Handbook on Intelligent Healthcare Analytics
Здесь есть возможность читать онлайн «Handbook on Intelligent Healthcare Analytics» — ознакомительный отрывок электронной книги совершенно бесплатно, а после прочтения отрывка купить полную версию. В некоторых случаях можно слушать аудио, скачать через торрент в формате fb2 и присутствует краткое содержание. Жанр: unrecognised, на английском языке. Описание произведения, (предисловие) а так же отзывы посетителей доступны на портале библиотеки ЛибКат.
- Название:Handbook on Intelligent Healthcare Analytics
- Автор:
- Жанр:
- Год:неизвестен
- ISBN:нет данных
- Рейтинг книги:4 / 5. Голосов: 1
-
Избранное:Добавить в избранное
- Отзывы:
-
Ваша оценка:
- 80
- 1
- 2
- 3
- 4
- 5
Handbook on Intelligent Healthcare Analytics: краткое содержание, описание и аннотация
Предлагаем к чтению аннотацию, описание, краткое содержание или предисловие (зависит от того, что написал сам автор книги «Handbook on Intelligent Healthcare Analytics»). Если вы не нашли необходимую информацию о книге — напишите в комментариях, мы постараемся отыскать её.
The book explores the various recent tools and techniques used for deriving knowledge from healthcare data analytics for researchers and practitioners. A Handbook on Intelligent Healthcare Analytics
Handbook on Intelligent Healthcare Analytics — читать онлайн ознакомительный отрывок
Ниже представлен текст книги, разбитый по страницам. Система сохранения места последней прочитанной страницы, позволяет с удобством читать онлайн бесплатно книгу «Handbook on Intelligent Healthcare Analytics», без необходимости каждый раз заново искать на чём Вы остановились. Поставьте закладку, и сможете в любой момент перейти на страницу, на которой закончили чтение.
Интервал:
Закладка:
Machine data : Machine data refers to the data generated by machines, such as wearable, sensor devices, web logs, and satellites.
Transactional data : The transactional data are generated as a result of the transactions. The transactions can be online or offline. Examples of the transactional data are the delivery receipts, order, invoices, etc.
Human generated data : The human generated data is extracted from the emails, electronic medical reports, messages, etc.
Search engine data : The search engine data are generated from the browsers.
All the abovementioned data are in diverse formats such as comments, videos, email, and sensor data, most of which are in unstructured format. Big data is the huge size of a data set that grows exponentially with time. Examples of big data: Amazon product list, YouTube videos, Google search engine, and Jet engine data. Storing and processing of abovementioned big data is not possible with conventional databases because traditional databases can contain only gigabytes of data. But, the big data contains several petabytes of data. The big data solutions solve this entire problem with distributed storage and processing systems.
3.2.2 Big Data: Characteristics
The massive set of information generated with the utilization of the latest technologies is called big data. This large set of data is used for individual and organizational purposes. Previously, the information was generated, stored, and processed easily because of limited sources of data. The conventional database was the single supply of data. Most of the data in the conventional database was in structured format. Presently, data is in a wide variety of formats such as sensor data, email messages, images, audio, and video, which makes the big data. Most of this data is unstructured. The five common big data characteristics are volume, variety, veracity, velocity, and value. Apart from the abovementioned five dimensions of big data, many researchers also added new dimensions of big data. Other dimensions of big data are volatility, validity, visualization, and variability. Big data is characterized by following commonly adopted V’s. Figure 3.1and Table 3.1represent the big data features in term of Vs [24].
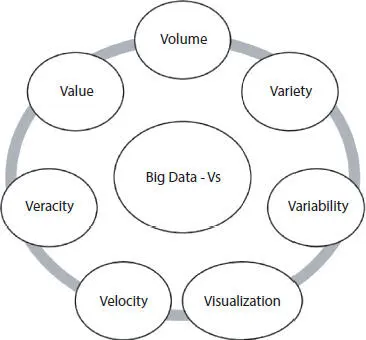
Figure 3.1 Dimensions of big data.
Table 3.1 Dimensions of big data.
