SIR model is one of the simplest diffusion models. It has three states where S stands for number of susceptible, I for number of infectious, and R for number of recovered or removed. Total number of people is considered collectively from these three states susceptible, infected, and recovered [15].
Figure 1.9 SIS model.
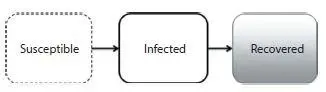
Figure 1.10 SIR model.
In social networks, once rumor is diffused and received by any user he/she becomes infectious if doesn’t know truth about rumor. If they knew truth, he/she recover by ignoring rumor or not passing to neighbors. This is ignored in SI and SIS models. Recovery from rumors is only between SIR and SIS models. Figure 1.10shows how users are transforming from one state to other.
In SIR model once a person recovered from disease he/she remains in same state in future. In general once a person is cured from any disease there is chance that they may be reinfected with same decease in future, which is ignored in SIR model. SIRS model addresses this problem where once a person is infected and have recovered by having immunity or medical treatment, they couldn’t be in same recovered state in future. After recovery, there is possibility that again infected by same decease [16].
In social networks, once a rumor is diffused and received by any user, if believed, user is infected; otherwise if fact is known about rumor, user recovers by ignoring or not passing to neighbors. There is possibility that this recovered node again will be reinfected in the future on social networks. For further details see Figure 1.11.
All these diffusion models are explained in Ref. [41]. There are independent cascade models to find rumor sources by analyzing network diffusion in reverse direction [42].
Figure 1.11 SIRS model.
1.5.3 Centrality Measures
In rumor source identification centrality measures are also considered as one of the important factors. Centrality measures are computed to assign a score to each node, which influences the diffusion process [43]. There are several centrality measures discussed in Ref. [17], such as Degree centrality, Closeness centrality, and Betweenness centrality and are explained in following sections.
1.5.3.1 Degree Centrality
It is defined as the total nodes connected to a node in network or graph. Currently, eminent people like politicians, actors, and sports players are having excellent degrees of centrality with others in the network [40]. Social networks like Facebook and Twitter are proving this as many famous people having more number of friends and followers in their accounts. Figure 1.12(a) illustrates this degree of centrality measures.
1.5.3.2 Closeness Centrality
It is defined as smallest distance among a node and other nodes in the graph [46]. See Figure 1.12(b) for more details, where closeness centrality is shown as node with black color and having the same distance with all other nodes in graph.
Figure 1.12 Centrality measures.
1.5.3.3 Betweenness Centrality
It is defined as a node i.e. bridge between any other two nodes and has the shortest path between them among it. It is observed that a node with better betweenness centrality may not have better degree which is necessary in information diffusion [47]. Figure 1.12(c) depicts how a betweenness centrality chosen, node in black color acts as a bridge between others. For more details about rumor centrality measures see Refs. [44, 45].
1.6 Source Detection in Network
Rumor source identification in social networks is an emerging topic, introduced by Ref. [9]. In order to find the rumor source in network, first task is to classify given data rumor or not, then apply source detection techniques on network which contain nodes that are infected by rumors. The following Figure 1.13will give idea about how a rumor is classified from given data using classification algorithms and how rumor sources are classified based on metrics. Initially, it is needed to collect dataset of sender and receiver messages (rumor) from social network, do data processing like removing urls, hashttags, stopwords, etc. and annotation. Next construct propagation graph finds rumor or misinformation, and selects any suitable diffusion model to get information about how a rumor is diffused in network, suitable metrics for source detection and evaluation. It also classifies sources based on metrics available, do validation and analysis of results.
Source detection approaches are classified into two most important categories: single source detection and multiple source detection [10].
Figure 1.13 Rumor source detection process.
1.6.1 Single Source Detection
This section explains detection of single source of rumor in social networks. Major research work has been performed on single source detection and proposed many techniques. All these techniques have been classified again as anti-rumor based, query based, and network observation, etc.
1.6.1.1 Network Observation
Network observation is discussed in Section 1.5.1.2. The three types of observation techniques are complete, snapshot, and monitor observations which are very useful in source identification. The three methods and how they used in detection of rumor source are explained in following sections.
1.6.1.1.1 Complete Observation
First, Rumor source identification in networks was proposed in Ref. [9], to find source in network, consider a tree-like network as structure of network is one of the factors to detect source. The authors assume a node receives information from its neighbor nodes in trees. SIR model considered to find how this information is diffusion occurs from one node to other. Next factor to be considered is centrality measures, and gives knowledge about rumor centrality of one node, it is defined as a number of links from source node. If any node is having better rumor centrality it is considered as source of rumor diffusion. For more details see Ref. [45]. Rumor source estimator and maximum likelihood estimators are explained in following section.
1 A. Rumor Source EstimatorTo find rumor source estimator first need to know how rumor spreading over network? Rumor spreading model gives solution to this problem. There are many models like SI, SIS, SIR and SIRS uses as diffusion models to spread rumor over the network. These models are applied to diffuse information in online social networks. In this section a simple example is discussed on how a rumor can spread over the network.Let’s consider an undirected graph G (V, E), where V is a countable infinite set of nodes, and E is the set of form (i, j) for some i and j in V, and consider the case where initially only one node ν* is the rumor source. For the rumor spreading model, use popular model subject to infected or SI representation which does not permit for any nodes to get well, i.e. once a node with the rumor, it remains such forever. Once a node i with the rumor, it is able to widen it to another node j if and only if there is an edge among them, i.e. if (i, j) ∈ E. A node i needs the time to widen the rumor to node j is modeled by an exponential random variable τij has the rate λ. Suppose there is no loss of generality that λ = 0. All τij’s are autonomous and identically distributed [9].
Читать дальше