Experiments used Refs. [10–12] as stated in Figure 1.2. Hellenic sugar Industry used FINKNN for sugar production forecast based on the population of assessment. FINKNN is studied as K-nearest neighbor classifier that performs over the metric lattice of traditional convex fuzzy set. Results proved that FINKNN showed improved results for efficiency in forecasting.
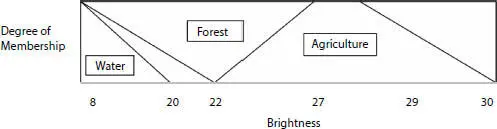
Figure 1.2 Fuzzy cluster membership function representation in various field [9].
Decision trees are the supervised learning techniques used in machine learning. The Decision Tree model is comprised of nodes, branches, leaf, terminal value, payoff distribution, rollback and certain equivalent and method. Decision trees have three variants of nodes and two variants of branches. Square is used to represent one of the decision points. The decision node is a point of choice for the tree. The decision nodes extend decision branches. Each node toward the end of the tree is called terminal node. There is an associated value associated with terminal node commonly referred to as payoff, outcome value. The terminal value is the measure of sequences of decisions or the resultant of the scenario in the tree. The construction of Decision tree algorithm is a two-step process that includes growth of tree and pruning. In the growth step the large decision tree is created, reduced and overfitting is removed. The second step does the tree pruning to reach a decision. The classification tree used for decision making is the obtained pruned tree [13].
Prediction is influenced by various factors in agricultural explorations [14]. Variables associated with agronomics, application of nitrogen and weed control were used for machine learning and decision tree for yield forecasting and development of yield mapping. Both decision trees and ANN were implemented and results showed that greater accuracies were obtained from ANN results.
Authors [15] modeled productivity of soybean using decision trees. Data for climate in Bhopal district was collected for since 1984 to 2003 considering the climatic factors of evaporation, minimum and maximum temperature, humidity, and rainfall. The factors were studied for the production of soybean crop. Interactive Dichotomizer3 (ID3) algorithm was implemented on data. ID technique is based on information and two assumptions. Relative humidity was found to be a major parameter that affected soybean crop yield. Few rules were generated that helped know the lowest and highest prediction of soybean. The only one limitation of the model was that, the amount of yield production cannot be predicted [4, 16], as shown in Figure 1.3.
The vast climatic diversity of India impacts the agricultural production in several parts of the country. Convenient decisions can be made by the farmers and policy inventors if the production can be forecasted in advance.
Figure 1.3 Decision tree structure for crop details prediction [4].
Crop Advisor is one of the advancement in this area. It is a user friendly webpage that identified the impact of weather parameters on the yield of crops. Crop Advisor implements C4.5 algorithm. The most effective parameter of the climate on the yields of specified crops in selected region of Madhya Pradesh was ascertained using C4.5. There is boom in cloud based decision and support system for agriculture these days. There exists a Decision support and Automation system (DSAS) to assist farmers and growers. Users of the application have controls for different features in web portal. There are different stages in DSAS. DSAS provides farmer with real time data via interconnection of several devices. The farmer had right to monitor the real time data and control the machine through software. Few another systems like spray controller will spray defined amount of pesticide in fields, irrigation controller manage irrigation and fertilizer controller takes care of fertilizer. The data to DSAS is given by different sensors of climate and soil [17].
1.1.1.4 Regression Analysis
There exist several statistical techniques for crop production. One of the most widely used is regression analysis [18, 19]. Regression models were developed as a technique for prediction of response variable called yield and weather, soil properties were addressed as explanatory variables [20]. Several yield forecasting models used parametric regression by taking known functional forms of the predictor variables [21]. Authors [22] described the linear regression as commonly used models for crop production. Researchers also found the use of polynomial regression models in nonlinear regression models in for agricultural applications [23]. The influence of temperature on Jowar crop was forecasted in Jowar production system. The production, minimum and maximum temperatures were the parameters of the experiment [4]. The significance was tested where 2-tailed test approach and Pearson correlation coefficients were used. The results obtained were significant at 0.01 level. Then regression analysis was carried out for yield of crop and measures of temperature. Results showed that that the Jowar yields were very less dependent on the temperature yield was highly affected by some other factors. The reduced temperature increased the yield of Jowar crops. Experiments used functional liner regression analysis to relate yield with rainfall and temperature as predictor variable [4, 24].
Correlation analysis was done taking the yield as output variable and temperature and precipitation as prediction parameters. Stepwise regression technique was used to select best predictors [4, 25]. The crop production changes with the changes in climate. This effect of changing climatic variables on crop production was studied under anticipated seasonal climate change conditions. Daily weather data was obtained from weather generator and authors used the multiple linear regression models. The parameters referencing the climate such as measures of minimum and maximum temperature, density of rainfall, humidity and precipitation rate, speed of wind and solar radiation were used for analysis. These factors were used to predict the corn yield they have results implied that climate variability significantly affects crop yields [4].
The process of identifying similar objects that are different from individuals in other groups is called Cluster analysis or clustering. Clustering finds wide usage in data analysis and many other fields such as machine learning, recognition patterns, analyzing images, retrieval of information and agriculture, etc. Clustering can be studied with several algorithms such as k-means, k-medoid, etc. K-means is most common and widely used clustering algorithm [26]. Demonstration of modified k-Means clustering algorithm in prediction of crop was done in Ref. [4]. Comparison of results for modified k-Means over k-Means and -Means++ clustering algorithms was done and it was found that the modified k-Means had the maxi-mum number of highly differentiable good quality clusters, highly correct results of crop prediction and maximum count for accuracy.
A weather forecast model was developed for classifying the metrological data. The model was based on the frequency of variables. Patterns associated to severe convective activity were identified for the task. Brazilian regions were spot for collection of features during summer of 2007. A fairly good classification performance was seen in results [27].
Читать дальше