Figure 1.22 Classification result by applying NB technique.
Case-V (NoF = 20)
Figure 1.23 Classification result by applying LR technique.
Figure 1.24Classification result by applying NN technique.
Figure 1.25Classification result by applying kNN technique.
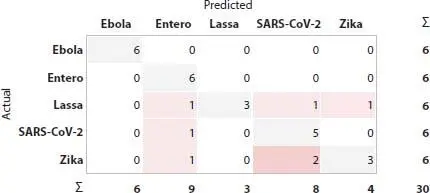
Figure 1.26 Classification result by applying NB technique.
From the analysis of Figures 1.7– 1.26and Table 1.1, it is observed that NB technique provides better classification results with CA values 0.667, 0.733 and 0.767 as compared to LR, NN and kNN when the NoF is 2, 10 and 20 respectively. Again, NB and kNN techniques provide better classification results with CA value 0.633 as compared to LR and NN technique when the NoF is 3. Similarly, NB and NN techniques provide better classification results with CA value 0.667 as compared to other techniques when the NoF is 5. Here, the maximum CA value is 0.767 which is provided by NB technique when the NoF is 20. So, CA value varies for each technique when different NoF are considered. However, NB technique attempts to provide better classification results in three different cases such as cases-I, -IV, and -V. In case-II, NB and kNN provide same CA values as 0.633 and in case-III, NB and NN provide same CA values as 0.667. However, the number of instances present in different cells of confusion matrix varies for the classification results of NB and kNN in case-II as well as NB and NN in case-III. So, NB technique is able to provide better classification results overall as compared to other technique.
Table 1.1 CA (in unit) of different classification techniques.
Method |
NoF = 2 |
NoF = 3 |
NoF = 5 |
NoF = 10 |
NoF = 20 |
LR |
0.567 |
0.533 |
0.633 |
0.567 |
0.600 |
NN |
0.567 |
0.567 |
0.667 |
0.667 |
0.667 |
kNN |
0.467 |
0.633 |
0.600 |
0.633 |
0.633 |
NB |
0.667 |
0.633 |
0.667 |
0.733 |
0.767 |
This chapter focuses on the processing of several TEMVIs such as EV, ENV, LV, SARS-CoV-2 and ZV using ML-based approach. The TEMVIs are analyzed by applying ML-based classification techniques such as LR, NN, kNN and NB. Each technique carries out the classification mechanism on several TEMVIs. From the analysis of results, it is concluded that the CA values changes for each classification technique when the NoF changes. The maximum CA value is provided by NB technique when the NoF is considered as 20. The NB technique provides overall better classification results as compared to LR, NN and kNN techniques by considering different NoF. This work will be extended to analyze the performance of these ML-based classification techniques along with other classification techniques by focusing on other types of TEMVIs as well as coronavirus dis-ease-19 (COVID-19) images in future.
1. Ray, U., Chouhan, U., Verma, N., Comparative study of machine learning approaches for classification and prediction of selective caspase-3 antagonist for Zika virus drugs. Neural Comput. Appl., 32, 11311–11328, 2020.
2. Singh, J.P., Pradhan, C., Das, S.C., Image Processing and Machine Learning Techniques to Detect and Classify Paddy Leaf Diseases: A Review, in: Machine Learning and Information Processing, pp. 161–172, Springer, Singapore, 2020.
3. Cao, Z., Identification of the Association between Hepatitis B Virus and Liver Cancer using Machine Learning Approaches based on Amino Acid, in: Proceedings of the 2020 10th International Conference on Bioscience, Biochemistry and Bioinformatics , 2020, January, pp. 56–63.
4. Sambasivam, G. and Opiyo, G.D., A predictive machine learning application in agriculture: Cassava disease detection and classification with imbalanced dataset using convolutional neural networks. Egypt. Inform. J. , 2020.
5. Al-Kasassbeh, M., Mohammed, S., Alauthman, M., Almomani, A., Feature Selection Using a Machine Learning to Classify a Malware, in: Handbook of Computer Networks and Cyber Security , pp. 889–904, Springer, Cham, 2020.
6. Yang, X., Yang, S., Li, Q., Wuchty, S., Zhang, Z., Prediction of human-virus protein–protein interactions through a sequence embedding-based machine learning method. Comput. Struct. Biotechnol. J., 18, 153–161, 2020.
7. Dey, L., Chakraborty, S., Mukhopadhyay, A., Machine Learning Techniques for Sequence-based Prediction of Viral–Host Interactions between SARS-CoV-2 and Human Proteins, Biomedical J., 2020.
8. Gibert, D., Mateu, C., Planes, J., The rise of machine learning for detection and classification of malware: Research developments, trends and challenges. J. Netw. Comput. Appl., 153, 1–22, 2020.
9. Karanja, E.M., Masupe, S., Jeffrey, M.G., Analysis of internet of things malware using image texture features and machine learning techniques. Internet Things, 9, 100153, 2020.
10. Sen, P.C., Hajra, M., Ghosh, M., Supervised Classification Algorithms in Machine Learning: A Survey and Review, in: Emerging Technology in Modelling and Graphics, pp. 99–111, Springer, Singapore, 2020.
11. Ahuja, R., Chug, A., Gupta, S., Ahuja, P., Kohli, S., Classification and Clustering Algorithms of Machine Learning with their Applications, in: Nature-Inspired Computation in Data Mining and Machine Learning , pp. 225–248, Springer, Cham, 2020.
12. Di Noia, A., Martino, A., Montanari, P., Rizzi, A., Supervised machine learning techniques and genetic optimization for occupational diseases risk prediction. Soft Comput., 24, 6, 4393–4406, 2020.
13. Firdausi, I., Erwin, A., Nugroho, A.S., Analysis of machine learning techniques used in behavior-based malware detection, in: 2010 Second International Conference on Advances in Computing, Control, and Telecommunication Technologies , 2010, December, IEEE, pp. 201–203.
14. Muda, Z., Yassin, W., Sulaiman, M.N., Udzir, N.I., Intrusion detection based on K-Means clustering and Naïve Bayes classification, in: 2011 7th International Conference on Information Technology in Asia , 2011, July, IEEE, pp. 1–6.
15. Chen, Y., Luo, Y., Huang, W., Hu, D., Zheng, R.Q., Cong, S.Z., Wang, X.Y., Machine-learning-based classification of real-time tissue elastography for hepatic fibrosis in patients with chronic hepatitis B. Comput. Biol. Med. , 89, 18–23, 2017.
16. Shruthi, U., Nagaveni, V., Raghavendra, B.K., A review on machine learning classification techniques for plant disease detection, in: 2019 5th International Conference on Advanced Computing & Communication Systems (ICACCS) , 2019, March, IEEE, pp. 281–284.
Читать дальше