 3.5it can be seen DA is giving the best results with a minimum number of cluster heads. There is a slight variation between the results of CLPSO and MOPSO.
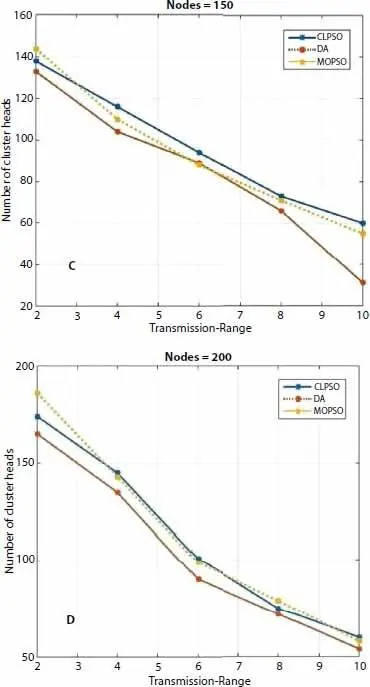
Figure 3.5 Transmission range versus Cluster Heads for Nodes 50–200.
Figure 3.6is showing the results for nodes 250 and 300. In both Figure labels A and B it can be seen that DA with a higher number of nodes is giving remarkable efficient results. On starting transmission ranges of 2, 4, and 6 DA’s performance is extremely different than CLPSO and MPSO.
Figure 3.6 Transmission range versus Cluster Heads for Nodes 250 and 300.
As transmission range increases to 8 and 10 MPSO is also trying to meet DA. But if we analyze overall results DA stands alone as the best participant. That formed a smaller number of clusters in almost all number of nodes and transmission ranges.
Wireless Body Area Network (WBAN) protects the patient’s life by its continuous monitoring and data transmission mechanism. For load balancing the most important method in WBAN is clustering which provides practical approach for energy optimization of senor nodes. We designed a cluster formation technique using Evolutionary algorithms. Optimized clustering is grouping the nodes of the network in the most efficient way. We also need a minimum number of possible clusters, long-lasting in the network. We analyzed the performance difference in Comprehensive Learning Particle Swarm Optimization (CLPSO), Dragonfly Algorithm (DA), and Multi-objective particle swarm optimization (MOPSO). Our experimentation has shown that the overall performance of DA is the most efficient among all three algorithms, as it forms fewest optimized long lasting clusters.
Читать дальше