For a specific product, the detectability of flash flood events is better for finer temporal scale. The hit rate of CR6 and CR24 is lower, less than 60%. Most of the flash flood events captured by these three precipitation products are located in western Yunnan, while in the relatively flat southwest and central Yunnan, there are fewer flash flood outbreaks. In general, if a flash flood event cannot be captured in a short period, it will not be captured at a lower time scale. Compared with IMERG‐F, IMERG‐E is significantly worse at capturing flood events; IMERG‐E’s hit rate is lower than 50% on all time scales. Conversely, IMERG‐F showed considerable accuracy in capturing CR1 and CR3 of the CMA flood, with a difference of 1%. For instance, the hit rate of IMERG‐F for CR1 is about 80%. But the hit rate decreases significantly as the time scale decreases from 1h to 24h.
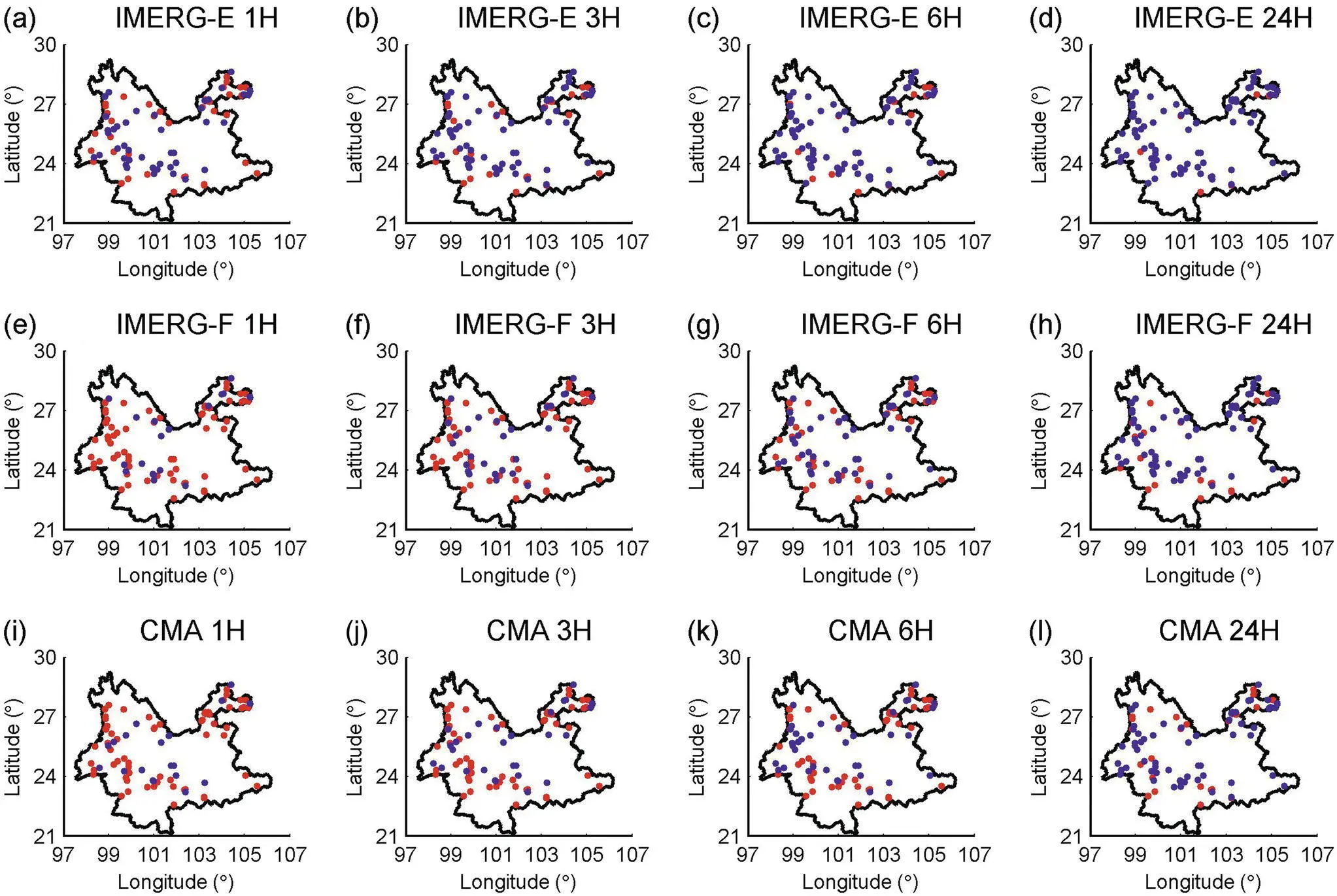
Figure 2.10 The performance of the flash floods captured by the three products (IMERG‐F, IMERG‐E, CMA) for different times (1 h, 3 h, 6 h, 24 h). Red indicates that the warning issued has captured the flash flood event; blue indicates that the issued warning has not captured the flash flood event.
Source: Based on Ma et al. (2020).
Figure 2.11 Percentage of flash floods caught by CMA, IMERG‐E, and IMERG‐F, based on Ma et al. (2020). Note: CRt represents the critical rainfall at time t.
Source: Based on Ma et al. (2020).
