We determine the coordinates of a person’s wrists using a state-of-the-art pose estimation framework, OpenPose [10]. OpenPose is the first real-time 2D multi-person human pose estimation framework that achieves the tasks of jointly detecting the human body, hand, face, and foot-related key points from a single image. The OpenPose framework identifies a total of 135 feature points in the detected human. This is accomplished using a multi-stage Convolutional Neural Network (CNN) that uses a nonparametric representation called Part Affinity Fields (PAFs) to learn how to associate the body parts with the corresponding humans in the image. The OpenPose multi-stage CNN architecture has three crucial steps:
1 1. The first set of stages predicts the PAFs from the input feature map.
2 2. The second set of stages utilizes the PAFs from the previous layers to refine the prediction of confidence maps detection.
3 3. The final set of detected PAFs and Confidence Maps are passed into a greedy algorithm, which approximates the global solution, by displaying the various key points in the given input image.
The architecture of the CNN used in OpenPose consists of a convolution step that utilizes two consecutive 3×3 convolutional kernels. The convolution is performed in order to reduce the number of computations. Additionally, the output of each of the aforementioned convolutional kernels is concatenated, producing the basic convolution step in the multistage CNN. Before passing the input image (in RGB color space) to the first stage of the network, the image is passed through the first 10 layers of the VGG-19 network to generate a set of feature maps. These feature maps are then passed through the multi-stage CNN pipeline to generate Part Confidence Maps and PAF. A confidence map is a 2D representation of the belief that a given body part can be located in a given pixel of the input image. PAF is a set of 2D vector fields that encodes the orientation and the location of body parts in a given image.
We use the OpenPose framework’s “BODY_25” pose model to extract the spatial coordinates of both wrist landmarks, PL ( x , y ) and PR ( x , y ), of a person denoted by keypoints 4 and 7, respectively, as shown in Figure 3.3.
Figure 3.3 Keypoints for pose output [10].
3.3.3.3 Calculation of Confidence Score
We determine the confidence score ( C ) which indicates the extent to which the given active garment is a garment of interest for a given customer. In order to accomplish this, we first calculate the Area of the active garment ( AAG ), whose top-left and bottom-right spatial coordinates are denoted as AGTL ( x , y ) and AGBR ( x , y ), respectively, using Equation (3.1).
(3.1) 
Then, we determine the minimum Euclidean distance ( D ) between the centroid of the active garment AGC ( x , y )) and the coordinates of the two wrists of a person using Equations (3.2)and (3.3).
(3.2) 
(3.3) 
Finally, we calculate the confidence score ( C ) for determining the extent to which the given active garment is a garment of interest using Equation (3.4).
(3.4) 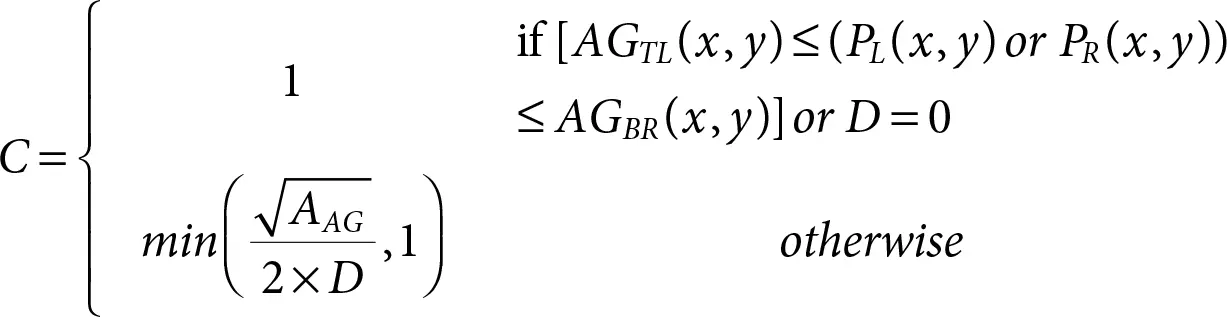
The confidence score ( C ) is calculated for each pair-wise distinct set of detected person’s wrists and active garment where the highest score is retained for that active garment . If this confidence score exceeds a specified confidence threshold (δ), then the active garment in consideration is considered to be a garment of interest to the given customer as his/her wrist landmarks are in close proximity with the garment. The proposed approach also notes the time duration for which such interaction takes place by keeping track of the number of frames for which the active garment is a garment of interest for the given customer.
In this section, we discuss the results of our proposed approach on the garment dataset. The experiments were conducted on a device with IntelⓇ Core i7-8750H 2.20 GHz CPU, NVIDIA RTX 2070 with 8 GB VRAM, and 16 GB DDR4 RAM. Additionally, some programs were computed on Google Colab [21] with IntelⓇ XeonⓇ 2.00 GHz CPU, NVIDIA Tesla T4 GPU, 16 GB GDDR6 VRAM, and 13 GB RAM. The programs were written in Python 3.6 and utilized OpenCV 4.2.0 and OpenPose 1.6.0.
The dataset used to evaluate the proposed approach is a collection of video surveillance footage of an Indian garment store. The videos capture the interaction between the salesperson and the customers while selecting a garment for purchase. The videos are obtained from a CCTV camera in the store’s infrastructure, thus enabling us to work on real-life surveillance videos that have practical illumination conditions and background noise. The raw videos in the dataset have a resolution of 944 × 576 pixels. These videos were pre-processed to have a resolution of 1,920 × 1,080 pixels and a consistent frame rate of 30 FPS before computation. There a total of 33 videos in the dataset with an average duration of 1 minute. Among these videos, 22 videos contain a single customer, 8 videos contain two customers, and 3 videos contain three or more customers. Thus, the chosen dataset represents practical conditions and is adequately diverse. Figure 3.4illustrates a few samples from the dataset.
3.4.2 Experimental Results and Statistics
The proposed approach was evaluated by measuring the effectiveness of the detection of active garments and the identification of garments of interest .
Figure 3.4 Samples from the dataset.
Table 3.2 Precision and recall of active garment detection.
Precision (%) |
Recall (%) |
87.36 |
85.92 |
The effectiveness of active garment detection was evaluated by measuring its precision and recall , which are shown in Table 3.2. These metrics have been calculated as follows:
(3.5) 
(3.6) 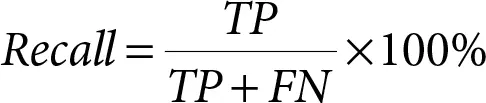
Читать дальше