1 ...7 8 9 11 12 13 ...19
2.4 Algorithms
2.4.1 Linear Regression
Linear regression is an approach linear in nature to modeling the relationship connecting a scalar response and one or more explanatory variables. A prognostic modeling technique finds a relationship among independent variable and dependent variable. The independent variables can be categorical or continuous, while dependent variables are only continuous.
LASSO regression is another sort of linear regression; it makes use of shrinkage. Its data values are reduce in measurement in the route of a valuable point like mean. The system encourages easy and sparse models; the acronym “LASSO” is for the Least Absolute Shrinkage and choice Operator [4, 5]. L1 regularization is done with the aid of LASSO regression; it gives a sanction, which is equal to the absolute fee of the coefficients significance. This form of regularization outcomes in sparse fashions with much less coefficients; many coefficients can emerge as zero and are eliminated from the model. Huge penalties result in values close by to zero, which produces less difficult fashions. On the opposite, L2 regularization (e.g., ridge regression) does not bring about the exception of the coefficients or sparse models. This makes the LASSO higher to elucidate than the ridge.
A selection tree is flowchart-like tree, in which a characteristic is represented by using inner node; the choice rule is represented with the aid of a branch and final results by way of each leaf node. The pinnacle node in a choice tree is called as the root node. It partitions the tree in a recursive way, namely, recursive partitioning. The time complexity is a characteristic of the range of statistics and the variety of attributes in the given records. Choice trees handle facts with high dimensionality and accuracy [13, 14].
2.4.4 Support Vector Machine
Support vector machine is a curated reading system and is used for classification and retrospective problems. The support vector machine is very popular, as it produces remarkable accuracy with low calculation power. It is widely used in segregation problems. It has three types: targeted, unsupervised, and reinforced learning. A support vector machine is a selected separator that is officially defined by separating the hyperplane. With the provision of training data, the release of advanced hyperplane algorithm that separates new models is labeled.
2.4.5 Random Forest Regressor
The Random Forest is a pliable and easy-to-use machine that produces good results most of the time with less time spent on hyperparameter setting. It has gained popularity because of its simplicity and the fact that it is use for split and reverse functions. Random forests are an amalgam of predictable trees in such a way that each tree is based on random vector values sampled independently and with the same distribution of all the trees in the forest. The general deforestation error changes as the limit of the number of trees in the forest grows.
XGBoost is a powerful way to build lower back-up fashions. The validity of this assertion can be characterized to the information of its (XGBoost) work with its students. Motive work includes job loss and time to get used to. It offers with the difference among actual values and expected values, e.g., how the model effects are from actual values. The typical loss features in XGBoost for deferral issues are reg: linear and, in binary categories, reg: logistics. Regularization parameters are as follows: alpha, beta, and gamma.
While working with regression fashions, it is very important to pick out an appropriate evaluation metric. It also addresses loss function for regression; few of them are mentioned in Table 2.2. If the distinction between the loss fee and the predicted value is less, then the loss/errors feature could be small and it characterized that the model is most suitable.
Table 2.2 Different evaluation metrics.
Metric |
Description |
Formula |
Mean squared error (MSE) |
It is generally used in a regression function, to check how close the regression line to the dataset points is. |
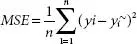 |
Root mean squared error (RMSE) |
It is often referred as root mean squared deviation. Its purpose is to find error in the numerical predictive models. |
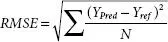 |
Mean absolute error (MAE) |
Similar to MSE, here, also, we take different between actual value and predicted value. |
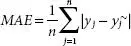 |
Coefficient of determination ( R 2) |
It is referred to as goodness of fit. The fraction of response/outcome is explained by the model. |
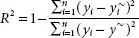 |
Pearson correlation coefficient |
It measures the strength of association between two variables. |
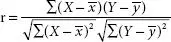 |
We can achieve RMSE just by taking square root of MSE. RMSE is very accessible with numerical prediction, to come across if any outliers are messing with the records prediction. Therefore, we select RMSE for version evaluation.
The dataset is divided into 80% of the training dataset and 20% of the testing dataset as seen in Table 2.3. The desired libraries were imported and GridSearchCV used to locate the satisfactory model. It compares the model on multiple regresses and different parameters and offers the best score among them. With the assistance of GridSearchCV, we have compared the algorithms, i.e., linear regression, LASSO regression, decision tree, support vector machine, random forest regressor, and XGBoost.
To aid this challenge, various device mastering algorithms are checked. It has been clear that XGBoost acts better with 85% accuracy and with an awful lot less blunders values. While this test is compared to the result, once those algorithms predicts properly. This task has been finished with the number one aim to determine the prediction for prices, which we have got efficiently completed using specific system analyzing algorithms like a linear regression, LASSO regression, decision tree, random forest, more than one regression, guide vector gadget, gradient boosted trees, neural networks, and bagging.
Consequently, it is clear that the XGBoost gives more accuracy in prediction in comparison to the others, and additionally, our research offers to locate the attributes contribution in prediction. In addition, python flask can be used as an http server and CSS/Html for creating UI for internet site. Therefore, one might agree with this that studies may be useful for the people and governments, and the future works are stated under every system, and new software program technology can assist in the future to expect the costs. Price prediction can be advanced by way of including many attributes like surroundings, marketplaces, and many different related variables to the houses.
Читать дальше