Predicting Heart Failure
Здесь есть возможность читать онлайн «Predicting Heart Failure» — ознакомительный отрывок электронной книги совершенно бесплатно, а после прочтения отрывка купить полную версию. В некоторых случаях можно слушать аудио, скачать через торрент в формате fb2 и присутствует краткое содержание. Жанр: unrecognised, на английском языке. Описание произведения, (предисловие) а так же отзывы посетителей доступны на портале библиотеки ЛибКат.
- Название:Predicting Heart Failure
- Автор:
- Жанр:
- Год:неизвестен
- ISBN:нет данных
- Рейтинг книги:3 / 5. Голосов: 1
-
Избранное:Добавить в избранное
- Отзывы:
-
Ваша оценка:
- 60
- 1
- 2
- 3
- 4
- 5
Predicting Heart Failure: краткое содержание, описание и аннотация
Предлагаем к чтению аннотацию, описание, краткое содержание или предисловие (зависит от того, что написал сам автор книги «Predicting Heart Failure»). Если вы не нашли необходимую информацию о книге — напишите в комментариях, мы постараемся отыскать её.
Predicting Heart Failure: Invasive, Non-Invasive, Machine Learning and Artificial Intelligence Based Methods
Predicting Heart Failure
Predicting Heart Failure: Invasive, Non-Invasive, Machine Learning and Artificial Intelligence Based Methods
Predicting Heart Failure — читать онлайн ознакомительный отрывок
Ниже представлен текст книги, разбитый по страницам. Система сохранения места последней прочитанной страницы, позволяет с удобством читать онлайн бесплатно книгу «Predicting Heart Failure», без необходимости каждый раз заново искать на чём Вы остановились. Поставьте закладку, и сможете в любой момент перейти на страницу, на которой закончили чтение.
Интервал:
Закладка:
With the use of artificial intelligence in health and especially in heart diseases, it has been possible to estimate the risk, diagnoses, and work flows and to apply sensitive treatment methods [21]. Thanks to artificial intelligence techniques, it has been possible to interpret large-sized data sets and thus obtain a more precise diagnosis. Within the artificial intelligence discipline, machine learning, image processing, robotics, and even natural language processing artificial intelligence techniques will be able to participate in the early detection and diagnosis of HF as well as outcome prediction and prognosis evaluation [22].
In a proposed study to help clinicians diagnose HF at an early stage, a scoring model based on a support vector machine (SVM) has been proposed. According to this model, samples were divided into three groups as healthy group, HF prone group, and HF group. The overall accuracy of the model in classification was 74.4% [23].
A study by Guidi et al. [24] was designed to help non-field experts make decisions in the analysis of HF. The system is based on three functional parts: diagnosis (severity assessment), prognosis, and follow-up. Four artificial intelligence techniques are used in the diagnostic function: artificial neural network (ANN), SVM, decision tree, and a fuzzy system with genetic algorithm support. A new technique for identifying HF patients using spectral analysis and neural networks was investigated in a study by Elfadil et al. [25]. A data set was used in the study, with 17 of the 53 samples being normal and the rest being patients. HF patients were divided into four groups with an accuracy of 83.65%.
Gharehchopogh et al. [26] presented a decision support model to help physicians in the study. The model, based on an ANN, was able to detect the presence or absence of HF with 85% accuracy. The study is within the scope of medical data mining.
Candelieri et al. developed a decision tree to determine patient stabilization [27] (with 88% accuracy). Pecchia et al. used decision tree techniques to classify patients into three severity groups using heart rate variability (HRV) measurements (HF: Healthy = 96% accuracy; Severe–Moderate = 79.3% accuracy) [28].
1.6 Machine Learning Supported Diagnosis
Invasive and non-invasive methods offer a wealth of diagnostic information. However, the interpretation of the available information can only be possible with the help of a physician. The increase in heart patients and the increase in patient data in parallel make it difficult to evaluate the data and extract information from them day by day. The intersection of the symptoms of heart disease with the symptoms of other diseases also makes the diagnosis of the disease a difficult problem. For this reason, there is a need to evaluate the data obtained with the help of invasive and non-invasive techniques with intelligent analysis tools in order to increase the diagnostic accuracy. Artificial intelligence and machine learning will assist physicians in intelligent analysis. Machine learning models trained with past patient data can be used to diagnose future cases. With the diagnostic capabilities to be gained by the machines, it will sometimes be possible for them to diagnose more precisely and more sensitively than the physicians. Supporting decision support systems working in expert systems logic with machine learning models will enable them to give better results.
