Artificial Intelligent Techniques for Wireless Communication and Networking
Здесь есть возможность читать онлайн «Artificial Intelligent Techniques for Wireless Communication and Networking» — ознакомительный отрывок электронной книги совершенно бесплатно, а после прочтения отрывка купить полную версию. В некоторых случаях можно слушать аудио, скачать через торрент в формате fb2 и присутствует краткое содержание. Жанр: unrecognised, на английском языке. Описание произведения, (предисловие) а так же отзывы посетителей доступны на портале библиотеки ЛибКат.
- Название:Artificial Intelligent Techniques for Wireless Communication and Networking
- Автор:
- Жанр:
- Год:неизвестен
- ISBN:нет данных
- Рейтинг книги:5 / 5. Голосов: 1
-
Избранное:Добавить в избранное
- Отзывы:
-
Ваша оценка:
- 100
- 1
- 2
- 3
- 4
- 5
Artificial Intelligent Techniques for Wireless Communication and Networking: краткое содержание, описание и аннотация
Предлагаем к чтению аннотацию, описание, краткое содержание или предисловие (зависит от того, что написал сам автор книги «Artificial Intelligent Techniques for Wireless Communication and Networking»). Если вы не нашли необходимую информацию о книге — напишите в комментариях, мы постараемся отыскать её.
The 20 chapters address AI principles and techniques used in wireless communication and networking and outline their benefit, function, and future role in the field.
Audience
Artificial Intelligent Techniques for Wireless Communication and Networking — читать онлайн ознакомительный отрывок
Ниже представлен текст книги, разбитый по страницам. Система сохранения места последней прочитанной страницы, позволяет с удобством читать онлайн бесплатно книгу «Artificial Intelligent Techniques for Wireless Communication and Networking», без необходимости каждый раз заново искать на чём Вы остановились. Поставьте закладку, и сможете в любой момент перейти на страницу, на которой закончили чтение.
Интервал:
Закладка:
– In Chapter 16, “Adaptive and Intelligent Opportunistic Routing Using Enhanced Feedback Mechanism,” V. Sharmila, Mandal K, Shankar Shalani and P. Ezhumalai discuss opportunistic routing of an intercepted packet to provide an effective wireless mesh network. Traditional opportunistic routing algorithms are being used to provide high-speed use batching of packets, which is a complex task. Therefore, an enhanced opportunistic feedback-based algorithm is proposed in this chapter in which individual packet forwarding uses a new route calculation in the proposed work that takes into consideration the cost of transmitting feedback and the capacity of the nodes to choose appropriate rates for monitoring operating conditions.
– In Chapter 17, “Enabling Artificial Intelligence and Cyber Security in Smart Manufacturing,” R. Satheesh Kumar, G. Keerthana, L. Murali, S. Chidambaranathan, C.D. Premkumar and R. Mahaveerakannan propose an efficient green manufacturing approach in SM systems with the aid of AI and cyber security frameworks. The proposed work employs a dual-stage artificial neural network (ANN) to find the design configuration of SM systems in industries. Then, for maintaining data confidentiality while communicating, the data are encrypted using the 3DES approach.
– In Chapter 18, “Deep Learning in 5G Networks,” G. Kavitha, P. Rupa Ezhil Arasi and G. Kalaimani discuss a 3D-CNN model combined with RNN model for analyzing and classifying the network traffic into three various classes, such as maximum, average and minimum traffic, which proves that the combined 3D-CNN and RNN model provides better classification of network traffic.
– In Chapter 19, “EIDR Umpiring Security Models for Wireless Sensor Networks,” A. Kathirvel, S. Navaneethan and M. Subramaniam provide an overview of WSNs with their classification, as well as comparisons between different routing algorithms and the proposed EIDR (enhanced intrusion detection and response). Soundness of proposed EIDR is tested using QualNet 5.0.
– In Chapter 20, “Artificial Intelligence in Wireless Communication,” Prashant Hemrajani, Vijaypal Singh Dhaka, Manoj Kumar Bohra and Amisha Kirti Gupta describe the applications of AI techniques in wireless communication technologies and networking, which can bring these changes through new research. Also, AI/ML techniques can improve the current state of network management, operations and automation. They can further support software-defined networking (SDN) and network function virtualization (NFV), which are considered important wireless communication technology components for the deployment of 5G and higher generation communication systems.
All of the contributors to this book deserve our heartfelt gratitude. With the fervent cooperation of the editorial director and production editor at Scrivener Publishing, we aspire to cross many more milestones to glory in the future academic year.
