Where
P(c/x) is the posterior probability of class.
P(c) is the prior probability of class.
P(x/c) is the likelihood which is the probability of given class.
P(x) is the prior probability of predictor.
Naive Bayes classifier is a combination of Bayes theorem and Naïve assumptions. This algorithm calculates assumption values even though use multiple parameters as input. Rumor detection is purely based on either classification of text or images. For example, try rumor detection in social networks like Twitter or Facebook, then it is required to consider several features like User features, Tweet features, and Comment features. All these features deal with text data [32]. If Tweet or post or comment includes these features then one can apply Naïve Bayes classifier algorithm to classify them whether it is a rumor or not. These features are classified into three categories. Some of dataset features are listed in Table 1.2.
First, consider user features, number of followers or friends to a particular person are more, then it may be considered as truth, otherwise it is a rumor. Because, in survey it is observed that many people who share rumors may have less number of followers or friends in their social networking accounts. Second, there are many features to be considered as Tweet features from which one can detect whether it is rumor or not. For example, consider number of retweets, number of words or number of characters. If count of any one of these or all of these are more than average range in size, then the tweet may be rumor, otherwise it is truth. Third one is comment features. These are very much important features used in rumor detection. This feature is based on comments given by many people who are already infected by the particular post or tweet. If found comments like Is it real? Impossible? How it is possible? Or I can’t believe this, then the particular post/tweet may be a rumor. There have many other features to distinguish whether a post/tweet is rumor or not. Figure 1.4below gives a brief idea about how Naïve Bayes algorithm classifies different classes of data points.
Figure 1.4 Naïve Bayes classifier.
It can be observed that there are two classes of data points and how they are classified with maximum distance.
Two classes are
1 i. Circle
2 ii. Triangle.
Adding more parameters in input dataset reduces the accuracy when compared to using less parameter. To increase the accuracy use another popular model SVM.
1.4.1.2 Support Vector Machine
Support vector machine (SVM) is a one of the best machine learning algorithm used for both classification and regression, widely it is used for classify given data points even though those input vectors are mapped non-linearly [8]. In social networks data available in many forms so to detect rumors it is required to classify given text data using classification algorithms based on dataset features. Classifying dataset which has multiple features and multiple dimensions is a challenging task, so using SVM will give better results.
The main objective of the SVM is to find a hyperplane in an N-dimensional space that distinctly classifies the data points. Rumor detection in social networks is mainly depending on text classification, using SVM algorithm it can be done. It is shown in Figure 1.5, how a SVM classifier classifies the given dataset that has multiple features and dimensions. SVM classifies as large margin in between two types of data: first one is in circle shape and the second one is in triangle shape. These two data points have been classified with maximum distance (thick line) between them. The large margin shown in Figure 1.5(a) says that it is classifying those circles and triangles equally from that point, which means distance between those two data types is maximum through that margin. As shown in Figure 1.5(b), SVM also supports multi-dimensional data.
Figure 1.5 Hyperplane in 2-D and 3-D.
1.4.1.2.1 Cost Function and Gradient Features
SVM algorithm looks to maximize the margin between the data points and the hyper plane. The loss function that helps maximize the margin is hinge loss [8] and is defined as follows:
(1.2) 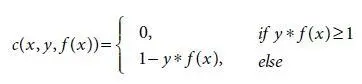
If predicted value and expected value have the same sign then the cost function is 0.
1.4.2 Combating Misinformation on Instagram
Classification of shared contents by users in social media is prevalent in combating misinformation. Baseline classification algorithms like Naïve Bayes theorem and SVM models have been used extensively for detecting rumor as discussed Section 1.4. Even though these algorithms classify rumors and facts in some manner, still there is a need to come up with some excellent techniques which may improve efficiency in rumor classification. Nowadays, social networks like Facebook, WhatsApp, Instagram and Twitter are using good techniques, but still they failed to classify the rumors exactly.
One of the popular social network, Facebook, has started in Instagram application (in US) to detect whether given post contains fact-information or false-information through some third party called as fact-checkers [33]. These third-party-fact-checkers are located globally and find rate of fact and false about particular post. When something is wrong in any post immediately fact-checkers check ratio of fact or misinformation.
If any post contains more false ratio then immediately it labels as “False information” otherwise no. Now it is the user’s responsibility to view or not that particular post based on false ratio and fact ratio, about share to their friends, communities or not. Using third-party-fact-checkers, Instagram is trying to combat misinformation on social networks. Figure 1.6will give you brief idea about this method.
Figure 1.6 Combating misinformation in Instagram [33].
1.5 Factors to Detect Rumor Source
Rumor detection is not only a solution to prevent these cyber-crimes in social media, but finding source plays an important role to prevent further diffusion and punish the culprit. Initially, finding source of rumors in network discussed by Ref. [9]. Later, much research has been done and has introduced several factors which are to be considered in RS identification. There are mainly four factors considered namely, diffusion models, network structure, evaluation metrics, and centrality measures. Each factor has been explained in the following section with examples. After rumor detection, consider these factors and find rumor source using source detection methods in social networks are explained in Section 1.5.2.
Network structure can be derived from two parameters: network topology and network observation [9]. Network topology describes the structure of network either in tree or graph. Source identification is more complex in the graph topology than tree topology, as tree has exactly one root node and no loops are allowed, Graph doesn’t have any root node and loops are allowed in network. Network observation is the second type of network structure and it is useful to observe the network during rumor propagation to get the knowledge about states of nodes in particular time. Network can be observed possibly in following three ways [11]: Complete, Snapshot and Monitor.
Читать дальше