Table 1.10 Comparison of DenseNet.
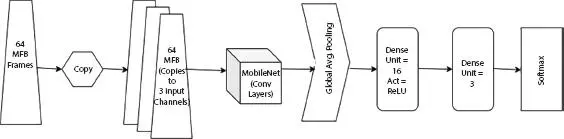
Figure 1.11 Architecture of MobileNets.
Table 1.11 Various parameters of MobileNets.
Type/Stride |
Filter shape |
Input size |
Conv / s2 |
3 × 3 × 3 × 32 |
224 × 224 × 3 |
Conv dw / s1 |
3 × 3 × 32 dw |
112 × 112 × 32 |
Conv / s1 |
1 × 1 × 32 × 64 |
112 × 112 × 32 |
Conv dw / s2 |
3 × 3 × 64 dw |
112 × 112 × 64 |
Conv / s1 |
1 × 1 × 64 × 128 |
56 × 56 × 64 |
Conv dw / s1 |
3 × 3 × 128 dw |
56 × 56 × 128 |
Conv / s1 |
1 × 1 × 128 × 128 |
56 × 56 × 128 |
Conv dw / s2 |
3 × 3 × 128 dw |
56 × 56 × 128 |
Conv / s1 |
1 × 1 × 1 × 128 × 256 |
28 × 28 × 128 |
Conv dw / s1 |
3 × 3 × 256 dw |
28 × 28 × 256 |
Conv / s1 |
1 × 1 × 256 × 256 |
28 × 28 × 256 |
Conv dw / s2 |
3 × 3 × 256 dw |
28 × 28 × 256 |
Conv / s1 |
1 × 1 × 256 × 512 |
14 × 14 × 256 |
5 × Conv dw / s1 Conv / s1 |
3 × 3 × 512 dw |
14 × 14 × 512 |
1 × 1 × 512 × 512 |
14 × 14 × 512 |
Conv dw / s2 |
3 × 3 × 512 dw |
14 × 14 × 512 |
Conv / s1 |
1 × 1 × 512 × 1024 |
7 × 7 × 512 |
Conv dw / s2 |
3 × 3 × 1,024 dw |
7 × 7 × 1,024 |
Conv / s1 |
1 × 1 × 1,024 × 1024 |
7 × 7 × 1,024 |
Avg Pool / s1 |
Pool 7 × 7 |
7 × 7 × 1,024 |
FC / s1 |
1024 × 1,000 |
1 × 1 × 1,024 |
Softmax / s1 |
Classifier |
1 × 1 × 1,000 |
Table 1.12 State-of-art of spine segmentation approaches.
Author |
Method/Algorithm |
Parameters |
Mader [11] |
V-Net |
MDSC (%) = 89.4MASD (mm) = 0.45 |
Bateson [12] |
Constrained domain adaptation employ ENet |
MDSC (%) = 81.1HD (mm) = 1.09 |
Zeng [13] |
CNN |
MDSC (%)= 90.64MASD (mm) = 0.60 |
Chang Liu [14] |
2.5D multi-scale FCN |
MDSC (%) = 90.64MASD (mm) = 0.60MLD (mm) = 0.77 |
Gao [15] |
2D CNN, DenseNet |
MDSC (%) = 90.58MASD (mm) = 0.61MLD (mm) = 0.78 |
Jose [17] |
HD-UNet asym |
MDSC (%) = 89.67MASD (mm) = 0.65MLD (mm) = 0.964 |
Claudia Iriondo [16] |
VNet-based 3D connected component analysis |
MDSC (%) = 89.71MASD (mm) = 0.74MLD (mm) = 0.86 |
1. LeCun, Y., Bottou, L., Bengio, Y., Haffner, P., Gradient-based learning applied to document recognition. Proc. IEEE , 86, 11, 2278–2323, 1998.
2. Krizhevsky, A., Sutskever, I., Hinton, G.E., ImageNet classification with deep convolutional neural networks. Commun. ACM , 60, 6, 84–90, 2017.
3. Zeiler, M.D. and Fergus, R., Visualizing and understanding convolutional networks. Lect. Notes Comput. Sci. (including Subser. Lect. Notes Artif. Intell. Lect. Notes Bioinformatics , 8689 LNCS, PART 1, 818–833, 2014.