Prof. Dr. R. Kanthavel
Department of Computer Engineering, King Khalid UniversityAbha, Saudi Arabia
Dr. K. Ananthajothi
Department of Computer Science and Engineering Misrimal Navajee Munoth Jain Engineering CollegeChennai, India
Dr. S. Balamurugan
Founder and Chairman, Albert Einstein Engineering and Research Labs (AEER Labs)Vice-Chairman, Renewable Energy Society of India, India
Dr. R. Karthik Ganesh
Department of Computer Science and EngineeringSCAD College of Engineering and Technology Cheranmahadevi, India January 2022
1
Comprehensive and Self-Contained Introduction to Deep Reinforcement Learning
P. Anbalagan*, S. Saravanan and R. Saminathan
Department of Computer Science and Engineering, Annamalai University, Annamalai Nagar, India
Abstract
Deep reinforcement learning is a type of machine learning and artificial intelligence in which smart robots, similar to the way people make good decisions, can learn from their actions. Implicit in this form of machine learning is that, depending on their behavior, an agent is rewarded or punished. Including unsupervised machine learning and supervised learning, reinforcement learning is yet another common type of artificial intelligence development. Deep reinforcement learning can lead to incredibly impressive results beyond normal reinforcement learning, due to the fact that it incorporates the core qualities of both deep learning and reinforcement learning. Since this is becoming a very broad and rapidly growing field, the entire application landscape will not be explored, but mainly based on comprehensive and self contained introduction to deep reinforcement learning. The goal of this chapter is twofold: (i) to provide a brief guide to the deep reinforcement learning process; (ii) to present detailed applications and research directions.
Keywords:Artificial intelligence, deep learning, machine learning, reinforcement learning
1.1 Introduction
Due to its effectiveness in solving complex sequential decision-making issues, Reinforcement Learning (RL) has become increasingly common over the past few years. Many of these accomplishments are due to the integration of deep learning techniques with RL. But, thanks to its ability to learn various levels of abstractions from data, deep RL has been effective in complex tasks with lower prior knowledge. For example, from visual perceptual inputs made up of thousands of pixels, a deep RL agent can successfully learn [14]. Deep RL also has potential for real-world areas such as medical, self-driving cars, finance and smart grids, to name a few. Nonetheless in implementing deep RL algorithms, many problems arise. The area of machine learning that deals with sequential decision-making is reinforcement learning (RL) [16, 20].
As an agent who has to make decisions in an atmosphere to maximize a given definition of accumulated rewards, the RL problem can be formalized. It will become apparent that this formalization extends to a wide range of tasks and captures many important characteristics of artificial intelligence, such as a sense of cause and effect, as well as a sense of doubt and non-determinism [5].
A main feature of RL is that good behavior is taught by an agent. This suggests that it incrementally modifies or acquires new habits and abilities. Another significant feature of RL is that it uses experience of trial and error (as opposed to for example, dynamic programming that a priori assumes maximum environmental knowledge). Therefore the RL agent does not need full environmental awareness or control; it just needs to be able to communicate with the environment and gather information. The knowledge is gained a priori in an offline environment, then it is used as a batch for learning (the offline setting is therefore also called batch RL) [3].
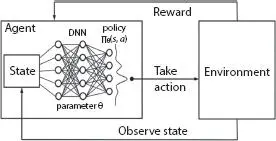
Figure 1.1Reinforcement learning process.
In comparison to the online world, this is where information becomes available in a linear order and is used to change the agent’s actions gradually. The core learning algorithms are exactly the same in both situations, but the key difference is that the agent will affect how it gathers experience in an online environment. This is an important difficulty, primarily because while studying, the agent has to deal with the problem of exploration/exploitation. But learning in the online world can also be a benefit, as the agent can collect data directly about the most important part of the environment. For that purpose, RL approaches may provide the most algorithmically efficient solution in practice, even when the context is fully understood, compared to other dynamic programming methods that would have been inefficient due to this lack of precision [8].
Читать дальшеИнтервал:
Закладка:
Похожие книги на «Artificial Intelligent Techniques for Wireless Communication and Networking»
Представляем Вашему вниманию похожие книги на «Artificial Intelligent Techniques for Wireless Communication and Networking» списком для выбора. Мы отобрали схожую по названию и смыслу литературу в надежде предоставить читателям больше вариантов отыскать новые, интересные, ещё непрочитанные произведения.
Обсуждение, отзывы о книге «Artificial Intelligent Techniques for Wireless Communication and Networking» и просто собственные мнения читателей. Оставьте ваши комментарии, напишите, что Вы думаете о произведении, его смысле или главных героях. Укажите что конкретно понравилось, а что нет, и почему Вы так считаете.