1.6.1 Introduction to Machine Learning
Disease prediction and diagnosis can be made with the help of machine learning models. Disease diagnosis applications have been developed and used extensively, especially with controlled machine learning techniques. This technique has enabled models to be created from historical data and these models have sometimes been used in diagnosis and treatment. Developing a system based on machine learning is not just about developing machine learning algorithms, but rather it is done by working on data step by step from start to finish in a way similar to the data mining process. For example, determining which variables are important and which are not important in the solution of a problem directly affects the quality of the solution. This process, called feature selection, determines which parameters will be used in the system to be installed. The feature selection process is often achieved by establishing the correct relationships between targeted data and predictive data.
The feature selection phase is followed by feature transformation. Data transformation, which is a method that improves data quality, has recently emerged as feature engineering, which includes feature studies performed to increase prediction success. Like feature selection, feature engineering will also affect the success of the result. Both feature selection and feature engineering also solve the problem of high dimensionality in data. Loss of data and methods of combating loss are also important. The fight against lost values is sometimes carried out by estimating the lost value and sometimes by replacing it with other values.
It is also important to choose the learning models to be used when creating machine learning systems. There are four basic learning methods under the title of machine learning: supervised learning, unsupervised learning, semi-supervised learning, and reinforced learning. The latter two methods, semi-supervised learning is a type of learning that occurs with the combination of supervised and unsupervised techniques; reinforced learning is an agent-based learning technique where the decision is made according to the rewarding mechanism, and it is used to reveal the most possible solution to a subject that we did not have any previous knowledge about.
The most prominent techniques – supervised and unsupervised learning – will be explained in the following paragraphs.
1.6.2 Machine Learning Algorithms
There are many machine learning algorithms developed to date. To understand algorithms, the two learning methods of supervised learning and unsupervised learning (see Figure 1.2) need to be understood.
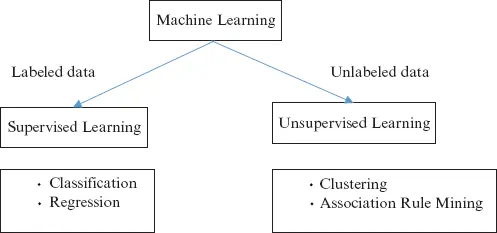
Figure 1.2 Supervised and unsupervised machine learning.
Supervised learning algorithms are often used in machine learning and perform two basic tasks: classification and regression. The output of classification operations is a nominal value, while the output of regression operations is usually a continuous value. Although supervised learning algorithms are often used to diagnose diseases, the field of machine learning is, as we have seen, wider and includes the additional methods we have mentioned, which are used for jobs where supervised learning is insufficient.
1.6.2.1 Supervised Learning
Supervised learning consists of two basic steps: creating a model with labeled data and testing with untagged data, the two prominent techniques in the category of supervised learning algorithms. Classification, one of the two prominent techniques in the category of supervised learning algorithms, is a supervised learning technique in which the target variable is of categorical type, while regression, the other prominent technique, is of the numerical type of the target variable. Operations are performed based on a model in which the target variable calculated from predictive variables is estimated. The purpose of classification is to assign records seen for the first time to one of the predefined categories. Identification and modeling of categories takes place with the help of training data. Training data and machine learning algorithms come together to form machine learning models. Machine learning models also match the records to the classroom that suits them best.The most important feature that distinguishes supervised learning from unsupervised learning is label information in supervised data. It is the class label in the data that provides the control. Although the output of both methods is different, the goal is to estimate the value of the output variable based on input variables.
Читать дальшеИнтервал:
Закладка:
Похожие книги на «Predicting Heart Failure»
Представляем Вашему вниманию похожие книги на «Predicting Heart Failure» списком для выбора. Мы отобрали схожую по названию и смыслу литературу в надежде предоставить читателям больше вариантов отыскать новые, интересные, ещё непрочитанные произведения.
Обсуждение, отзывы о книге «Predicting Heart Failure» и просто собственные мнения читателей. Оставьте ваши комментарии, напишите, что Вы думаете о произведении, его смысле или главных героях. Укажите что конкретно понравилось, а что нет, и почему Вы так считаете.