1 ...8 9 10 12 13 14 ...18 10 Imbalance between availability of number of normal class images and images with disorders and disproportion of availability of annotated and unannotated images.
11 Most of the models are trained for ImageNet data set which has altogether different features as compared to medical images [5].
12 Confounding variables in the x-ray images ruin the performance of models therefore there is a need of pre-processing [72].
To overcome these issues, following strategies can be deployed.
1 2-D or 3-D image patches are taken as an input rather than full sized image [11, 14, 36, 50, 56–58, 63].
2 Annotate existing images using affine transformation and expand existing dataset by adding annotated image with existing images, and then, network will be trained from scratch on expanded dataset [11, 50, 56, 57].
3 Use of deep models trained on off-the shelf dataset for feature extraction and then use final classifier at last layer for classification by fine tuning the model with target dataset [14, 36, 58].
4 Initialize parameters of new models with value of parameters of pre-trained models applied on ImageNet dataset [60] and then fine tune the network with task specific dataset.
2.2.2 Performance Measuring Parameters
There are various parameters used by researchers to evaluate performance of their models, namely, ROC, AUC, F1-score, recall, accuracy, specificity, and sensitivity. Use of metric varies from application to application, and sometimes, single parameter does not justify the working of models. In such cases, subset of parameters are used to evaluate performance of model. For testing the accuracy of classification models, accuracy, precision, recall, F1-score, sensitivity, specificity, ROC curve, and AUC curve are utilized on the basis of which comparison is performed in the existing research. These parameters are discussed as follows.
1 Accuracy: It is defined as the ratio of number of correctly classified chest pathologies to the number of available samples. If the dataset has 1,000 images having some pathology or no-pathology and the model has correctly classified 820 pathologies and 10 as normal cases, then accuracy will be 830×100/1,000 = 83%.
2 Precision: When there is imbalance in dataset with respect to availability of class-wise images, then accuracy is not an acceptable parameter. In such cases, model is tuned to major class only which does not make sense. For example, if in CXR dataset, images belonging to class nodule are more, then predicting maximum images of most frequent class will not solve the purpose. Therefore, class specific metric is needed known as precision.
3 Recall: It is also a class specific parameter. It is a fraction of images correctly classified for given class.
4 F1-Score: For critical application, F1-score is needed which will guide which parameter is more appropriate, i.e., precision or recall. Sometime, both metrics are equally important. Therefore, F1-score is a combination of both which is calculated as:
5 Sensitivity and Specificity: These metrics are generally used for medical application which are calculated as follows.
6 ROC Curve: It is a Receiver Operating Characteristic curve generally used to measure the performance of binary classifier model. This curve is plotted as True-Positive Rate against False-Positive Rate. It depicts overall performance of model and helps in selecting good cut-off threshold for the model.
7 AUC Curve: Area Under Curve (AUC) is a binary classifier’s aggregated output metric for all possible threshold values (and therefore it is threshold invariant). Under the ROC curve, AUC calculates the field, and hence, it is between 0 and 1. One way to view AUC is as the likelihood that a random positive example is ranked more highly by the model than a random negative example.
2.2.3 Availability of Datasets
Mostly, deep learning models used for identification of chest radiography pathologies and training processes are carried out on the basis of available CXR datasets, of which the most famous datasets are the Indiana dataset [15], KIT dataset [54], MC dataset [29], Japanese Society of Radiological Technology (JSRT) dataset [59], ChestX-ray14 dataset [19], NIH Tuberculosis Chest X-ray [17], and Belarus Tuberculosis [6]. There are major limitations to each cited dataset, some of which are addressed in a survey published in August 2018 [48]. The ChestX-ray14 is recognized as one of the most widespread CXR datasets among the available datasets, which contains 108,948 x-ray images obtained from 32,717 patients. These images are labeled by means of natural language processing with one or more diagnostic labels. A number of recent AI reports, such as Wang et al . [70], Yao et al . [71], Rajpurkar et al . [49], and Guan et al . [4], have used ChestX-ray14 dataset. All of these studies are trained and tested on ChestX-ray14 dataset accompanied with annotation for 14 different types of chest pathologies. Details of availability of number of each type of pathology in ChestX-ray14 dataset are shown in Table 2.1[7].
It is observed that there is a presence of disproportion in the number of available images among 14 chest pathologies. This is one of the factors affecting performance of different deep models. Before analyzing existing models, 14 chest pathologies are described as follows in Figure 2.2.
1 Atelectasis: It is a disorder where there is no space for normal expansion of lung due to malfunctioning of air sacs in it.
2 Cardiomegaly: It is a disorder related to heart where heart enlarged due to stress or some medical condition.
3 Consolidation: When the small airways in lungs are filled with fluids like pus, water, or blood instead of air, then consolidation occurs.
4 Edema: It occurs due to deposition of excess fluid in lungs.
5 Effusion: In this disorder excess fluid filled in between chest wall and lungs.
6 Emphysema: Alveoli which are known as air sacs of lungs when damaged or get weak then person suffers with Emphysema.
7 Fibrosis: When lung tissues get thickened or stiff, then it becomes difficult for lungs to work normally. This condition is known as fibrosis.
8 Hernia: protuberance of thoracic contents outside their defined location in thorax region is known as thoracic hernia.
9 Infiltration: When there is a trail of denser substance such as pus, blood, or protein occurs within the parenchyma of the lungs, then it is known as a pulmonary infiltration.
10 Mass: It is a tumor that grows in mediastinum region of chest that separates the lungs is termed as Mass.
11 Nodule: A small masses of tissue in the lung are known as lung nodules.
12 Pleural Thickening: When the lung is exposed to asbestos, it causes lungs tissue to scar. This condition is known as pleural thickening.
13 Pneumonia: When there is an infection in air sacs of either or both lungs, then its results in Pneumonia.
14 Pneumothorax: When air leaks from lungs into the chest wall then this condition is known as Pneumothorax disorder.
Table 2.1 Details of ChestX-ray14 dataset.
Type of pathology |
No. of images with label |
Type of pathology |
No. of images with label |
Atelectasis |
11559 |
Consolidation |
4,667 |
Cardiomegaly |
2776 |
Edema |
2,303 |
Effusion |
13317 |
Emphysema |
2,516 |
Infiltration |
19894 |
Fibrosis |
1,686 |
Mass |
5782 |
Pleural thickening |
3,385 |
Nodule |
6331 |
Hernia |
227 |
Pneumonia |
1431 |
Normal chest x-ray |
60,412 |
Pneumothorax |
5302 |
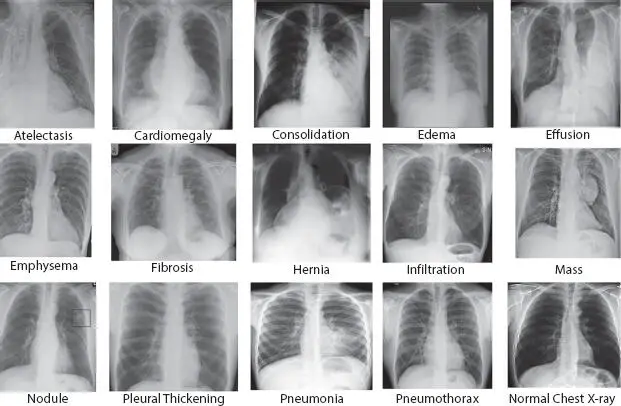
Читать дальше