According to the accuracy in capturing flood events, CMA is better than IMERG‐F, followed by IMERG‐E. However, another important issue is the latency of those products. CMA is a merged product based on CMORPH and ground station data and thus has a nonnegligible latency time. IMERG‐F has a latency time of more than one month. IMERG‐E has a latency time of several hours. Currently, many studies use near‐real‐time satellite products such as IMERG‐E to monitor flood hazards. However, operational flood forecasting must rely on nowcast precipitation from weather models. Therefore, how to make those products useful in practical application is still challenging.
2.5. SUMMARY AND CONCLUSION
This study evaluates the performance of retrospective IMERG precipitation estimates from 2000 to 2018 at hourly and daily scales and compares it with nine satellite and reanalysis precipitation estimates in China. Various metrics and evaluation methods are employed. Special attention is paid to snowfall validation using an objective error analysis method. In addition, IMERG products are applied to capture flash flood hazards in a typical region, Yunnan Province. The conclusions are as below.
IMERG performs very well on the daily scale in the whole China and three subregions concerning all accuracy metrics. It is better than other satellite and reanalysis products, except for GSMaP. At the hourly scale, IMERG is also satisfying and exhibits better performance than previous versions through indirect comparison. PCDR and CHIRPS exhibit limited performance compared to microwave‐based or microwave‐infrared combined products. However, PCDR and CHIRPS are better at estimating precipitation during winter in TP, XJ, and NE. In contrast, CMORPH almost loses the capability of detecting precipitation occurrence for the same season and regions, indicating that infrared data are more useful than passive microwave data under a cold climate or over snowy/icy surfaces. SM2RAIN is the worst among all products. SM2RAIN performs relatively better in arid regions such as XJ and Inner Mongolia than the moister regions in south and east China because soil moisture is seldom saturated in an arid climate.
Regarding the flood warning in Yunnan Province, we find that (1) IMERG‐F presents acceptable accuracy over the study area with a relatively high hourly correlation coefficient of 0.46 and relative bias of 23.33% on the grid, while IMERG‐E shows worse performance as expected; (2) by applying the RTI method, CMA and IMERG‐F exhibit similar performance in capturing flood hazards, while IMERG‐E captures fewer floods than CMA and IMERG‐F. Besides, all products show better performance at the finer temporal scale. It should be noted that the large time lag of IMERG‐F prevents its real application and makes it suitable only in historical studies. The climatological correction of near‐real‐time satellite precipitation products (e.g., IMERG‐E) is an important research direction to make them more useful in flood monitoring.
Meanwhile, IMERG also needs improvement. For example, IMERG should consider a transfer from monthly‐scale gauge adjustment based on GPCC to daily‐scale adjustment because (1) the monthly‐scale adjustment has limited effect on metrics such as CC, RMSE, and CSI at the hourly scale, (2) IMERG_uncal shows similar performance with IMERG_cal in detecting precipitation occurrence at daily and hourly scales, and (3) GSMaP outperforms IMERG, benefiting from its daily‐scale gauge adjustment. Moreover, IMERG shows weak capability in estimating precipitation under cold climate (e.g., during winter and over high latitudes) due to the effect of snowfall, which could be improved through better rainfall‐snowfall classification or physically based retrieval algorithms. The trend of snowfall is most notable in TP, whereas different data sets show contradictory trends. The measurement or estimation of snowfall remains a challenging problem.
Abbreviation |
Full phrase |
CC |
Pearson correlation coefficient |
CGDPA |
Chinese Rain Gauge‐based Daily Precipitation Analysis |
CHIRPS |
Climate Hazards group Infrared Precipitation with Stations |
CMA |
China Meteorological Administration |
CMORPH |
Climate Prediction Center (CPC) MORPHing technique bias corrected (CRT) |
CPC |
Climate Prediction Center |
CR |
critical rainfall |
CSI |
Critical success index |
ERA‐Interim |
ECMWF ReAnalysis Interim |
ERA5 |
Fifth generation of ECMWF atmospheric reanalyses of the global climate |
FAR |
False alarm ratio |
FFG |
Flash Flood Guidance |
GPM |
Global Precipitation Measurement |
GSMaP |
Global Satellite Mapping of Precipitation |
GSMaP |
Gauge‐adjusted Global Satellite Mapping of Precipitation V6/V7 |
IMERG |
Integrated Multi‐satellitE Retrievals for Global Precipitation Measurement |
IMERG_cal |
IMERG calibrated precipitation |
IMERG_uncal |
IMERG uncalibrated precipitation |
IMERG‐E |
IMERG Early run |
IMERG‐F |
IMERG Final run |
KGE’ |
Kling‐Gupta efficiency |
ME |
Mean error |
MERRA2 |
The Modern‐Era Retrospective Analysis for Research and Applications, Version 2 |
MTC |
Multiplicative TC |
NE |
Northeastern region |
PCDR |
PERSIANN‐Climate Data Record |
PERSIANN |
Precipitation Estimation from Remotely Sensed Imagery Using Artificial Neural Networks |
PERSIANN‐CCS |
PERSIANN‐ Cloud Classification System |
POD |
Probability of detection |
RMSE |
Root mean square error |
RTI |
Rain Trigger Index |
SM2RAIN |
SM2RAIN based on ESA Climate Change Initiative (CCI) |
SMI |
Soil Moisture Index |
T3B42 |
TRMM Multi‐satellite Precipitation Analysis (TMPA) 3B42 V7 |
TC |
Triple collocation |
TMI |
TRMM microwave imager |
TMPA |
TRMM multi‐satellite precipitation analysis |
TP |
Qinghai‐Tibet Plateau |
XJ |
Xinjiang Province |
Читать дальше