Various data mining tools are available for researchers and organizations. We will discuss the hands-on process of installing three major tools, namely Python, KNIME, and Rapid Miner [19–25].
1.7.1 Python for Data Mining
We will discuss Python for data mining in this last section with various techniques. Regression is a technique to reduce errors by estimating the relationship that may exist between variables. It is also possible to form clusters in Python. One can implement this regression method using Python as follows:
User can develop a regression model for given variables and helps researchers, students to estimate the relationship exists between them. It also helps in classifying the given objects, analyze the clusters formed, etc., using tools provided in Python [24].
Panda,” a library supported by Python, helps to clean and process the input data.
NumPy—a package supported by Python to perform computations.
Matplotlib—once the data is processed, there is a need to visualize this data, and it is possible using this package supported by Python.
Scikit-learn—a library supported by Python to model the data.
Python used in data mining, and machine learning executes the following steps:
1 Import the required libraries
2 Dataset loading (import)
3 If the dataset consists of missing data, then it must handle this missing data
4 Classifying or handling categorical data
5 Dividing the dataset into training and testing dataset
6 Features scaling (actually, it is a transformation of variables).
Installation and Setup of Python
1) Click on the link below and select OS: https://www.anaconda.com/download/[24]
2) Download Python 3.7 version (around 500 MB)
3) Once installed, launch the Anaconda Navigator (search by clicking the windows button)
4) Run the required Application (Jupyter, Spyder, etc.)
Make sure you constantly update the entire Anaconda distribution as it takes care of updating all the modules and dependencies inside (For more on installation, go to https://docs.anaconda.com/anaconda/install/windows/for Windows version).
Features of KNIME: KNIME [25] is an open-source analytical platform for data science. It helps to understand and design data science workflows, understanding time-series data analysis, to build machine learning models, and understand the data using visualization tools (charts, plots, etc.). It also helps to export the reports generated. KNIME workbench consists of KNIME explorer, Workflow bench, Node Repository, Workflow Editor, Description, Outline, and Console . It supports the data wrangling technique where one can collect and process the data from any source. It comes in two flavors:
◦ KNIME analytical platform
◦ KNIME server.
Both these platforms are available in Microsoft Azure and Amazon AWS
KNIME TOOL Installation
You can download the installer from the KNIME website. Once you successfully download it start the installation as specified in the next diagrams ( Figure 1.5). Every installation requires you must accept the agreement, click on the button and accept the agreement ( Figure 1.6). Installation requires specifying the path for installing the software, and as shown in the above diagram, it is a default path. If you wish, you can change the path by clicking on the “Browse” ( Figures 1.7and 1.8).
Figure 1.5 Installation of KNIME.
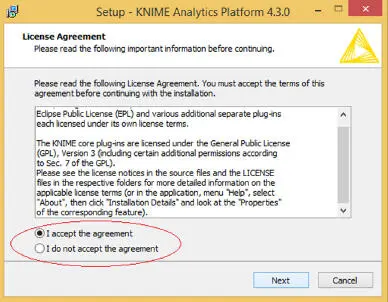
Figure 1.6 Installation of KNIME (2).
Figure 1.7 Setting path for installing KNIME.
Figure 1.8 Starting installation of KNIME.
Figures 1.9– 1.16show the complete workflow for selecting a Workspace path, and if you want to change the way, you can change it by clicking on the “Browse.” Finally, Figure 1.16gives you the home screen for mining purpose.
Figure 1.9 Selecting directory as a workspace.
Figure 1.10Starting KNIME.
Figure 1.11Completing setup wizard.
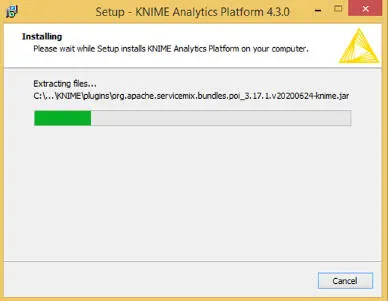
Figure 1.12Installing Workspace in KNIME.
Figure 1.13Installing KNIME (2).
Figure 1.14Specifying memory for KNIME.
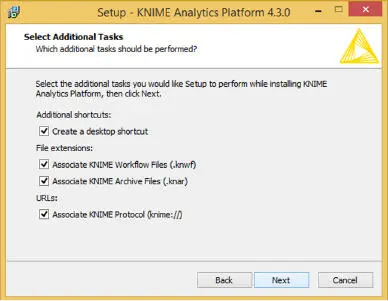
Figure 1.15Finalizing the installation of KNIME.
Figure 1.16 Initial screen of KNIME.
One can visit https://rapidminer.com/products/studio/for further instructions to download this tool. Its main features are as follows speedy creation of predictive models; Rich set of libraries to build the model like Bayesian modeling, Regression, Clustering, Neural networks, Decision trees . A rapid miner comes with templates, which are provided for guidance. One can use any data source like MS-excel, Access, CSV, NoSQL, MongoDB, Microsoft SQL Server, MySQL, Cassandra, PDF, HTML, XML . Rapid Miner Supports ETL (extract–transform–load), multiple file types, and Data exploration using exact statistical analysis. The Code control & management module is responsible for Background process execution, Automatic optimization, Scripting, Macros, Logging, Process control, and Process-based reporting. One can obtain good visualization using Scatter, scatter matrices, Line, Bubble, Parallel, Deviation, Box, 3-D, Density, Histograms, Area, Bar charts, stacked bars, Pie charts, Survey plots, Self-organizing maps, Andrews curves, Quartile, Surface/contour plots, time series plots, Pareto/lift chart. And finally, One can validate the designed model before deployment through Split validation, Bootstrapping, Batch cross-validation, Wrapper cross-validation, Lift chart, and Confusion matrix [24].
Читать дальше