(1.1) 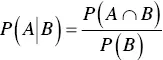
The support vector machine (SVM) is a supervised machine learning algorithm that is used for both classification and regression purposes. For a given training dataset, the SVM training algorithm builds a model that assigns new examples to one category or the other, making it a non‐probabilistic binary linear classifier.
1.2.3 AI Image Processing
Image segmentation techniques are useful also to detect product anomalies enabling predictive maintenance procedures of production lines [43]: quality monitoring of defect clusters checked on product surface, can be performed by image segmentation and image filtering techniques, predicting defectiveness. Defect prediction in manufacturing processing is achieved by ANNs [44]. The image vision technique [45, 46] also verifies the correct position of each product component, enabling pick and place removal action of defective pieces, according also to in‐line machine timing processing [45]. Image vision and mechatronic technologies improve the automation of quality check processes according to ISO9001:2015. A typical mechatronic scheme for real‐time defect in‐line control and actuation is illustrated in Figure 1.6where:
A product is moved on a conveyor belt.
The product passes in the image vision‐controlled area in a time between t1 and t2 (observation window for quasi real‐time processing).
The fixed smart camera locally processes the detected image by estimating the defect tolerance (output O).
If the measured defect overcomes the tolerance the input command (input i) of the pick and place robotic system is actuated, eliminating the piece.
The AI engine also predicts defect and machine failures thus improving the quality control of the whole production line.
Figure 1.6 Scheme of a pick and place automated system for defect removal, based on image processing.
The real‐time image processing in industrial applications permits:
Execution of a pre‐image filtering thus optimizing light and in general noise conditions (camera setting and auto‐calibration).
Extraction of the main features of the product to check contours, shape, color, etc.
Estimation of defect tolerance.
Provision of the driven command of mechanic components.
Classification of defects (defect classification by AI algorithms).
Estimation of defect clustering (by clustering algorithms)
Reconstruction of a 3D image useful to enhance defect features.
The post image processing modality allows prediction of the:
Number of defects.
Defect typologies according to the extraction of features (defect classification).
Machine failures (predictive maintenance application).
Image processing is a part of the whole process monitoring all the production in each stage. The phases of the image processing are shown in Figure 1.7, and are:
Pre‐filtering (image is adjusted by setting brightness, contrast, luminosity, background subtraction, red, green, and blue [RGB] filtering, and other parameters by optimizing noisy images for the post‐processing).
Image segmentation extracting features such as geometries and shapes directly classifying product defects (different techniques can be applied for defect classification such as the thresholding method, region and edge detection‐based techniques, clustering‐based techniques, watershed‐based techniques, partial differential equation‐based approaches, morphological segmentation, and others); image segmentation techniques are applied also for radiometric images.
Application of image post‐processing techniques based on AI algorithms classify defects enhancing hidden ones, by means of a training dataset structured by considering the specific defects associated with the production processes.
Defect classification and defect counting phase enabling defect storage into the DB system.
Defect detection enabling actuation processes by stopping or decelerating the machine in order to avoid further defective parts (the actuation process acts also to remove wrong pieces).
Defect prediction estimated by AI algorithms able to process historical production data, collected into the DB and big data system improving the training model.
Predictive maintenance of production machines analyzing and counting defective parts (the maintenance standard plan is modified by analyzing predictive maintenance results, thus decreasing drastically failure conditions).
Quality improvement performed by analyzing simultaneously predictive maintenance results and process production mapping.
Figure 1.7 Image processing and intelligent system in production processes.
The AI algorithms can be applied directly on the image to process. In this way, the convolutional neural networks (CNNs) [47] represent a good approach for defect classification [48] and laser positioning in industrial applications [49]. The typology of features to extract by the AI algorithms or by traditional image vision are different and are a function of the defect to detect. For example, in electronic welding the defects are classified as [45]:
Irregular welding geometries.
Big/small welds.
Non solder.
Irregular layout patterns.
Agglomerates (coupled welds).
No welds made (detection by welds counting).
Dirty welds.
Excess/less deposited welding materials.
Component missing.
Component shift.
Component lift.
Generic component damage.
Considering tire production industries, the defects are classified as:
Tread/belt separations.
Accumulation of contaminants in trapped areas (moisture, grease, oil, etc.).
Snaked, wavy or off centered steel belts.
Belt edge deformation (due to the anomalous mechanical stress).
Interrupted layout patterns (crack identification, tire stretching).
Swelled layout patters (contaminants accumulation).
Misaligned patterns.
Added edges on the basic patterns (contaminants accumulation).
Different height of the elements constituting the patterns.
Writing not correctly printed.
Layout elements unified.
Different agglomerated contaminant materials (inhomogeneous geometry characterized by different volumes).
Misalignment between tire and wheel.
Others.
All these features are classified by particular image features extracted by image processing algorithms. Concerning the welding control of a large metallic surface as for tank production, IRT provides more information about weld homogeneity. Other algorithms, such as the K‐means clustering algorithm, are applied in order to enhance for example leakages [13], or in general for radiometric defect detection in photovoltaic systems [48].
1.2.4 Production Process Mapping
Process mapping represents a very important procedure to follow for quality control. All production data provided by sensors, and also results of the image processing tools, are in general stored in DB systems and mapped by diagrams such as fishbone diagrams, p ‐charts, the Plan‐Do‐Check‐Act (PDCA) cycle, and by XmR charts, thus improving the quality check during the time. The PDCA cycle, also known as the Deming cycle diagram, is a four‐step iterative management method used for the control and continuous improvement of processes and products. Steps in PDCA include:
Читать дальше