Predicting Heart Failure
Здесь есть возможность читать онлайн «Predicting Heart Failure» — ознакомительный отрывок электронной книги совершенно бесплатно, а после прочтения отрывка купить полную версию. В некоторых случаях можно слушать аудио, скачать через торрент в формате fb2 и присутствует краткое содержание. Жанр: unrecognised, на английском языке. Описание произведения, (предисловие) а так же отзывы посетителей доступны на портале библиотеки ЛибКат.
- Название:Predicting Heart Failure
- Автор:
- Жанр:
- Год:неизвестен
- ISBN:нет данных
- Рейтинг книги:3 / 5. Голосов: 1
-
Избранное:Добавить в избранное
- Отзывы:
-
Ваша оценка:
- 60
- 1
- 2
- 3
- 4
- 5
Predicting Heart Failure: краткое содержание, описание и аннотация
Предлагаем к чтению аннотацию, описание, краткое содержание или предисловие (зависит от того, что написал сам автор книги «Predicting Heart Failure»). Если вы не нашли необходимую информацию о книге — напишите в комментариях, мы постараемся отыскать её.
Predicting Heart Failure: Invasive, Non-Invasive, Machine Learning and Artificial Intelligence Based Methods
Predicting Heart Failure
Predicting Heart Failure: Invasive, Non-Invasive, Machine Learning and Artificial Intelligence Based Methods
Predicting Heart Failure — читать онлайн ознакомительный отрывок
Ниже представлен текст книги, разбитый по страницам. Система сохранения места последней прочитанной страницы, позволяет с удобством читать онлайн бесплатно книгу «Predicting Heart Failure», без необходимости каждый раз заново искать на чём Вы остановились. Поставьте закладку, и сможете в любой момент перейти на страницу, на которой закончили чтение.
Интервал:
Закладка:
2.4.2 Electrocardiogram
The electrical activity of skeletal muscles was first noticed by Dr. Luigi Galvan, a physician in Italy, who pointed out that this activity could be recorded using electrical currents. In 1842, another physicist, Dr. Carlo Matteucci, found the electrical activity or responses for heartbeats in a frog. Several years later, a physiologist from London, Augustus Desiré Waller, showed the first human electrocardiogram, which used a capillary electrometer and electrodes placed on the chest and back. From this experiment, many demonstrations grew, including triphasic electric cardiac activity using improved capillary electrometers, followed by an improvement in refining electrometers by using five measured deflections in the electrocardiograph [18].
The electrocardiogram machine initially had three electrodes forming a triangle. Two were later removed due to the poor yielding. The machine contained cylinders of an electrolyte solution and patients placed their arms and one leg inside these cylinders [18]. Examiners continued to use this machine until a new design was introduced in 1928 which was much lighter than the previous design and had five electrodes. Further improvements consisting of 12 electrodes were then made, giving more accurate measurements.
Today, ECG systems are available in much smaller and portable forms, such as smartwatches, ECG patches, etc., which allow users to place their fingers on them to generate the ECG results within seconds.
2.4.3 Echocardiogram (Ultrasound Devices)
Echocardiogram devices were first introduced in 1950, when the first M-mode echocardiography was developed by two physicists in order to study mitral stenosis and its diagnosis. Their work inspired many cardiologists, as they carried out their work to develop Doppler, two-dimensional (2D), contrast, and transesophageal echocardiograms. The echocardiogram was inspired by stethoscopes, and actually enables users to observe what occurs underneath the human skin [19]. The general setup of the echocardiography method is given in Figure 2.7.
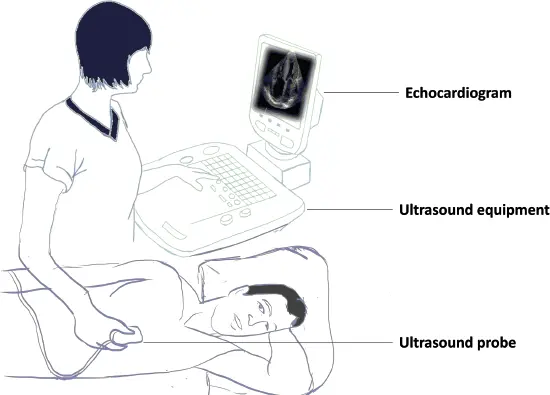
Figure 2.7 The echocardiography method. The patient lies in the left lateral decubitus position, and the ultrasound probe generates sound waves, receives an echo from body tissues, and sends the results to the ultrasound processing unit/computer to create a sonogram image.
The echocardiogram is an ultrasound of the heart that provides moving pictures capable of identifying the structure and function of the heart. It is more effective and efficient than ECG for detecting heart disease. ECG mainly provides information on the rhythm of the heart and involves a lengthy procedure compared to the an echocardiogram which involves a quick test that can be done in 5 minutes. An echocardiogram helps to visualize the heart beating and pumping blood, and aids the identification of heart disease. Providing information about heart functioning by measuring heart rate and rhythm, ECG is the preliminary test for detecting heart problems; any abnormality found here demands further diagnostic tests like an echocardiogram. Moreover, defects in heart valves are often undetected by ECG and the echocardiogram is the more preferred method for detecting valvular heart diseases. The echocardiogram is more efficient, but expensive compared to ECG.
