Interactive Online Learning Environment and Test Bank
We’ve worked hard to provide some really great tools to help you with your certification process. The interactive online learning environment that accompanies the AWS Certified Machine Learning Study Guide: Specialty (MLS-C01) Exam provides a test bank with study tools to help you prepare for the certification exam—and increase your chances of passing it the first time! The test bank includes the following:
Sample Tests:All the questions in this book are provided, including the assessment test at the end of this introduction and the review questions at the end of each chapter. In addition, there is a practice exam with 76 questions. Use these questions to test your knowledge of the study guide material. The online test bank runs on multiple devices.
Flashcards:The online text bank includes flashcards specifically written to challenge you, so don’t get discouraged if you don’t ace your way through them at first. They’re there to ensure that you’re really ready for the exam. And no worries—armed with the book, reference material, review questions, practice exams, and flashcards, you’ll be more than prepared when exam day comes. Questions are provided in digital flashcard format (a question followed by a single correct answer). You can use the flashcards to reinforce your learning and provide last-minute test prep before the exam.
Glossary:A glossary of key terms from this book is available as a fully searchable PDF.
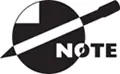
Go to www.wiley.com/go/sybextestprep
, register your book to receive your unique PIN, and then once you have the PIN, return to www.wiley.com/go/sybextestprep
and register a new account or add this book to an existing account.
Conventions Used in This Book
This book uses certain typographic styles in order to help you quickly identify important information and to avoid confusion over the meaning of words such as on-screen prompts. In particular, look for the following styles:
Italicized text indicates key terms that are described at length for the first time in a chapter. (Italics are also used for emphasis.)
A monospaced font indicates the contents of configuration files, messages displayed at a text-mode Linux shell prompt, filenames, text-mode command names, and Internet URLs.
Italicized monospaced text indicates a variable—information that differs from one system or command run to another, such as the name of a client computer or a process ID number.
Bold monospaced text is information that you're to type into the computer, such as at a shell prompt. This text can also be italicized to indicate that you should substitute an appropriate value for your system.
In addition to these text conventions, which can apply to individual words or entire paragraphs, a few conventions highlight segments of text:
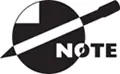
A note indicates information that's useful or interesting but that's somewhat peripheral to the main text. A note might be relevant to a small number of networks, for instance, or it may refer to an outdated feature.

A tip provides information that can save you time or frustration and that may not be entirely obvious. A tip might describe how to get around a limitation or how to use a feature to perform an unusual task.
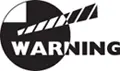
Warnings describe potential pitfalls or dangers. If you fail to heed a warning, you may end up spending a lot of time recovering from a bug, or you may even end up restoring your entire system from scratch.
Real World Scenario
Real-World Scenario
A real-world scenario is a type of sidebar that describes a task or an example that's particularly grounded in the real world. This may be a situation we or somebody we know has encountered, or it may be advice on how to work around problems that are common in real, working ML environments.
AWS Certified Machine Learning Specialty Exam Objectives
AWS Certified Machine Learning Study Guide has been written to cover every AWS exam objective at a level appropriate to its exam weighting. The following table provides a breakdown of this book's exam coverage, showing you the weight of each section and the chapter where each objective or subobjective is covered:
Subject Area |
% of Exam |
Domain 1: Data Engineering Domain 2: Exploratory Data Analysis Domain 3: Modeling Domain 4: Machine Learning Implementation and Operations |
20% 24% 36% 20% |
Total |
100% |
Domain 1: Data Engineering
Subdomain 1.1: Create Data Repositories for Machine Learning
Exam Objective |
Chapter |
1.1-1. Create data repositories for machine learning |
5 |
Identify data sources |
5 |
Determine storage mediums |
2, 5 |
Subdomain 1.2: Identify and Implement a Data Ingestion Solution
Exam Objective |
Chapter |
1.2-1. Data job styles/types (batch load/streaming) |
6, 7 |
1.2-2. Data ingestion pipelines |
7 |
Kinesis |
7 |
Kinesis Analytics |
7 |
Kinesis Firehose |
7 |
EMR |
7 |
Glue |
7 |
1.2-3. Job scheduling |
7, 15 |
Subdomain 1.3: Identify and Implement a Data Transformation Solution
Exam Objective |
Chapter |
1.3-1. Transforming data transit (ETL: Glue, EMR, AWS Batch) |
6 |
1.3-2. Handle ML-specific data using map reduce (Hadoop, Spark, Hive) |
6, 7 |
Domain 2: Exploratory Data Analysis
Subdomain 2.1: Sanitize and Prepare Data for Modeling
Exam Objective |
Chapter |
2.1-1. Identify and handle missing data, corrupt data, stop words, etc. |
6 |
2.1-2. Formatting, normalizing, augmenting, and scaling data |
6 |
2.1-3. Labeled data (recognizing when you have enough labeled data and identifying mitigation strategies [Data labeling tools (Mechanical Turk, manual labor)]) |
1, 5, 6 |
Subdomain 2.2: Perform Feature Engineering
Exam Objective |
Chapter |
2.2-1. Identify and extract features from datasets, including from data sources such as text, speech, image, public datasets, etc. |
7 |
2.2-2. Analyze/evaluate feature engineering concepts (binning, tokenization, outliers, synthetic features, One-hot encoding, reducing dimensionality of data) |
7 |
Subdomain 2.3: Analyze and Visualize Data for Machine Learning
Читать дальше