What’s the soonest it could happen? Even if we knew the best possible way to build human-level AGI using today’s computer hardware, which we don’t, we’d still need to have enough of it to provide the raw computational power needed. So what’s the computational power of a human brain measured in the bits and FLOPS from chapter 2? *4This is a delightfully tricky question, and the answer depends dramatically on how we ask it:
• Question 1: How many FLOPS are needed to simulate a brain?
• Question 2: How many FLOPS are needed for human intelligence?
• Question 3: How many FLOPS can a human brain perform?
There have been lots of papers published on question 1, and they typically give answers in the ballpark of a hundred petaFLOPS, i.e., 10 17FLOPS.58 That’s about the same computational power as the Sunway TaihuLight (figure 3.7), the world’s fastest supercomputer in 2016, which cost about $300 million. Even if we knew how to use it to simulate the brain of a highly skilled worker, we would only profit from having the simulation do this person’s job if we could rent the TaihuLight for less than her hourly salary. We may need to pay even more, because many scientists believe that to accurately replicate the intelligence of a brain, we can’t treat it as a mathematically simplified neural-network model from chapter 2. Perhaps we instead need to simulate it at the level of individual molecules or even subatomic particles, which would require dramatically more FLOPS.
The answer to question 3 is easier: I’m painfully bad at multiplying 19-digit numbers, and it would take me many minutes even if you let me borrow pencil and paper. That would clock me in below 0.01 FLOPS—a whopping 19 orders of magnitude below the answer to question 1! The reason for the huge discrepancy is that brains and supercomputers are optimized for extremely different tasks. We get a similar discrepancy between these questions:
How well can a tractor do the work of a Formula One race car?
How well can a Formula One car do the work of a tractor?
So which of these two questions about FLOPS are we trying to answer to forecast the future of AI? Neither! If we wanted to simulate a human brain, we’d care about question 1, but to build human-level AGI, what matters is instead the one in the middle: question 2. Nobody knows its answer yet, but it may well be significantly cheaper than simulating a brain if we either adapt the software to be better matched to today’s computers or build more brain-like hardware (rapid progress is being made on so-called neuromorphic chips).
Hans Moravec estimated the answer by making an apples-to-apples comparison for a computation that both our brain and today’s computers can do efficiently: certain low-level image-processing tasks that a human retina performs in the back of the eyeball before sending its results to the brain via the optic nerve.59 He figured that replicating a retina’s computations on a conventional computer requires about a billion FLOPS and that the whole brain does about ten thousand times more computation than a retina (based on comparing volumes and numbers of neurons), so that the computational capacity of the brain is around 10 13FLOPS—roughly the power of an optimized $1,000 computer in 2015!
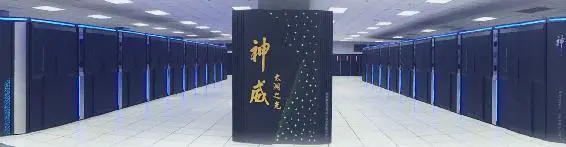
Figure 3.7: Sunway TaihuLight, the world’s fastest supercomputer in 2016, whose raw computational power arguably exceeds that of the human brain.
In summary, there’s absolutely no guarantee that we’ll manage to build human-level AGI in our lifetime—or ever. But there’s also no watertight argument that we won’t. There’s no longer a strong argument that we lack enough hardware firepower or that it will be too expensive. We don’t know how far we are from the finish line in terms of architectures, algorithms and software, but current progress is swift and the challenges are being tackled by a rapidly growing global community of talented AI researchers. In other words, we can’t dismiss the possibility that AGI will eventually reach human levels and beyond. Let’s therefore devote the next chapter to exploring this possibility and what it might lead to!
THE BOTTOM LINE:
• Near-term AI progress has the potential to greatly improve our lives in myriad ways, from making our personal lives, power grids and financial markets more efficient to saving lives with self-driving cars, surgical bots and AI diagnosis systems.
• When we allow real-world systems to be controlled by AI, it’s crucial that we learn to make AI more robust, doing what we want it to do. This boils down to solving tough technical problems related to verification, validation, security and control.
• This need for improved robustness is particularly pressing for AI-controlled weapon systems, where the stakes can be huge.
• Many leading AI researchers and roboticists have called for an international treaty banning certain kinds of autonomous weapons, to avoid an out-of-control arms race that could end up making convenient assassination machines available to everybody with a full wallet and an axe to grind.
• AI can make our legal systems more fair and efficient if we can figure out how to make robojudges transparent and unbiased.
• Our laws need rapid updating to keep up with AI, which poses tough legal questions involving privacy, liability and regulation.
• Long before we need to worry about intelligent machines replacing us altogether, they may increasingly replace us on the job market.
• This need not be a bad thing, as long as society redistributes a fraction of the AI-created wealth to make everyone better off.
• Otherwise, many economists argue, inequality will greatly increase.
• With advance planning, a low-employment society should be able to flourish not only financially, with people getting their sense of purpose from activities other than jobs.
• Career advice for today’s kids: Go into professions that machines are bad at—those involving people, unpredictability and creativity.
• There’s a non-negligible possibility that AGI progress will proceed to human levels and beyond—we’ll explore that in the next chapter!
*1 If you want a more detailed map of the AI-safety research landscape, there’s an interactive one here, developed in a community effort spearheaded by FLI’s Richard Mallah: https://futureoflife.org/landscape.
*2 More precisely, verification asks if a system meets its specifications, whereas validation asks if the correct specifications were chosen.
*3 Even including this crash in the statistics, Tesla’s Autopilot was found to reduce crashes by 40% when turned on: http://tinyurl.com/teslasafety.
*4 Recall that FLOPS are floating-point operations per second, say, how many 19-digit numbers can be multiplied each second.
Chapter 4 Intelligence Explosion?
If a machine can think, it might think more intelligently than we do, and then where should we be? Even if we could keep the machines in a subservient position…we should, as a species, feel greatly humbled.
Alan Turing, 1951
The first ultraintelligent machine is the last invention that man need ever make, provided that the machine is docile enough to tell us how to keep it under control.
Irving J. Good, 1965
Since we can’t completely dismiss the possibility that we’ll eventually build human-level AGI, let’s devote this chapter to exploring what that might lead to. Let’s begin by tackling the elephant in the room:
Can AI really take over the world, or enable humans to do so?
Читать дальше