Dimensions of big data | Description |
Volume | In general, volume refers to quantity or amount. The data that contains gigantic larger data sets is called volume in big data. Big data is known for its voluminous size. The data is being produced on a daily basis from various places. |
Variety | Meaning of variety is diversity. The data that are generated and collected from diversified sources is called a variety in big data. Usually, big data is in a variety of forms that comprises structured, semi-structured, and unstructured data. |
Velocity | Velocity means speed. In big data context, the velocity denotes the speed of data creation. Big data is arriving at a faster rate, like a stream of water. |
Veracity | The veracity is the credibility, reliability, and accuracy in big data and the quality of the data sources. |
Variability | The variability is not the same as variety. The variability means data that are regularly changing. This is an important feature of big data. |
Visualization | Big data is illustrated in the pictorial form using the data visualization tools. |
Value | The potential derived from the big data is known as value. |
3.3 Big Data Tools and Techniques
3.3.1 Big Data Value Chain
The value chain of big data is the process of creating potential value from the data. The following steps are the value chain process for creating knowledge insights from big data [12, 25, 29].
• Production of big data from various sources
• Collection of big data from various sources
• Transformation of raw big data into information for storing and processing
• Preprocessing of big data
• Storage of big data
• Data analytics
Production of big data from various sources
The production and generation of big data at various sources refers to big data generation. This is the initial step in the value chain process. Big data is known for its volume. The data is generated from various sources on a regular basis. Similarly, medical data is generated by the clinical notes, IoT, social media data, etc. Big data includes internal data of the industry and also includes internet and IoT data.
Big Data Collection
The second phase of the value chain process is the collection of data from a fusion of different places. Big data is generated at various sources. All these are in various formats like text, image, and email, which may be structured, semi-structured, and unstructured data. Big data collection process used to retrieve raw data from the various sources. The most common big data sources are computers, smartphones, and the Internet [14].
Big Data Transmission
Once the data collection is over, it should be transformed to the preprocessing infrastructure.
Big Data Pre-Processing
Big data is collected from a variety of sources. So, the data may consist of inconsistent, noisy, and redundant data. The data preprocessing phase improves the data quality by removing inconsistent, noisy, incomplete, and redundant data. This process improves the data integrity for data analysis.
Big Data Storage
The data should be stored for exploration. Big data refers to huge data that cannot be processed and stored using traditional information systems. The large amount of big data can be stored and handled easily with the help of big data storage technologies. All these technologies ensure data security and integrity.
Big Data Analysis
The final phase in the value chain process is analysis. Artificial intelligence, deep learning, machine learning algorithms, and other data analytical tools are used to analyze the data and to extract knowledge from a huge data set. Based on the data analysis process, the decision-makers can solve the problem and make good decisions.
3.3.2 Big Data Tools and Techniques
Technologies are used to analyze, process, and extract the useful insights available in complex raw data sets. The major technologies of big data are storage and analytics technologies. The most accepted big data tools are Apache Hadoop, Oracle NoSQL, etc. The technologies of big data are classified into operational and analytical.
Operational big data: This data is generated on a daily basis. Ticket booking, social media, online shopping, etc., are examples of operational big data.
Analytical big data: This data is nothing but analyzing the operational big data for real-time decisions. Examples of analytical big data are the medical field and weather forecast.
The technologies and tools used in big data are classified on basis of following steps [11]:
• Gathering of big data
• Processing the big data
• Storing the big data
• Analyzing the big data
• Visualizing the big data
Читать дальшеИнтервал:
Закладка:
Похожие книги на «Handbook on Intelligent Healthcare Analytics»
Представляем Вашему вниманию похожие книги на «Handbook on Intelligent Healthcare Analytics» списком для выбора. Мы отобрали схожую по названию и смыслу литературу в надежде предоставить читателям больше вариантов отыскать новые, интересные, ещё непрочитанные произведения.
Обсуждение, отзывы о книге «Handbook on Intelligent Healthcare Analytics» и просто собственные мнения читателей. Оставьте ваши комментарии, напишите, что Вы думаете о произведении, его смысле или главных героях. Укажите что конкретно понравилось, а что нет, и почему Вы так считаете.