Predicting Heart Failure
Здесь есть возможность читать онлайн «Predicting Heart Failure» — ознакомительный отрывок электронной книги совершенно бесплатно, а после прочтения отрывка купить полную версию. В некоторых случаях можно слушать аудио, скачать через торрент в формате fb2 и присутствует краткое содержание. Жанр: unrecognised, на английском языке. Описание произведения, (предисловие) а так же отзывы посетителей доступны на портале библиотеки ЛибКат.
- Название:Predicting Heart Failure
- Автор:
- Жанр:
- Год:неизвестен
- ISBN:нет данных
- Рейтинг книги:3 / 5. Голосов: 1
-
Избранное:Добавить в избранное
- Отзывы:
-
Ваша оценка:
- 60
- 1
- 2
- 3
- 4
- 5
Predicting Heart Failure: краткое содержание, описание и аннотация
Предлагаем к чтению аннотацию, описание, краткое содержание или предисловие (зависит от того, что написал сам автор книги «Predicting Heart Failure»). Если вы не нашли необходимую информацию о книге — напишите в комментариях, мы постараемся отыскать её.
Predicting Heart Failure: Invasive, Non-Invasive, Machine Learning and Artificial Intelligence Based Methods
Predicting Heart Failure
Predicting Heart Failure: Invasive, Non-Invasive, Machine Learning and Artificial Intelligence Based Methods
Predicting Heart Failure — читать онлайн ознакомительный отрывок
Ниже представлен текст книги, разбитый по страницам. Система сохранения места последней прочитанной страницы, позволяет с удобством читать онлайн бесплатно книгу «Predicting Heart Failure», без необходимости каждый раз заново искать на чём Вы остановились. Поставьте закладку, и сможете в любой момент перейти на страницу, на которой закончили чтение.
Интервал:
Закладка:
2.5.2 AI in Electrocardiography
Electrocardiography has a high impact in detecting abnormalities in heart rhythm. Most of the existing machine learning applications based on ECG focus on classification of electrical signals to spot abnormal activity in the heart. With today’s availability of ECG in small portable devices such as smartwatches, AI integration helps patients to monitor their heartbeat levels and detect any abnormalities that might require seeing a doctor or having further tests.
The work of Li et al. [28] applied a multichannel, multiscale deep neural network model to perform ECG classification of normal and abnormal signals, and their method achieved 96.02% accuracy. Other research work by Rajput el. [29] developed an automated ECG signal detection specifically for detecting the severity of hypertension, a high-risk factor for heart disease. Their method included using a two-band optimal biorthogonal wavelet bank filter as well as machine learning techniques to classify the wavelets into low, high-risk hypertension, and healthy. The average classification accuracy for this method was found to be 99.95%, and highlights the ability of this system to be deployed in clinics.
A method for ECG identification and detection of congestive heart failure (CHF) and arrhythmia (ARR) using deep learning techniques is that proposed by Eltrass et al. [30]. It mainly uses both CNN and constant-Q non-stationary Gabor transform (CQ-NSGT), which mainly transforms the 1D ECG signal to a 2D time-frequency representation. The output is then fed to a pretrained CNN model (AlexNet) to extract the features of these signals and then the features are used to diagnose the case by either classifying it as CHF, ARR, or normal sinus rhythm (NSR). The work achieved 98.82% accuracy, 98.87% sensitivity, 99.21% specificity, and 99.20% precision.
In the work by Moridani et al. [31], the authors experimented with multiple machine learning algorithms for ECG signal classification. They concentrated on performing preprocessing techniques for the initial signal and then applying feature extraction for both linear and nonlinear features for the heart rate variability as well as the application of statistical characteristics for the signal. Afterward, they used multilayer perceptron (MLP) and SVM, along with all features as well as optimal features to classify normal and abnormal signals in separate experiments. Their outcomes highlighted the performance of the SVM model that was able to get the highest accuracy while using all features and optimal features. For the optimal features, it achieved 98.3% accuracy, 99.10% sensitivity, and 97.5% specificity.
2.5.3 AI in CT
CT scans are mainly used to view parts of the body in detail. In the case of a cardiac CT scan, it displays the heart and the blood vessels clearly which helps experts to diagnose or detect any abnormality. CT scans can detect the early signs of heart disease by scanning the heart’s arteries for any calcified plaque formation to create a coronary artery calcium (CAC) score, which has been proven to be a strong predictor for CVDs (Figure 2.9).
