FP : the number of incorrect classifications of negative examples (false positive)
TN : the number of correct classifications of negative examples (true negative)
Precision is defined as the percentage of correctly identified documents among the documents returned; whereas recall is defined as the percentage of relevant results among the correctly identified documents. Practically, high recall is achieved at the expense of precision and vice versa [61]. However, the metric f-measure is suitable when single metric is needed to compare different models. It is defined as the harmonic mean of precision and recall. Based on the confusion matrix, the precision, the recall, and the f-measure of the positive class are defined as
(1.3) 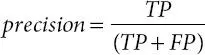
(1.4) 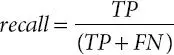
(1.5) 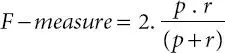
(1.6) 
Ontology-based Semantic Indexing (OnSI) model is evaluated by the metrics such as precision, recall, and accuracy, as shown in Equations 1.3, 1.4, 1.5, and 1.6.
Document collection like Healthcare service reviews (Dataset DS1) were collected from different social media web sites for 10 different hospitals, as detailed in Tables 1.2and 1.3.
Table 1.2 Social media reviews for healthcare service (DS1).
|
Number of reviews |
Data source |
Positive |
Negative |
Twitter |
1200 |
525 |
Mouthshut.com |
425 |
110 |
BestHospitalAdvisor.com |
200 |
85 |
Google Reviews |
580 |
320 |
Total Reviews |
2405 |
1040 |
Table 1.3 Number of reviews of features and hospitals (DS1).
Features |
Reviews |
Cost |
663 |
Medicare |
748 |
Nursing |
776 |
Infrastructure |
554 |
Time |
704 |
Number of reviews |
H1 |
596 |
H6 |
308 |
H2 |
411 |
H7 |
313 |
H3 |
399 |
H8 |
297 |
H4 |
227 |
H9 |
281 |
H5 |
252 |
H10 |
361 |
Table 1.3shows that 663 reviews were collected for the feature “cost,” and 596 reviews were collected for the hospital H1. The list of features is identified from the previous work, related articles, and social media websites. For example, the document [62] clearly identified the necessary criteria for healthcare services. The expectations of patients such as cost, hospital characteristics, infrastructure facility, recommendations by other users, treatment and nursing care, and medical expertise were clearly mentioned in the article [63]. Features were also selected by referring web sites like, BestHospitalAdvisor, Mouthshut.com, webometrics, and so on. The comments given by patients or by their care takers in these websites were analyzed for different features of healthcare services of these different hospitals.
1.7 Results and Discussions
Ontology-based Semantic Indexing (OnSI) model builds domain Ontology for each product/service review documents using the selected features from the CFS LDAmodel. Protégé software is used to build and query the Ontology model. The top five terms selected by LDA model, related to each topic for the dataset is shown in Table 1.4. Each topic is manually labeled in context with the first term in the list. It is difficult to carry out human annotation for all the terms grouped under the topic.
Table 1.4 List of top five terms by LDA model.
Topics/features of (DS1) |
Feature terms by FSLDA model (DS1) |
Topic 1—Cost |
cost, test, money, charge, day |
Topic 2—Medicare |
doctor, nurse, team, treatment, bill |
Topic 3—Staff |
staff, patient, child, problem, face |
Topic 4—Infrastructure |
hospital, people, room, experience, surgery |
Topic 5—Time |
time, operation, hour, service, check |
For example, the term “bill” is one of the top words under the topic “medicare.” However, it is more related to the topics “cost” or “infrastructure” or “time.” Similarly, the term “appointment” is not present in any of the list under top 5 or top 10 terms; however, it is more appropriate to the topics “time” and “medicare.” In order to alleviate this problem, the CFS LDAmodel selects the representative terms of each topic with reference to the first term (the term which has the highest term-topic probability in each topic) in the list, using the correlation analysis. As stated in the previous example, the term “bill” is not related to the term “doctor,” and it is highly correlated with the terms “cost,” “hospital,” and “time.” The correlation values of these terms are shown in Table 1.5. For example, the term-topic probability “Φ tw” of “room” is 0.0134 and correlated value “c” with “cost” is 0.0222. As stated in another example, the term “appointment” is highly correlated with the terms “doctor” and “time,” and it is grouped under the topic “medicare” and “time” as shown in Table 1.5. As an another example, the term “disease” is related with “doctor” and “hospital,” and it is not related with the terms “cost,” “time,” and “staff,” as shown in Table 1.5.
Table 1.5 Sample correlated terms selected by CFS LDA.
Features |
Cost |
Medicare |
Staff |
Infrastructure |
Time |
High probable terms |
cost |
doctor |
Staff |
Hospital |
time |
Term-topic probability (Φ tw) |
0.0923 |
0.2132 |
0.2488 |
0.3152 |
0.1247 |
Correlated value (c ) |
1 |
1 |
1 |
1 |
1 |
Sample terms modeled by CFS LDA |
Room |
Φ twC |
0.01340.0222 |
0.00040.1378 |
0.00050.2392 |
0.04710.0402 |
0.01340.0222 |
Disease |
Φ twC |
0.0004-0.0347 |
0.02220.0547 |
0.0005-0.0462 |
0.00040.0948 |
0.0004+0.0408 |
Appointment |
Φ twC |
0.0134-0.0343 |
0.00920.1802 |
0.0005-0.0462 |
0.0004-0.0414 |
0.00040.0477 |
Patient |
Φ twC |
0.01770.0042 |
0.00040.1415 |
0.12470.1429 |
0.00040.1502 |
0.00050.2238 |
Bill |
Φ twC |
0.00040.0468 |
0.0265-0.0015 |
0.00010.1614 |
0.01210.1176 |
0.00050.2111 |
Table 1.6shows the list of feature terms selected by the CFS LDAmodel. Among the pre-processed and PoS tagged nouns, 68 terms are selected for the topic “cost,” 110 for “medicare,” 112 for “staff,” 101 for “infrastructure,” and 73 for “time.”
Читать дальше