Unsupervised learning implies designing algorithms to use data that have no labeling to evaluate the behavior or structure being analyzed. The algorithm is the best techniques to work on its own to discover patterns and information that was previously undetected. Clustering, anomaly, detection, Neural Networks etc. are all the examples of unsupervised learning.
Clustering: The internal groupings in the products, such as the grouping of customers, are investigated through a clustering problem. Modeling approaches including centroid-based and hierarchical are typically organized through clustering techniques.
Association: The question of the association rule is used to classify laws that describe significant quantities of input data, such as individuals who purchase X products, who also purchase Y objects. Association research can be achieved by evaluating rules for repeated if/then statement inputs and utilizing help requirements and trust to distinguish associations between unconnected data in a relational database.
Semi-Supervised Learning: Semi-controlled instruction is between approaches regulated and unregulated. Information is a labeled and blank experimental combination. Such architectures are synthetic are intended to consider and counteract the weaknesses of the main groups.
Reinforcement Learning: To order to optimize the principle of accrual compensation, enhanced learning, an ML area influenced by behavioral science, is concerned with the way virtual agents are to work to an environment. RL algorithms are used to learn policy of control, particularly if no prior information exists and a large amount of training data are available.
1.10.5 Machine Learning Tasks in Smart Building Environment
The key ML activities that are applicable to SB will be identified. For the general description of ML activities in SBs and measures to incorporate ML in an SB setting the reader is alluded to in Figure 1.24.
Collecting and collecting data: A range of methods were used to collect data, each of varying resources, energy consumption and networking deals. Sensors and related artifacts in SBs simultaneously produce raw information and these devices can store or record the information on monitored components for a specified period of time.
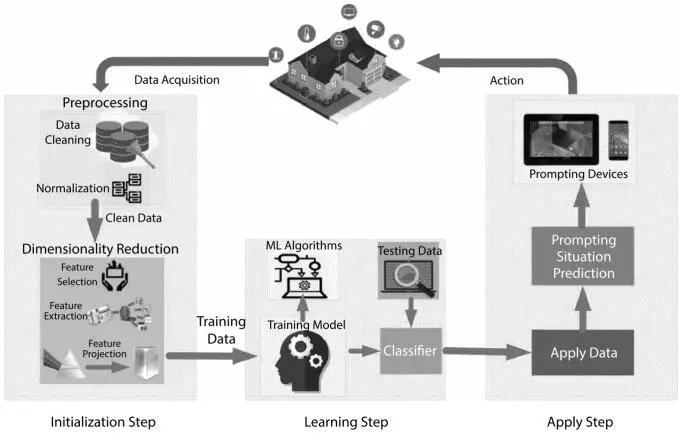
Figure 1.24ML tasks in SB Environment.
It can be utilized by decision-makers, planners, running and sustaining staff and building customers, many healthcare services and so on.
Data Pre-processing: Much data is generated in SBs by sensors from various sources with specific formats and architectures. The data come from different sources. This knowledge is not usually ready to be evaluated, since its poor battery capacity, bad tuning, access to numerous harmful elements and intervention may be incomplete or redundant.
Dimensionality Reduction: Raising volumes of raw data from heterogenous and all-embracing sensors used in SBs are enormous. The bulk of data from these sensors is redundant and needs to be minimized by utilizing techniques to limit their dimensionality to a smaller number of features without missing any valuable details.
1.10.6 ML Tools and Services for Smart Building
The learning method is facilitated across a number of ML frameworks and resources. The challenge of choosing the best platform in order to data analytics flow sharing can also be achieved from alternative viewpoints challenging despite the growing amount of such toolkits. There is generally no one toolkit that completely suits all challenges (Table 1.6) and includes remedies. Some of the toolkits available could overlap, with benefits and drawbacks.
Table 1.6Difference between deep learning and machine learning tools [56].
Tool |
Creator |
OS |
Open source? |
Written In |
Interface |
CUDA support? |
Algorithms |
Release date |
Tensor Flow |
Google Brain team |
Linux. Mac OS X (Windows support on road map |
Yes |
C++, Python |
Python, C/ C++ |
Yes |
Deep learning algorithm: RNN, CN, RBM and DUN. |
Novembeir 2015 |
Theano |
Universit de Montral |
Cross-platform |
Yes |
Python |
Python |
Yes |
Deep learning algorithm: RNN, CN, RBM and DBN. |
September 2007 |
H20 |
H20.ai |
Linux, Mac OS, Microsoft Windows And Cross-platform inch Apache HDFS; Amazon EC2, Google Compute Engine, and Microsoft Azure. |
Yes |
Java, Scala, Python, R |
Python, R |
No |
Algorithms for classification, clustering, generalized linear models, statistical analysis, ensembles, optimization tools, data pre-processing options and deep neural networks. |
August 2011 |
Deeplearning4j |
Various. Original author Adam Gibson |
Linux, OSX, Windows, Android, CyanogenMod (Cross-platform) |
Yes |
Java, Scala, C, CUDA |
Java, Scala, CIo-jure |
Yes |
Deep learning algorithms including: RBM, DBN. RNN. deep autoencoder |
August 2013 |
MLlib Spark |
Apache Software Foundation. UC Berkeley AMPLab, Databricks |
Microsoft Windows, OS X, Linux |
Yes |
Scala. Java, Python, R |
Scala, Java, Python. R |
No |
Classification, regression, clustering, dimensionality reduction, and collaborative filtering |
May 2014 |
Azure |
Dave Cutler from Microsoft |
Microsoft Windows, Linux |
No |
C++ |
C++, Java. ASP.NET, PHP. Nodejs, Python |
Yes |
Classification, regression, clustering |
October 2010 |
Torch |
Ronan Collobert, Koray Kavukcuoglu, Clement Farabet |
Linux. Android, Mac OS X TiOS |
Yes |
C, Lua |
Lua, LuaJlT. C, utility library for C++/ OpenCL |
Yes |
Deep algorithms |
October 2002 |
MOA |
University of Waikato |
Cross-platform |
Yes |
Java |
GUI, the commandline. and Java |
No |
ML algorithms (classification, regression, clustering, outlier detection, concept drift detection and recommender systems) |
November 2014 |
Caffe |
Berkeley Vision and Learning Center. community contributors |
Ubuntu, OS X, AWS, unofficial Android port, Windows support by Microsoft Research, unofficial Windows port |
Yes |
C++, Python |
C++, command line. Python, MATLAB |
Yes |
Deep learning algorithms: CN, and RNN |
December 2012 |
1.10.7 Big Data Research Applications for SBs in Real-Time
Many systems need a stream data processing in real time, so it is not feasible for this form of program to wait for data to be stored so evaluated. Stream processing is typically configured to interpret large volumes of data and operate on data streaming in real time by using constant queries, including SQL queries, to manage streaming data in real time, using an interface that is robust, accessible and fault resistant (Table 1.7).
1.10.8 Implementation of the ML Concept in the SB Context
Figure 1.25 illustrates specific measures to forecast an event in the SB sense by utilizing ML methods.
On the other hand, the aim of optimization is to optimize long-lasting gains by proper decisions. Strengthening learning with these issues can be used. Many optimization issues may be treated as predicting issues such that benefit is estimated for different activities and the activity with the largest income is chosen. The most important form in optimization is decision-making. A variety of factors and compromises about the effects of specific environmental locations need to be addressed.
Читать дальше