DEFINITION OF MACHINE LEARNING
Machine learning unlocks the hidden insight of data by allowing machines to learn from examples and experiences. Instead of writing the code explicitly, what you do is feed the data to the generic algorithms in the machines. The machines then analyze this data, change the data patterns, and build the logic to serve predictions on previously unseen data.
Evolution of Machine Learning
Ever imagine what business was like 50 years ago when there were no computing machines? We can thank those genius philosophers, mathematicians, and computer scientists who made what was once fiction a reality. Today, the technology that helps us do everything from housecleaning to driving cars is no longer science fiction. Machine learning helps all businesses and individuals to improve decision making, detect diseases, increase productivity, navigate vehicles and suggest the fastest route after analyzing traffic patterns, forecast weather, detect fraud, and much more.
Figure 1.2shows the journey of how technology came together and how machine learning evolved.
The constant evolution of ML from robotic process automation to technical expertise has made its mark in many sectors. All businesses, ranging from start‐ups to global multinationals, want to develop a robust ML strategy in an increasingly ambitious and technical market.
Currently, businesses are working to achieve the following: advancement in cybersecurity, regulation of digital data, and faster computing power. In the following chapters, this book will teach you to utilize advanced ML solutions in Dynamics 365 for executing complex tasks and sustaining accuracy for the success of businesses.
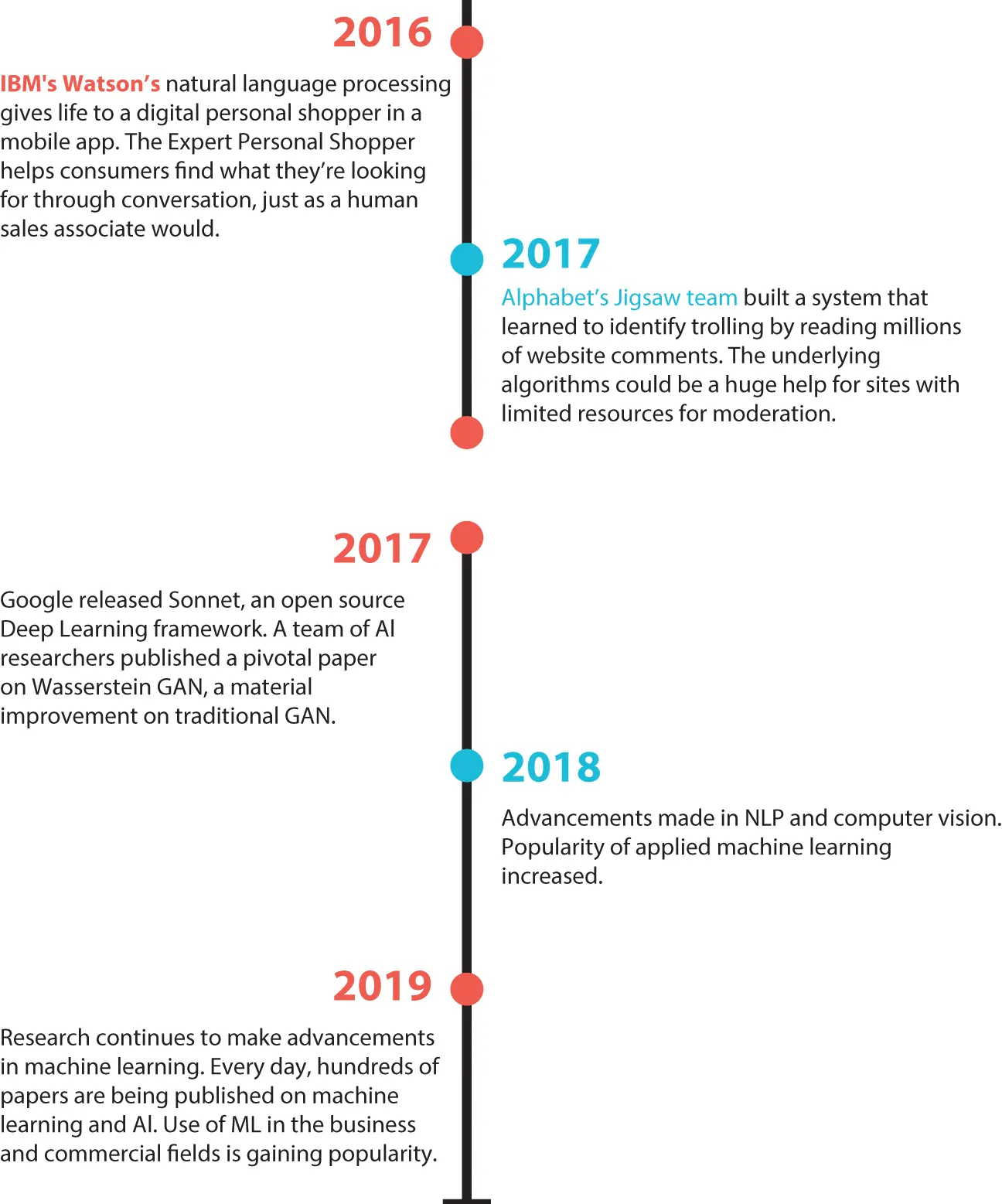
FIGURE 1.2 Evolution of machine learning.