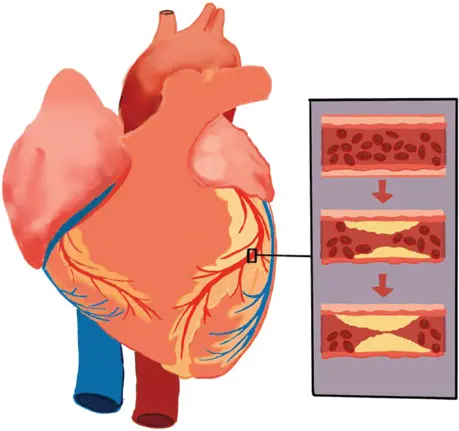
Figure 2.9 Plaque formation in the heart arteries.
The data captured by a CT scan generates a 3D model of a patient’s heart. Cardiac segmentation in chest CT images facilitates partitioning the entire chest CT image into numbers of anatomically significant regions that focus on the four chambers of the heart. The manual process of segmentation is becoming replaced by computer-aided techniques such as graph-based segmenting, mean-thresholding, fuzzy clustering methods, and the latest deep learning approach, which has shown promising results. The deep learning system can be trained to identify and quantify coronary artery calcium. This makes an effective early detection device for coronary calcification from atherosclerosis as it detects calcification before symptoms develop. This forms a powerful predictor of future heart problems.
One of the works proposed in the literature regarding CAC was about developing an algorithm that excludes CT scans with negative CAC scores and segments CAC in cases where positive scores are found. The segmented region can further proceed to radiologists for accurate detection. The proposed method highly reduces the workload of radiologists [32]. The proposed method utilized an integrated architecture model which consists of two CNNs where one is for classification and the other for segmentation. The model was able to exclude 86% of the negative cases and segmentation achieved a Dice coefficient of 0.63 and 0.84, for internal and external validation, respectively.
Another research group developed a deep learning model for CAC classification and segmentation [33]. The group focused on calcium quantification of chest CT scans and compared it to manual evaluation. The model was a combination of CNNs along with a ResNet architecture for image feature extraction, as well as an FCN for spatial coordinate features. Their method displayed a high correlation with the manual evaluation for detecting the presence of calcification, with results of 91% sensitivity and 92% specificity. In the case of calcium volume determination, the AI model was able to produce an excellent correlation with the manual determination with no significant difference found. The method proved to be fully automated, reducing the time for evaluation while optimizing clinical calcium scoring.
The work by Zhang et al. [34] utilized deep learning for calcium quantification from CT scan images. They developed a fully automated framework to detect CAC, using 3D CT scans as input for the model and extracting features to apply segmentation and calcification. The obtained results of the proposed method have no significant difference compared to the manual method.
Dekker et al. [35], implemented the deep learning technique to calculate the CAC score during myocardial perfusion imaging (MPI) assessment. They defined a threshold for CAC scores where it was low (<400) and high (≥400). The deep learning model used in the proposed method was previously validated in other approaches. The outcomes of the research work highlighted that high CAC scores presented higher accumulative event rates. They also proved that their method could progress risk stratification leading to customized treatments for patients.
In conclusion, AI methods showed better automation and higher accuracy in diagnosis in some applications. Some of the outcomes are even open to further enhancement which could lead to higher performance. This could help to reduce the effort of physicians and radiologists in diagnosing heart disease in the future. AI based methods can’t replace clinical methods such as ECG, EEG, or CT scans; however, they can automate the decision-making task.
2.6 Conclusion
Heart diseases are very common nowadays and are one of the major contributors of world mortality rate. The prompt diagnosis of heart disease can reduce the casualty as well as mortality associated with the risk of heart disease to a great extent. Today, due to the technological advancement in signal processing, medical imaging, sensors, etc., diagnosis of heart disease and finding the underlying specific reasons is not hard. Future versions of the medical devices, including the stethoscope and ECG machines, will utilize AI technologies for efficient detection of heart abnormalities in hospitals and clinics. However, conventional methods will also be used alongside advanced methods, with most of the former, including inspection and palpation, being cost-effective and not requiring any advanced devices or sensors. Recent advancements that enabled the development of neural network-based stethoscopes will reduce the effort of physicians to perform conventional auscultation examinations.
Читать дальшеИнтервал:
Закладка:
Похожие книги на «Predicting Heart Failure»
Представляем Вашему вниманию похожие книги на «Predicting Heart Failure» списком для выбора. Мы отобрали схожую по названию и смыслу литературу в надежде предоставить читателям больше вариантов отыскать новые, интересные, ещё непрочитанные произведения.
Обсуждение, отзывы о книге «Predicting Heart Failure» и просто собственные мнения читателей. Оставьте ваши комментарии, напишите, что Вы думаете о произведении, его смысле или главных героях. Укажите что конкретно понравилось, а что нет, и почему Вы так считаете.