Keywords : Data mining, security, intrusion detection, anamoly detection, outlier detection, classification, privacy preserving data mining
A computer system has the ability to protect its valuable information, raw data along with its resources in terms of privacy, veracity and authenticity; this ability is known as computer security. A third party cannot read or edit the contents of a database by using the parameters i.e., Privacy/confidentiality and integrity. By using the parameter authenticity, an unauthorised person is not allowed to modify, use or view the contents of a database. When one or more resources of a computer compromises the availability, integrity or confidentiality by an action, it is known as intrusion. These types of attacks can be prevented by using firewall and filtering router policies. Intrusions can happen even in the most secure systems and therefore it is advisable to detect the same in the beginning. By employing data mining techniques, patterns of features of a system can be detected by an intrusion detection system (IDS) so that anomalies can be detected with the help of an appropriate set of classifiers. For easy detection of intrusion, some important data mining techniques such as classification and clustering are helpful.
Test data could be analysed and labelled into known type of classes with the help of classification techniques. For objects grouping into a set of clusters, clustering methods are used. These methods are used in such a way that a cluster has all similar objects. There could be some security challenges for mining of underlying knowledge from large volumes of data as well as extraction of hidden patterns by using data mining techniques (Ardenas et al. , 2014). To solve this issue, Privacy Preserving Data Mining (PPDM) is used, which aims to derive important and useful information from an unwanted or informal database (Friedman, Schuster, 2008). There are various PPDM approaches. On the basis of enforcing privacy principle, some of them can be shown in Figure 2.1.
a) Suppression
An individual’s private or sensitive information like name, salary, address and age, if suppressed prior to any calculation is known as suppression. Suppression can be done with the help of some techniques like Rounding (Rs/- 15365.87 can be round off to 15,000), Full form (Name Chitra Mehra can be substituted with the initials, i.e., CM and Place India may be replaced with IND and so on). When there is a requirement of full access to sensitive values, suppression cannot be used by data mining. Another way to do suppression is to limit rather than suppress the record’s sensitive information. The method by which we can suppress the identity linkage of a record is termed as De-identification. One such de-identification technique is k-Anonymity. Assurance of protection of data which was released against re-identification of the person’s de-identification (Rathore et al. , 2020), (Singh, Singh, 2013). K-anonymity and its application is difficult before collecting complete data at one trusted place. For its solution, secret sharing technique based cryptographic solution could be used.
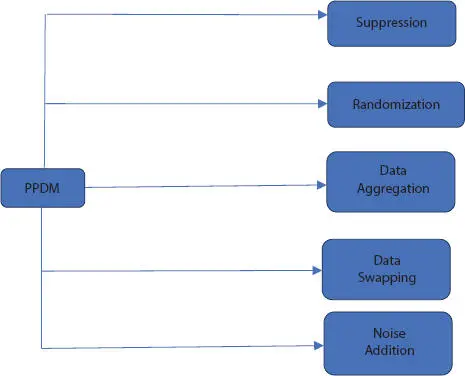
Figure 2.1 Privacy preserving data mining approaches.
b) Data Randomization
The central server of an organization takes information of many customers and builds an aggregate model by performing various data mining techniques. It permits the customers to present precise noise or arbitrarily bother the records and to find out accurate information from that pool of data. There are several ways for introduction of noise, i.e., addition or multiplication of the randomly generated values. To achieve preservation of the required privacy, we use agitation in data randomization technique. To generate an individual record, randomly generated noise can be added to the innovative data. The noise added to the original data is non-recoverable and thus leads to the desired privacy.
Following are the steps of the randomization technique:
1 After randomizing the data by the data provider, it is to be conveyed to the Data Receiver.
2 By using algorithm of distribution reconstruction, data receiver is able to perform computation of distribution on the same data.
c) Data Aggregation
Data is combined from various sources to facilitate data analysis by data aggregation technique. By doing this, an attacker is able to infer private- and individual-level data and also to recognize the resource. When extracted data allows the data miner to identify specific individuals, privacy of data miner is considered to be under a serious threat. When data is anonymized immediately after the aggregation process, it can be prevented from being identified, although, the anonymized data sets comprise sufficient information which is required for individual’s identification (Kumar et al. , 2018).
d) Data Swapping
For the sake of privacy protection, exchange of values across different records can be done by using this process. Privacy of data can still be preserved by allowing aggregate computations to be achieved exactly as it was done before, i.e., without upsetting the lower order totals of the data. K-anonymity can be used in combination with this technique as well as with other outlines to violate the privacy definitions of that model.
e) Noise Addition/Perturbation
For maximum accuracy of queries and diminish the identification chances its records, there is a mechanism provided by addition of controlled noise (Bhargava et al. , 2017). Following are some of the techniques used for noise addition:
1 Parallel Composition
2 Laplace Mechanism
3 Sequential Composition
2.2 Data Mining Techniques and Their Role in Classification and Detection
Malware computer programs that repeat themselves for spreading out from one computer to another computer are called worms. Malware comprises adware, worms, Trojan horse, computer viruses, spyware, key loggers, http worm, UDP worm and port scan worm, and remote to local worm, other malicious code and user to root worm (Herzberg, Gbara, 2004). There are various reasons that attackers write these programs, such as:
1 i) Computer process and its interruption
2 ii) Assembling of sensitive information
3 iii) A private system can gain entry
It is very important to detect a worm on the internet because of the following two reasons:
1 i) It creates vulnerable points
2 ii) Performance of the system can be reduced
Therefore, it is important to notice the worm ot the onset and categorize it with the help of data mining classification algorithms. Given below are the classification algorithms that can be used; Bayesian network, Random Forest, Decision Tree, etc. (Rathore et al. , 2013). An underlying principle is that the intrusion detection system (IDS) can be used by the majority of worm detection techniques. It is very difficult to predict that what will be the next form taken by a worm.
There is a challenge in automatic detection of a worm in the system.
Intrusion Detection Systems can be broadly classified into two types:
1 i) On the basis of network: network packets are reflected till that time unless they are not spread to an end-host
2 ii) On the basis of host: Those network packets are reflected which have already been spread to the end-host
Furthermore, encode network packets is the core area of host-based detection IDS to hit the stroke of the internet worm. We have to pay attention towards the performances of traffic in the network by focusing on the without encoding network packet. Numerous machine learning techniques have been used for worm and intrusion detection systems. Thus, data mining and machine learning techniques are essential as well as they have an important role in a worm detection system. Numerous Intrusion Detection models have been proposed by using various data mining schemes. To study irregular and usual outlines from the training set, Decision Trees and Genetic Algorithms of Machine Learning can be employed and then on the basis of generated classifiers, there could be labeled as Normal or Abnormal classes for test data. The labelled data, “Abnormal”, is helpful to point out the presence of an intrusion.
Читать дальше