2.2 Specific Advantages of Agent‐Based Modeling and Microsimulation Modeling
Agent‐Based Modeling
Agent‐based modeling (ABM) offers four specific advantages for public health research. First, because each actor in the system under study can be explicitly represented, no aggregation or statistical summary is required in treatment of either individual characteristics or outcomes. As a result, ABM is a powerful tool for considering heterogeneity—whether in biology, cognition, demography, or context. This is especially important for topics such as health disparities (Kaplan et al. 2017). Second, ABM offers an effective way to consider adaptation—processes of learning, evolution, or bidirectional interaction between individuals over time. This means that not only can we consider short‐run impacts of policies or interventions, but we can also explore potential impacts over very long time horizons. Topics such as obesity, antibiotic resistance, and developmental origins of health and disease often benefit from such considerations. Third, ABM is able to incorporate very sophisticated representations of structure and space, including social network data, physical space data from geographic information systems (GISs) or light detection and ranging (LIDAR), and biological space (e.g. physiology). Rather than either assuming away spatial elements or reducing them to summary statistics (for example, zip code‐level density of retailers), agent‐based models can carry a full accounting of spatial exposure and interaction throughout the dynamic simulation. Recent efforts to consider “precision prevention” in communities (Gillman and Hammond 2016; Economos and Hammond 2017) and retailer‐oriented tobacco control policies (Luke et al. 2017) leverage this facility of ABM. Finally, ABM is well suited for multilevel modeling. Each individual agent can contain detailed representations of “below‐the‐skin” processes such as energy balance, cognition, decision‐making, or disease progression; at the same time, the agents can interact with each other, with physical environments, and with population‐level signals (Hammond 2009; Hammond and Ornstein 2014). Although arguably essential to full understanding of many chronic disease challenges, crossing the “skin barrier” remains rare in social epidemiology.
Microsimulation models (MSM) enable simulations of policies on samples of economic agents (individual, households, and firms) at the individual level (Bourguignon and Spadaro 2006). These simulations allow for the projection of the consequences of modifying economic conditions for each individual agent in the sample. Through such projections, we can estimate the overall aggregate impacts of a policy as well as the distributional consequences of the policy in terms of “winners” and “losers.” These could be population subgroups as defined by social axes including age, gender, race/ethnicity, and socioeconomic status. Such policies may be expensive and not readily feasible to undertake in the real world. For example, changing the income tax structure can alter what absolute income individuals in a population receive and influence the distribution of income (i.e. levels of income inequality) within the population. Through tax microsimulation, we can hence project the absolute and relative income impacts without actually implementing these changes in the real world. MSM can thus offer a convenient and inexpensive means to estimate the overall population impacts of social policies.
Other Complex Systems Modeling Tools
Other key systems science approaches (not reviewed in this book) include system dynamics models and social network analysis (SNA). System dynamics models differ from ABM and MSM by aggregating factors and their interactions within endogenous systems to better understand high‐level phenomena such as the impacts of interventions and policies and their unintended consequences (Homer and Hirsch 2006). SNA studies the relationships between actors and entities—be they individuals, organizations, or countries. Like ABM, SNA can be useful in telescoping between the micro (individual) and the macroscales of analysis; yet unlike ABM, SNA does not always include dynamic simulation nor account for adaptation. Some forms of SNA overlap with ABM. SNA is widely used for understanding the transmission of infectious diseases such as HIV/AIDS and influenza, and the contagion of behaviors such as obesity and depression, since each of these can be transmitted socially (Christakis and Fowler 2007).
2.3 Comparison of Agent‐Based and Microsimulation Models
Figure 2.1illustrates some key differences between ABM, MSM, and statistical models (e.g. regression) as commonly used in population health. Unlike traditional models which draw on existing observational data, system science approaches such as ABM and MSM conduct ex ante assessments—for example, to consider the potential effects of policy interventions for which no data yet exist (and which therefore cannot easily be addressed by linear regression). In doing so, they leverage an ability to account for dynamic histories of individual agents, thereby incorporating changes in exposures over time, and to account for heterogeneous actors and behavioral responses. Behavioral responses include changes in the behaviors of agents in response to a new economic policy (e.g. tax policy) that imposes changes in individuals' budget constraints. Microsimulation is particularly well suited for studying the impacts of economic policies, including tax and welfare policies. Meanwhile, neither ABM nor MSM are specifically designed to enhance causal inference—such as by removing endogeneity—unlike advanced epidemiologic methods such as marginal structural regression and inverse probability weighting approaches that have been developed in recent years (Hernan and Robins 2010).
