2.4.3.1 Supervised Learning
This learning method requires a supervisor that tells the system what is the expected output for each input. Then, the machine learns from this knowledge. Specifically, the learning algorithm is given labeled data and the corresponding output. The machine learns a function that maps a given input to an appropriate output. For example, if we provide the ML system during the training phase with different pictures of cars, and with information indicating that these are pictures of cars, it will be able to build a model that can distinguish the cars’ pictures from any other pictures. The quality of a supervised model depends on the difference between the predicted output and the exact output. The convergence speed of supervised learning is high although it requires large amount of labeled data [6]. Next, we discuss the well-known supervised learning algorithms.
Figure 2.2Machine learning types.
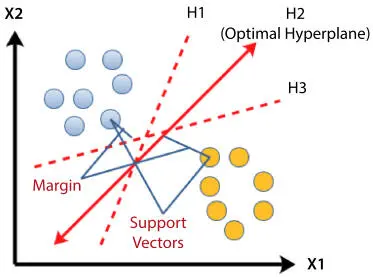
Figure 2.3Illustration of SVM.
Support Vector Machine
Support Vector Machine (SVM) algorithm is a linear supervised binary classifier. It separates data points using a hyperplane. The best hyperplane is the one which results in maximum separation between the two given classes. It is called the maximum margin hyperplane. SVM is considered to be a stable algorithm applied for binary classification. For multiple classification problems, the classification tasks must be reduced to multiple binary classification problems. The basic principle of SVM is illustrated in Figure 2.3.
K-Nearest Neighbors
A non-parametric learning algorithm used for classification and regression. The algorithm does not require any assumption on the data distribution. The objective of KNN is to decide the class of a data point based on the results of majority voting of its K-nearest neighbors (KNNs). The idea is that a data point is identified to belong to a certain class if KNNs belong to that class. A weight can be used for each neighbor that is proportional to the inverse of its distance to the data point in the classification process. KNN is easy to implement, not sensitive to outliers, highly accurate, and easily calculates features. It is also suitable for multi-class classification applications. The basic principle of KNN is illustrated in Figure 2.4.
2.4.3.2 Unsupervised Learning
In this technique, data is submitted to the learning algorithm without predefined knowledge or labels. Thus, the machine has to learn the properties of the dataset by itself through the study of unlabeled training data. The algorithm shall be able to define patterns from the input data. Observations are clustered in groups according to the similarities between them. The clustering algorithm examines the similarity of observations based on their features.
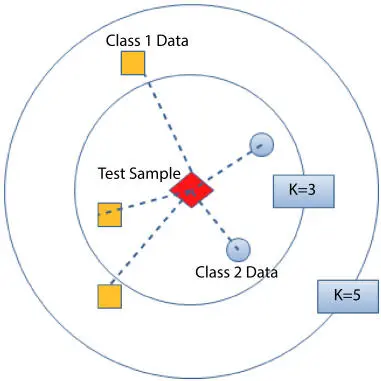
Figure 2.4Illustration of KNN.
Observations are then grouped in a way that puts elements that share a high similarity in the same group. Normally, algorithms use distance functions to measure similarities of observations. With Unsupervised learning, no prior knowledge is required. However, this comes at the cost of reduced accuracy [6].
The most commonly known unsupervised algorithm is clustering. Clustering algorithms divide data samples into several categories, called clusters. Clustering algorithms are of four main types [7]:
Centroid-Based Clustering: Clusters are defined using centroids. Centroids are data points that represent the proto-element of each group. The number of clusters has to be defined beforehand and is fixed. In the beginning, cluster centroids are defined randomly and will be shifted in the state space iteratively until the specified distance function is minimized.
K-Means Clustering is a simple and most common centroid-based method. The objective is to partition data points into K clusters, where each data point should belong to the cluster with the nearest mean. Initially, K mean points are randomly picked (the centroids). Then, the algorithm iterates on each data point and computes the distance to the centroids. The data point is judged to belong to the point to which the computed Euclidean distance is minimum. Thus, the method minimizes the distance between points and their corresponding centroids. Centroids are updated based on their assigned data points. The process continues until the centroids do not change. Figure 2.5 illustrates the concept of K-means clustering.
Hierarchical Clustering: In this type, the number of clusters is not defined a priori; rather, it is iteratively increases or decreases. In the beginning, all observations are included in one cluster. Then, the cluster is split according to the largest distance between the data points. Once a sufficient number of clusters is reached, the process is stopped.

Figure 2.5Illustration of K-means clustering.
Density-Based Clustering: In this type of clustering, the algorithm tries to find the areas with high and low density of observations. Data points that are within a specified distance become centers of a cluster. Other data points either belong to a cluster border or considered as noise.
2.4.3.3 Semi-Supervised Learning
This learning approach combines both supervised and unsupervised ML techniques. Thus, the machine learns from both labeled and unlabeled data. This approach is more realistic for many applications, wherein small amount of labeled data is available, but the collection of large set of labeled data is not easy or impractical.
2.4.3.4 Reinforcement Learning
Similar to unsupervised learning in the sense that the machine has to learn by itself. However, a reward mechanism is applied to tune the algorithm based on observation of performance, enabling continuous self-update of the machine. Reinforcement learning algorithms try to define a model of the environment by determining the dynamics of the environment. The algorithm uses an agent which interacts with a dynamic environment in a trial-and-error manner. It provides feedback to the algorithm. The agent makes decisions on what actions to be performed to optimize the reward. A policy determines how the agent should behave at a given time. Thus, the algorithm learns by exploring the environment and exploiting the knowledge. The feedback from the environment is used to learn the best policy to optimize the cumulative reward.
The most commonly known reinforcement algorithm is the Q-Learning. The RL algorithm interacts with the environment to learn Q values. The Q value is initialized. The machine observes the current state, chooses an action from a set of possible actions, and performs the action. The algorithm observes the reward and the new state. The Q-value is updated based on the new state and the reward. Then, the state is set to the new state and the process repeats until a terminal state is reached.
2.4.4 ML and Wireless Networks
Читать дальше