4. Simonyan, K. and Zisserman, A., Very deep convolutional networks for large-scale image recognition, 3rd Int. Conf. Learn. Represent. ICLR 2015 -Conf. Track Proc ., 1–14, 2015.
5. Szegedy, C. et al ., Going deeper with convolutions. Proc. IEEE Comput. Soc. Conf. Comput. Vis. Pattern Recognit . 07-12-June, 1–9, 2015.
6. He, K., Zhang, X., Ren, S., Sun, J., Deep residual learning for image recognition. Proc. IEEE Comput. Soc. Conf. Comput. Vis. Pattern Recognit . 2016-Decem, 770–778, 2016.
7. Xie, S., Girshick, R., Dollár, P., Tu, Z., He, K., Aggregated residual transformations for deep neural networks. Proc. -30th IEEE Conf. Comput. Vis. Pattern Recognition, CVPR 2017 . 2017-Janua, 5987–5995, 2017.
8. Hu, J., Squeeze-and-Excitation_Networks_CVPR_2018_paper.pdf, CVPR . 7132–7141, 2018.
9. Huang, G., Liu, Z., Van Der Maaten, L., Weinberger, K.Q., Densely connected convolutional networks. Proc. -30th IEEE Conf. Comput. Vis. Pattern Recognition, CVPR 2017 , 2017-Janua, 2261–2269, 2017.
10. Howard, A.G. et al ., MobileNets: Efficient Convolutional Neural Networks for Mobile Vision Applications, 2017.
11. Mader, A.O., Lorenz, C., Meyer, C., Segmenting Labeled Intervertebral Discs in Multi Modality MR Images. Springer Computational Methods and Clinical Applications for Spine Imaging: 5th International Workshop and Challenge, CSI 2018, Held in Conjunction with MICCAI 2018, Granada, Spain, September 16, 2018, 3, 11397, 178–180, 2019.
12. Bateson, M., Kervadec, H., Dolz, J., Lombaert, H., Ben Ayed, I., Constrained Domain Adaptation for Segmentation. Lect. Notes Comput. Sci. (including Subser. Lect. Notes Artif. Intell. Lect. Notes Bioinformatics) , 11765 LNCS, 326–334, 2019.
13. Zeng, G., Belavy, D., Li, S., Zheng, G., Evaluation and comparison of automatic intervertebral disc localization and segmentation methods with 3D multi-modality MR images: A grand challenge. Lect. Notes Comput. Sci. (including Subser. Lect. Notes Artif. Intell. Lect. Notes Bioinformatics) , 11397 LNCS, 163–171, 2019.
14. Liu, C. and Zhao, L., Intervertebral disc segmentation and localization from multi-modality MR images with 2.5D multi-scale fully convolutional network and geometric constraint post-processing. Lect. Notes Comput. Sci. (including Subser. Lect. Notes Artif. Intell. Lect. Notes Bioinformatics) , 11397 LNCS, 144–153, 2019.
15. Gao, Y., Deep learning framework for fully automated intervertebral disc localization and segmentation from multi-modality MR images. Lect. Notes Comput. Sci. (including Subser. Lect. Notes Artif. Intell. Lect. Notes Bioinformatics) , 11397 LNCS, 119–129, 2019.
16. Iriondo, C. and Girard, M., Vesalius: VNet-Based Fully Automatic Segmentation of Intervertebral Discs in Multimodality MR Images. Springer Computational Methods and Clinical Applications for Spine Imaging: 5th International Workshop and Challenge, CSI 2018, Held in Conjunction with MICCAI 2018, Granada, Spain, September 16, 11397, 175–177, 2019.
17. Dolz, J., Desrosiers, C. and Ayed, I.B., IVD-Net: Intervertebral disc localization and segmentation in MRI with a multi-modal Unet, Springer International Workshop and Challenge on Computational Methods and Clinical Applications for Spine Imaging, 11397, 130–143, 2018.
1 *Corresponding author: leenasilvoster@gmail.com
2
Location-Aware Keyword Query Suggestion Techniques With Artificial Intelligence Perspective
R. Ravinder Reddy1*, C. Vaishnavi1, Ch. Mamatha2 and S. Ananthakumaran3
1 Chaitanya Bharathi Institute of Technology, Hyderabad, India
Читать дальше