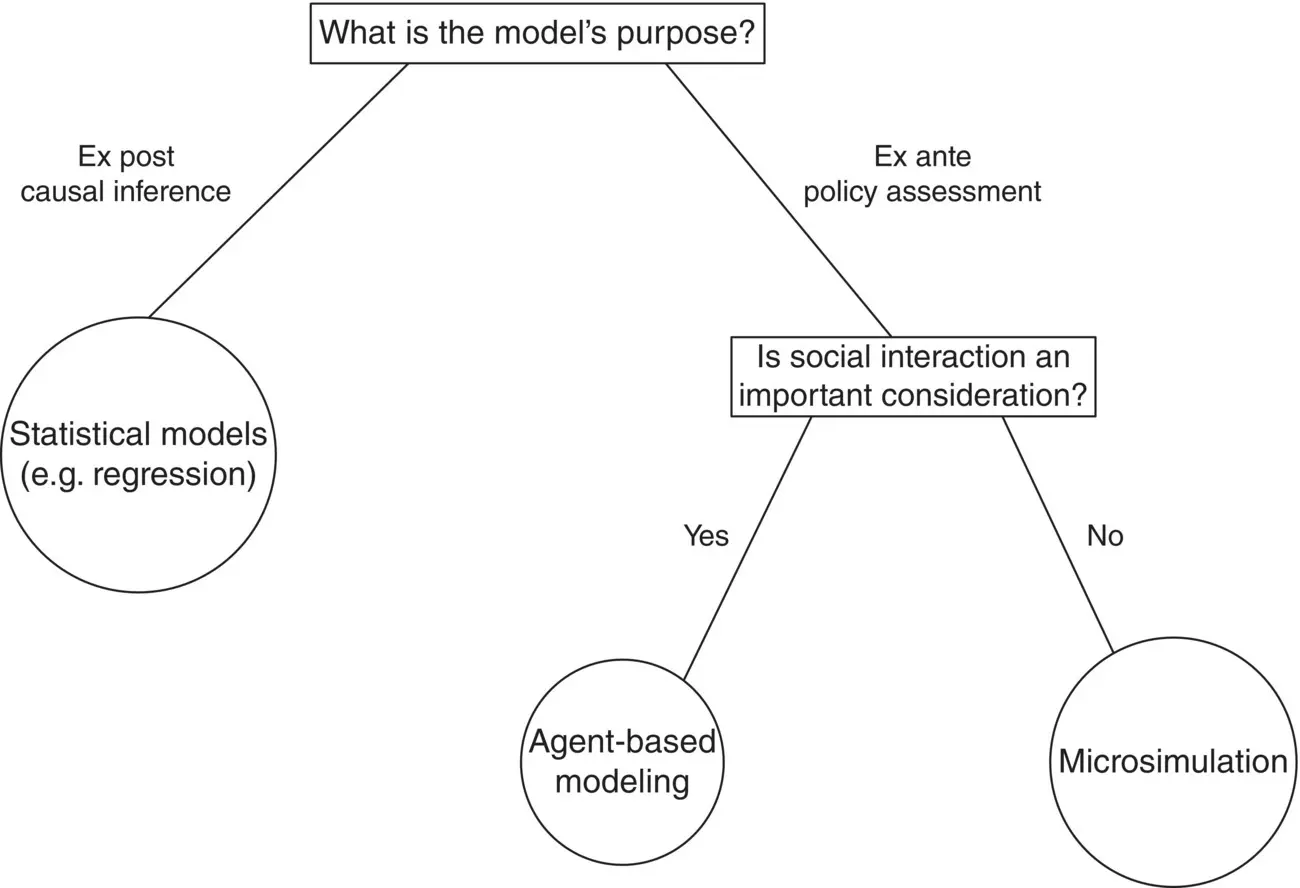
Figure 2.1 Key differences between agent‐based modeling, microsimulation modeling, and traditional statistical models.
An important distinction between ABM and MSM as commonly used in population health and social science is that MSM generally do not include any characterization of social interactions between individuals (except indirectly via a social‐level variable). By contrast, ABM models are generally focused on such interactions. Hence, MSM might be best suited for, say, consideration of tax policy, whereas ABM might be better suited for studying contagion of infectious disease.
2.4 Why ABM and MSM are Useful for Studying the Social Determinants of Health
In both of the fields of social epidemiology and social policy, understanding the nature of these relationships (such as the effect of a particular social determinant on health) using traditional models is greatly limited by the lack of consideration of the complexity of systems. In order to delineate the true effects of the social determinants of health within the complex systems of entire societies—characterized by multiple agents, nonlinearities, and complex feedback loops—novel modeling and simulation tools such as ABM and MSM are often required. For example, simulation studies can model the intergenerational transmission of socioeconomic disadvantage, an inquiry that is impractical in more traditional studies. Importantly, systems science approaches such as ABM and MSM can enable exploration of the possible impacts of policy options before actually implementing them (Maglio and Mabry 2011), which can avoid the ethical and feasibility issues that can arise from implementing interventions in real life. For example, in the review of the evidence‐based interventions for the social determinants of health by Bambra et al. (2010) described in the last chapter, no intervention studies on income inequality were found. Through MSM, we can readily simulate the potential health effects of a tax policy that modifies the income distribution within a population.
Читать дальше