2.5 Artificial Intelligence for Assisting Clinical Cardiac Examination
Artificial intelligence (AI) is the ability of the computer to make human-like decisions based on previous learning to solve problems, recognize an object, or respond to languages. Currently, AI is widely used in various sectors, such as business, agriculture, and health. In recent years, the various AI methods such as machine learning and deep learning have been predominantly used in the health sector in applications such as development assistive systems [20] and automated disease diagnosing systems for various chronic health disorders, such as cancer [21] and coronary heart disease [22].
In the case of cardiac examination, AI is mostly integrated for processing the physiological readings obtained from echocardiography, electrocardiography, and cardiac computed tomography (CT). Various image processing, signal processing, and computer vision algorithms are utilized in the literature to predict the cardiac condition using physiological values obtained in these three procedures.
2.5.1 AI in Echocardiography
The role of ultrasound devices is vital in the early diagnosis of cardiovascular diseases (CVDs). In addition, some of the risk factors of CVDs can be due to high blood pressure or high cholesterol, and one of the main causes of such diseases can be the buildup of inflammatory cells known as plaques, which occur in the arterial wall and result in both blood restriction to the heart and lower oxygen intake. This medical condition is known as atherosclerosis. Early prediction of a CVD disease might help in preventing the progression of atherosclerosis as well as possible heart failures. In Figure 2.8, it is shown that plaque formation occurs mostly in the common carotid artery and internal carotid artery.
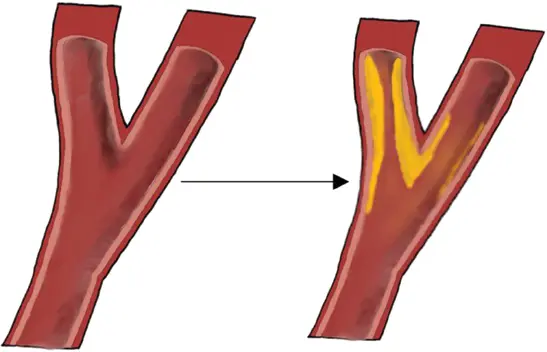
Figure 2.8 Formation of plaques in the common carotid artery and internal carotid artery.
One of the ways to identify the plaques in the arterial wall is by analyzing the carotid artery, which consists of a pair of blood vessels and has several parts, namely internal, external, and common parts. Plaques occur in the internal section as well as in the common blood vessels of the carotid artery. Hence, plaques create a thicker wall in these vessels, and can be measured as intima-media thickness (IMT). Thus, carotid IMT is used as a risk marker for early heart disease prediction. It can be done by measuring the difference between the lumen-intima (LI) and media adventitia (MA) walls [23].
Recently, many applications regarding ultrasound image segmentation using machine learning and deep learning techniques have been implemented to detect atherosclerosis. The work proposed in Nagaraj [24] used support vector machines (SVM) to train and segment IMT using a dataset of 49 images and resulted in 93% accuracy. Other researchers (see Biswas, Saba, et al. [25]) have worked on a screening tool that integrates a two-stage AI model for IMT and carotid plaque measurements, and consists of a convolutional neural network (CNN) and a fully convolutional network (FCN). The system goes through two deep learning models. The first divides the common carotid artery from the ultrasound images into two categories: the rectangular wall and non-wall patches. Then, the region of interest is analyzed and fed to the second stage, which identifies features in order to calculate the carotid IMT and the plaque total.
The work in Biswas, Kuppili, et al. [26] combined both the CNN algorithm and the machine learning based regression technique for IMT segmentation. Further, Menchón-Lara and colleagues [27] implemented an autoencoder model for segmenting IMT. Their method included regions of interest (ROI) prediction and then LI interface (LII) and MA interface (MAI) walls predictions in the predicted ROI. The authors reported that they used extreme learning machines along with autoencoders to distinguish between the block included in the ROI and the excluded from the ROI. The LII and MAI recognition was done using pixel classification.
Читать дальшеИнтервал:
Закладка:
Похожие книги на «Predicting Heart Failure»
Представляем Вашему вниманию похожие книги на «Predicting Heart Failure» списком для выбора. Мы отобрали схожую по названию и смыслу литературу в надежде предоставить читателям больше вариантов отыскать новые, интересные, ещё непрочитанные произведения.
Обсуждение, отзывы о книге «Predicting Heart Failure» и просто собственные мнения читателей. Оставьте ваши комментарии, напишите, что Вы думаете о произведении, его смысле или главных героях. Укажите что конкретно понравилось, а что нет, и почему Вы так считаете.