Lifecycle of Machine Learning
Data‐driven organizations face different challenges in developing ML models, from prototyping to production. To derive practical business values, data scientists and data engineers serve the model with a huge amount of data and train it to take advantage of ML algorithms. To create a desirable ML system, businesses need to comprehend the ML lifecycle process. Now let's understand why ML lifecycle is so important for businesses.
According to sas.com, 50 percent of models never make it to production due to the following reasons:
Insufficient data. Insufficient data, when fed to the model, result in an increase in variance. Variance is a value that is equal to the difference between the prediction accuracy of training data and test data in the ML model. If the prediction accuracy between training data and test data is high, the model will produce accurate results with training data but will stop working as soon as test data is fed into it.
Nonrepresentative training data. It is the training set of data that doesn't reflect the cases of the deployment environment. This problem is also called sampling bias. It is necessary to make sure that the sample you are feeding to the model matches the environment it's going to be deployed in.
Poor quality data. It refers to the data that has missing observations, errors, outliers (values that deviate from other observations on data), and noise (spurious and unnecessary data).
Overfitting the data. It is a situation when the model learns the detail and noise in the training data so well that it produces negative results when fed with new data.
Underfitting the data. This situation occurs when you want to build an accurate model with fewer data. Due to a lack of data, the model is unable to capture the underlying trend of the data.
So, to build a model, it is crucial to have the right data, at the right time, in the right location. The ML lifecycles play a key role in building custom ML algorithms to support learning models. The main purpose of the lifecycle is to create a model with a good workflow that can be reproduced, revisited, and deployed to production easily.
Now let's understand what the machine learning lifecycle is and how it works:
The machine learning lifecycle is a repetitive process to build an efficient machine learning system called a “model.”
The machine learning lifecycle consists of four phases: (1) data preparation, (2) machine learning model, (3) validation, and (4) deployment. This lifecycle is all about gaining deeper insights from data. It is leveraged by data engineers, data scientists, and those working with data to develop, train, validate, and serve machine learning models. Figure 1.3depicts a typical ML lifecycle and its phases.
Let's jump into these phases one by one.
Machine learning algorithms need the right data to solve the problem. So first, you need to make sure that the data you have collected is on a useful scale and format. Data preparation is a process that involves converting raw data into a clean dataset before applying machine learning algorithms to the data.
FIGURE 1.3 Machine learning lifecycle.
“The goal is to turn data into information and information into insight.”
— Carly Fiorina
Data preparation involves four phases (also see Figure 1.4):
1 Data collection or data selection. The performance of the model solely depends on how effectively it can achieve accuracy, precision, classification, anomaly detection, and recommendation. This step involves gathering the subset of all available data from multiple resources within your organization. It's not always a good idea to include all the data that is available. The key is to select quality data. People always think that more is better. But while selecting data, you need to strongly address the problem you are working on. For example, you need your model to predict the travel time information of a vehicle for a given expressway by predicting the average speed of the expressway in the future. FIGURE 1.4 Data preparation process.This problem definition does not provide any specific inputs that should be taken into consideration, so it is important to create a list of feasible inputs and output variables. To estimate the average speed of the expressway in the future, you need to map past data in the model. Past data should include the theoretical knowledge of the transportation system, user behavior, user statistics, traffic on business days and on weekends, traffic at daytime and nighttime, and so on.To select quality data, make some assumptions and be sure of the solution you want from your model. However, the process of collecting data is actually a really important step in the ML lifecycle. And all the different decisions that you have to make while collecting data can end up having a pretty big effect on your model results.
2 Data cleansing. To cleanse your data, you first need to understand your data. To gain valuable insights, you need quality data, but the raw data consist of incorrect or missing values. So relevant data must be sourced and cleansed. There are two key stages of selecting relevant data from raw data:Data assessment. It is a task to evaluate the feasibility of available data and how it aligns to your business problem. To understand the data, you need to label your examples in an appropriate category so that the machine learning model should learn to predict. There should be sufficient data to build a machine learning model because the usage of data depends on the algorithms you are using and the complexity of the problem.Data exploration. This is the phase to test your assumption and to create meaningful summaries of your data. In this phase, you search for missing values, outliers, and unbalanced data. Data cleansing is a process that deals with issues occurring in raw data such as missing values, outliers, unbalanced data, typos in data, duplicate data, and so on. Let's see how data is cleaned in this stage:Missing values. If your data has missing values, then you should explore the reason behind it to decide whether or not it is possible to drop these data points as a whole. If dropping data points is not an option, then impute the substitute values in place of missing data.Outliers. Outliers are the values that differ from other observations in the data. To deal with outliers, you need to impute alternate values to make it useful for your machine learning model. You can use algorithms such as random forest or gradient boosted trees.Unbalanced data. Unbalanced data refers to the lack of a similar number of examples. For instance, to detect fraud, you need to have the same number of fraud cases as normal cases, as we know that machine learning learns from examples. So, if your data lack in fraud cases, it will be difficult for a ML model to identify it. So, it is necessary to have balanced data.
Читать дальше