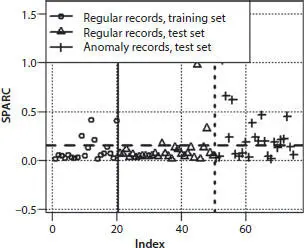
Figure 3.7 Sparsity values on the records of the training and test sets. Horizontal dashed line - anomaly detection cutoff producing an expected false discovery rate of 20%.
Figure 3.8 Anomaly detection. Histograms of posterior true discovery rate (TDR) for two values of expected false discovery rate. AD method: aggregated. 20 regular records in each training set.
Table 3.2 Mean and three quantiles of distribution of AUC values.
|
Mean |
Quantiles |
Width of quantile region |
P=0.05 |
Median P=0.5 |
P=0.95 |
Divergence |
0.897 |
0.89 |
0.895 |
0.909 |
0.019 |
Aggregated |
0.866 |
0.862 |
0.87 |
0.901 |
0.039 |
Distance |
0.795 |
0.633 |
0.818 |
0.885 |
0.252 |
Sparsity |
0.765 |
0.576 |
0.786 |
0.868 |
0.292 |
The results shown in Figure 3.8characterize the performance of an adaptive aggregated classifier that was applied to 1000 bootstrap randomly formed training - test datasets. Optimization of the aggregated classifier was done on the part of the test records identified by the sparsity as anomaly. Figure 3.8shows histograms of values of the posterior true discovery rate for expected false discovery rates of 20% and 5%. According to Figure 3.8, a higher expected false discovery rate of 20% leads to a higher posterior true discovery rate in the range of 83%- 87%. In the case of lower expected FDR of 5%, posterior discovery rate is within 70% -85%.
The results of comparative analysis of the performance of several classifiers in detection of gas-filled sand anomaly are shown in the Table 3.2. This table shows mean, median, and two quantiles of posterior AUC values for four classifiers. According to the Table 3.2, the highest quality results are produced with the divergence classifier. Performance of the adaptive aggregated classifier is slightly lower but close to performance of divergence. The distance and the sparsity classifiers are characterized by smaller median AUC values and wider widths of the quantile region.
3.7 Bootstrap Based Tests of Anomaly Type Hypothesis
The hypothesis test discussed in this section is the first step in a more definite and automatic determination of anomaly type. It relies on the use of the anomaly indicator defined by Eq. 3.11:
(3.11) 
detectedAnomalyPart - is the dataset formed by records identified as anomaly by an AD classifier, φj - anomaly characterization functions on average taking larger values on regular records and smaller values on records within hypothesised anomaly, ηj - positive coefficients, J is the total number of anomaly characterization functions.
Further we use Poisson’s ratio as a single anomaly characterization function and assign a single η1 value 1.
In that case, anomalyIndicator takes the form:
(3.12) 
According to Ostander [22], high-porosity, brine-saturated sandstones tend to have high Poisson’s ratios of 0.30 to 0.40, while gas-saturated sandstones may have a Poisson’s ratio as small as 0.1. Data presented by Jain et al. [23] show that Poisson’s ratio for gas-saturated sands is in the range of 0.2-0.25, so that the largest possible value of the anomaly indicator (3.12)in the case of a gas-filled sand anomaly is around 0.8. So the following assumption might be valid for gas-saturated sands with porosity in a wide range of values: If AnomalyIndicator is larger than 0.8, then the anomaly type is not gas-saturated sands. Then the hypothesis H0 for anomaly type with alternative H1 may take the form:
(3.13) 
where significance cutoff = 0.8 and significance level α = 0.05.
Hypothesis H0 is rejected if the probability to exceed the significance cutoff is smaller than a predefined significance level. Rejection of hypothesis H0 does not contradict the assumption that the detected anomaly is gas-filled sands. If hypothesis H0 is not rejected, then there is a contradiction between the results of the test and the assumption that the detected anomaly is gas-filled sands.
The anomalyIndicator in Eq. 3.12is a random variable which value is affected by many random factors. These factors include the noise that distorts records in the training and test sets, the variability of the detectedAnomalyPart, and the non-zero posterior false discovery rate. To test the anomaly type hypothesis and to estimate the p-value we used a bootstrap that generates multiple pairs of training-test sets and, respectively, multiple bootstrap samples of anomalyIndicator. In the experiment presented in this section, the number of bootstrap-generated samples of anomalyIndicator calculated for four AD classifiers was 1000. These samples were used to calculate distributions of the anomaly indicators and to estimate the probability of exceeding the significance cutoff by the anomalyIndicator.
Figure 3.9 Histograms of anomalyIndicator for three types of classifiers. (a)-adaptive aggregated classifier, (b)-distance, (c)-sparsity. Intersection of vertical dashed lines with x-axis - value of significance level
Table 3.3 Percent of values of anomalyIndicator exceeding significance cutoff α (p-value).
Number of records in the train set |
Expected false discovery rate (%) |
Percent of values of anomalyIndicator exceeding significance level (p-value) |
Sparsity |
Distance |
Aggregated |
25 |
20 |
0.2 |
3.7 |
0.01 |
20 |
20 |
2.1 |
16.2 |
0.04 |
15 |
20 |
14.5 |
36.4 |
0.1 |
12 |
20 |
28.7 |
47.4 |
0.8 |
10 |
20 |
42.5 |
47.5 |
1.7 |
7 |
20 |
69.2 |
56.5 |
6.7 |
5 |
20 |
77.3 |
60.4 |
9.9 |
Figure 3.9shows three histograms of bootstrap generated values of anomaly indicators for three types of AD classifiers. Vertical dashed lines are drawn at the value of significance cutoff a equal to 0.8. Histograms were calculated for the 20 records training sets. According to Figure 3.9, distribution of the anomaly indicator is the narrowest and is shifted in the direction of small values when an adaptive aggregated classifier is used. Distribution obtained with the distance classifier is characterised by the largest percent of values of an indicator exceeding the significance level a. Generally, the percent of anomalyIndicator samples exceeding the significance cutoff is higher for universal classifiers compared to the aggregated classifier.
Читать дальше