EVOLUTIONARY BIOROBOTICS
The idea of evolving robots is not new to my laboratory. Stefano Nolfi and Dario Floreano brought the concept to the general academic world with their book, Evolutionary Robotics , which was published in 2000. From the context of artificial intelligence, cognitive science, and engineering, they helped create a framework in which researchers could harness evolutionary processes—randomness, selection, and differential reproduction—to create without their guidance new kinds of behaviors and intelligence in mobile robots.
What we’ve done is to take Nolfi and Floreano’s evolutionary robotics framework and apply it to biology (Figure 2.3). Whereas Nolfi and Floreano weren’t originally trying to build biologically realistic robots, that’s where we start. And the inspiration for that approach came from Barbara Webb, an invertebrate neuroscientist and behaviorist who figured out that she could use robots to test hypotheses about the neural underpinnings of animal behavior. [9] Barbara Webb, “Can Robots Make Good Models of Biological Behaviour?” Behavioral and Brain Sciences 24, no. 6 (2001): 1033–1055.
When this approach—using physical robots to test hypotheses about biological systems—is thought of in general terms, Webb calls the field biorobotics. The combination of these two approaches creates evolutionary biorobotics.
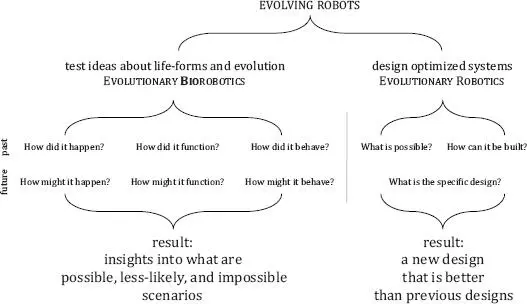
FIGURE 2.3. People evolve robots for two main purposes: to test ideas about evolution and to design new kinds of robots. In our laboratory at Vassar College we create evolving robots in physically embodied or digital form to test ideas about animals, evolution, and behavior. We also create evolving robots to make new designs for intelligent machines.
So if we’re going to build robots that can really play the game of life, they must be able to reproduce, have behaviors and other traits that are genetically heritable, and have limits placed on the number of offspring that can be reproduced. Putting these features into a robotic system gives us what we like to call the lifecycle of evolving robots (Figure 2.4).
To be frank, evolutionary biorobotics has four important limitations when it deals with extinct species and their evolution. First, as we discussed earlier when talking about the kinds of evidence that you need to explain an adaptation (Figure 2.2), analyses of past selection are fraught with potentially crippling and untestable assumptions about the genetic structure of the population; the genetics of traits in question; and the pattern, strength, and phenotypic targets of selection. Second, what you can reconstruct and test is only the ecological function of the character, the selection environment, and the response of the population to selection. Third, because we create model simulations with our robots, our reasoning is by analogy. So as we set out to explore the evolution of backbones in robotic fish, the best we could hope for was robust support—in digital and embodied populations—for the prediction that selection for swimming abilities drove the evolution of the backbone in real fish. In the worst case, the best we’d be able to say is the obvious: that different selection environments can produce different results in different robot-world systems. Fourth and finally, our use of digital and embodied robots interacting in constructed worlds grossly simplifies the animal, its environment, and the animal-environment interaction.
Still, there is much to be excited about: at the minimum, if varying our robotic backbones changes robotic behavior, at least we’d have a proof of concept that we were studying an important variable that may or may not have been under selection at some point. Second, the fact that robots evolve can give us insight into how the process of adaptation works, whether in robots or biological organisms. And at least we knew we were in good company: model simulations with digital agents have already been used, most notably by Charles Ofria and Richard Lenski at the Digital Evolution Laboratory at Michigan State, to test a range of biological hypotheses about evolution.
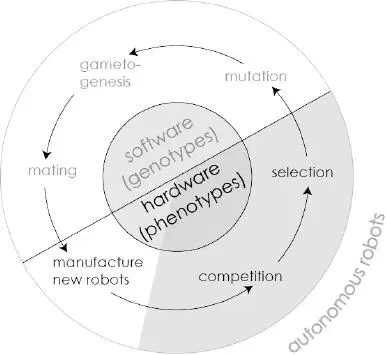
FIGURE 2.4. The lifecycle of evolving robots. Although all the behavioral interaction and selection in the population occurs when autonomous and embodied robots are competing (dark gray pie slice), their lifecycles also involve complex genetic interactions that occur in software (light gray font). Who gets to mate is based on evolutionary fitness as judged by a predetermined set of rules (the “fitness function”). Because the genetic interactions involve processes like mutation and mating, the genetic instructions for the next generation of robots are the outcome of random processes (mutation, mating) and nonrandom selection. One spin around the lifecycle equals one generation.
That left us with the task of designing our first biorobots. Let’s engineer some players for the game of life.
Chapter 3
ENGINEERING EVOLVABOTS
“IF YOU UNDERSTAND IT, YOU CAN BUILD IT.” [10] Richard Feynman, the Nobel Laureate in Physics, said something similar: “What I cannot create, I do not understand.”
THIS IS THE engineers’ secret code. It is so secret, in fact, that I can’t be sure that it’s real. No engineer has ever said this to me, a non-engineer, but I’m guessing that it’s the last question on every licensing exam and is whispered during their secret handshake.
Regardless of whether they say it, it’s definitely how they work. I figured this out for myself, having worked with many of them over the years, building robots. Engineers decide what their device should do—they understand it—and then they build it. Not surprisingly, that attitude drove me crazy—I was working and thinking in the opposite direction. At one company’s early design meeting, in which the hardware, software, and mechanical engineers were pressing me for specifications, I let loose my exasperation: “Let’s build the robot and then see what she can do! If I knew the specifications, then I’d know the answer. What we are doing is testing a hypothesis!” Silence. With eyebrows raised and knowing looks exchanged, the three engineers politely ended the meeting with a collective, “We’ve gotta get back to work.”
This left me sitting with an old friend, Charles, a.k.a. “Chuck” Pell, a chief designer at the company and someone trained as a sculptor and not, I began to appreciate, as an engineer. He interpreted. Chuck explained that engineers never use the word “hypothesis,” were never taught about how you test one, and were, instead, trained to build contraptions that work to do a job. The job that a contraption does, he continued, is defined by the specifications. So most engineers are literally lost without the specifications. You can’t get somewhere without knowing where you are going. This all makes sense, I conceded. But it doesn’t tell someone interested in evolutionary biorobotics the first thing about how to proceed. Damn the code! [11] What’s really useful about the secret code is that it implies that if you can’t build it (whatever “it” is), then you don’t really understand it. This is another way of thinking about what people call an “existence proof,” which is the ultimate in physical evidence: if it exists, then it can exist.
Or don’t. What I’ve learned since then—thanks to designers like Chuck and his band of merry engineers—is that the code provides a great starting point for any kind of design, even the crazy stuff that we do with evolving robots. In fact, implicitly, the code got us started in Chapter 2, and thanks to it, we now understand more about the game of life, evolution, and how we might go about simulating it. In this chapter we’ll stick to the code and go hunting for an understanding of something more elusive—the first vertebrate. Understanding those first fish-like vertebrates—what they looked like and how they behaved over five hundred million years ago—will help us design and engineer the robotic agents that become the players in our simulation of the game of life.
Читать дальше